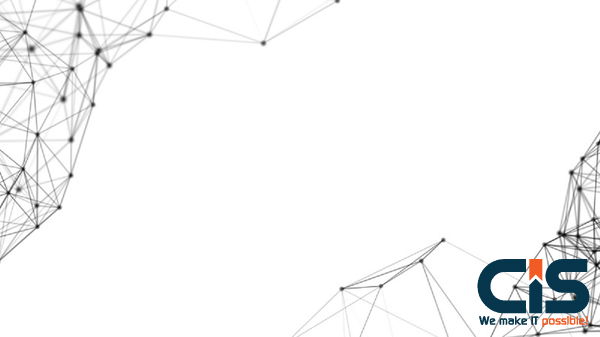
Any entrepreneur can sign-up for BigQuery or Snowflake today and get a data solution that scales with their business within hours. The massive increase in data volume prompted the development of flexible and affordable data storage solutions that can scale with changing business needs.
Currently, the world produces 2.5 quintillion data bytes per day. The explosion of data continues in the roaring '20s, both in terms of generation and storage -- the amount of stored data is expected to continue to double at least every four years. One component of the modern data infrastructure is still lacking solutions that can meet the Big Data era's challenges. This is monitoring and validation of data quality.
Let me tell you how we got to this point and what the future holds for data quality.
Big Data's dilemma between volume and value
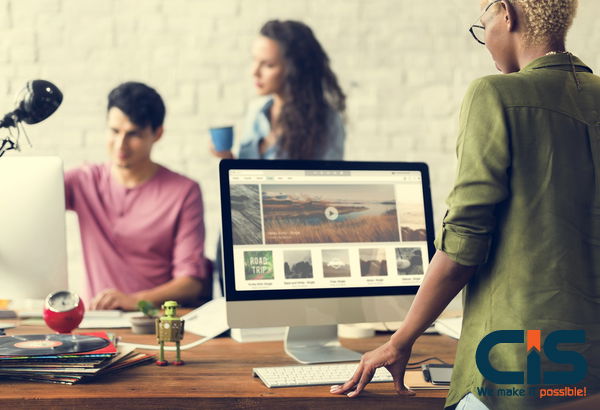
Tim O'Reilly's groundbreaking 2005 article, "What is Web 2.0?" set off the Big Data race. Roger Mougalas, O'Reilly, introduced the term "Big Data", in its modern context. This refers to a large amount of data that is almost impossible to process or manage using traditional BI tools.
In 2005, managing large amounts of data was a major challenge. Data infrastructure tools were expensive and difficult to use, and the market for cloud services was just beginning (AWS was not launched publicly until 2006). The other was speed: As Tristan Handy from Fishtown Analytics (the company behind debt) notes, before Redshift launched in 2012, performing relatively straightforward analyses could be incredibly time-consuming even with medium-sized data sets. These two issues have been addressed by an entire data tooling ecosystem.
It used to be difficult to scale relational databases or data warehouse appliances. A company that wanted to understand customer behavior had to purchase and rack servers to allow its data scientists and engineers to generate insights. This was only 10 years ago. Large-scale data storage and ingestion were prohibitively expensive.
We must ensure that large amounts of Big Data are sufficiently high quality before they can be used.
Then, there was a (Redshift). AWS introduced Redshift in October 2012. It was cloud-native and massively parallel processing (MPP), a database that anyone could use at a monthly cost of $100. This is 1,000x less than the "local-server" setup. A price drop this large opened the floodgates to allow every company, no matter how big or small, to store and process huge amounts of data and unlock new possibilities.
As Jamin Ball from Altimeter Capital summarizes, Redshift was a big deal because it was the first cloud-native OLAP warehouse and reduced the cost of owning an OLAP database by orders of magnitude. Also, the speed at which analytic queries were processed increased significantly. Snowflake was the first to do this. Later, they separated storage and computing, which allowed customers to scale their computing and storage resources independently.
What was this all about? A surge in data storage and collection.