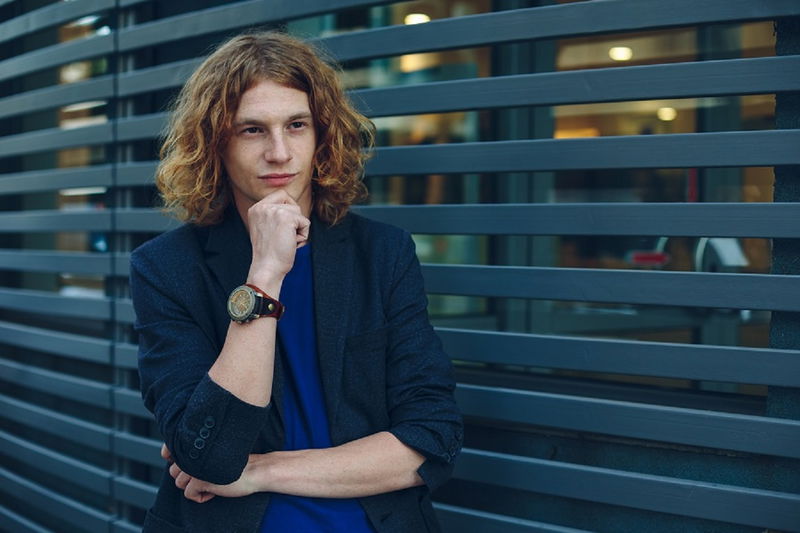
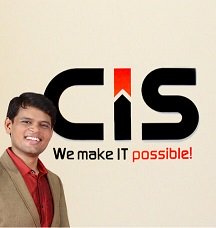
Contact us anytime to know more β Amit A., Founder & COO CISIN
As part of Artificial Intelligence, machine learning involves making computers learn from data by discovering patterns to predict outcomes without explicitly programming computers.
Statistics are utilized for trend identification and prediction using experience. At the same time, machine learning algorithms allow computers to analyze complex forms of data quickly to detect emerging patterns and build models that adapt as the data changes over time.
Machine learning, an artificial intelligence (AI) area, allows systems to learn and evolve without explicit programming.
In this blog post, we'll look at how machine learning has transformed how businesses operate - particularly how Intelligent Process Automation with Machine Learning automates the capture, processing and submission of documents such as invoices or purchase orders to streamline your operations today!
What is Machine Learning?
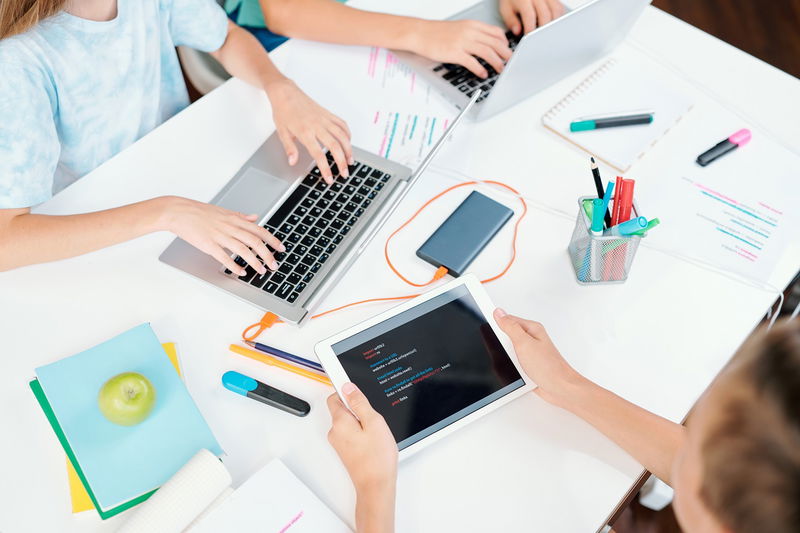
Machine learning, an emerging computer science discipline, allows computers to automatically learn from experience and make improvements without being explicitly programmed.
Machine learning works by analyzing large datasets for emerging patterns; these patterns are utilized as predictive models in later machine-learning steps.
Machine learning offers businesses an innovative solution for improving performance and operations.
AI and Machine Learning: What is the Difference?
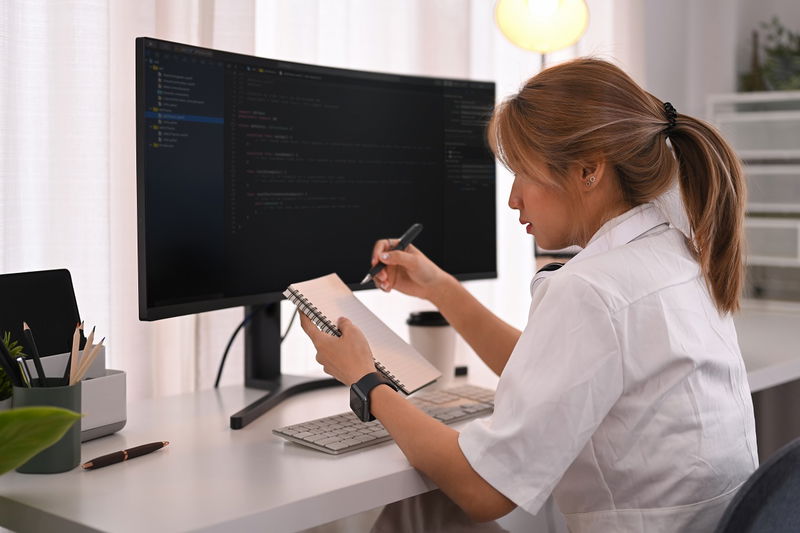
Artificial Intelligence and machine learning may seem related but they are different disciplines. AI involves building intelligent machines capable of performing human intelligence-like functions such as problem-solving, reasoning, decision-making and language processing.
Machine Learning relates closely to AI but differs significantly.
As part of artificial Intelligence (AI), machine learning involves teaching computers how to continually enhance their performance on specific tasks by learning from data.
AI encompasses many techniques, from rule-based and genetic algorithms to decision trees. AI comes in supervised and unsupervised forms; both types may be utilized for applications including autonomous cars, chatbots, image recognition etc.
AI refers to all techniques and methodologies for developing intelligent machines, while machine learning is one specific AI technique.
Examples of Machine Learning in Business Software
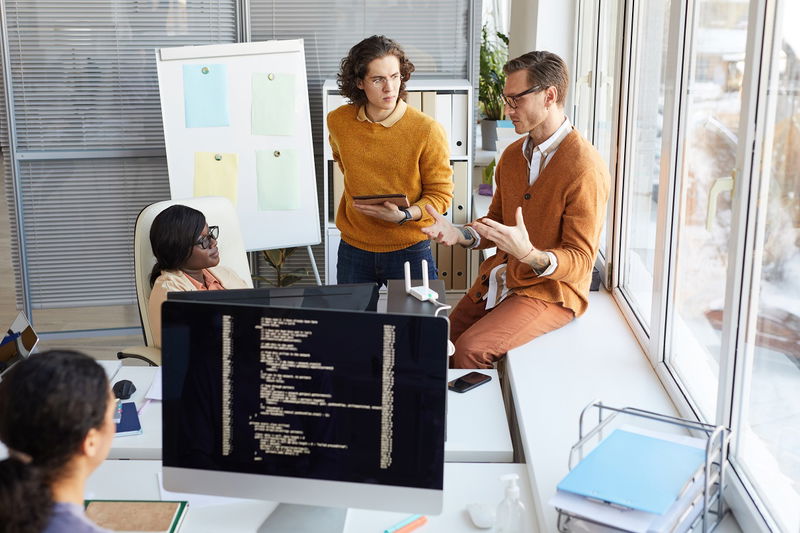
Artificial Intelligence has profoundly transformed our world with products such as ChatGPT and Notion AI. Document automation offers another great opportunity for AI use; machine learning reduces human error, while Arty's Auto-Find uses machine-learning techniques to recognize similarly structured documents for faster document processing.
Auto-Find is designed to extract information from documents for approval or correction by an operator. At the same time, Advanced Auto-Find is more comprehensive, categorizing fields by type while employing regular expressions to detect irregularities within data structures and providing active AI training capabilities.
These tools were created to alleviate operators of an oppressive burden and enable more efficient data collection.
Machine learning and AI will save valuable time for you so you can concentrate on tasks you excel in doing your best.
Data Science and Machine Learning: Not the Same?
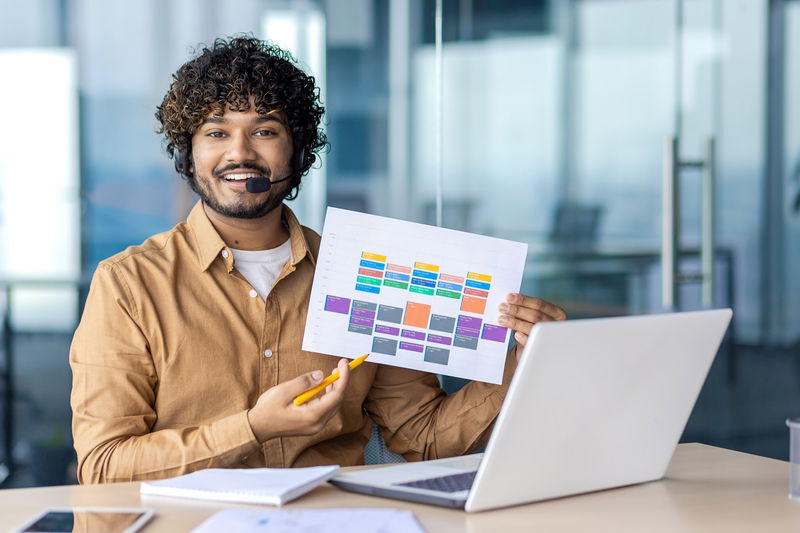
Machine learning and data science are both related but different concepts.
- Data science is multidisciplinary and uses algorithms, systems, scientific processes and methods to gain insights from unstructured and structured data. Data science is a multidisciplinary field that uses various tools and techniques from different fields, such as computer science, statistics and domain knowledge, to solve complex problems and make sense of data.
- On the other hand, machine learning is a branch of AI that involves teaching machines how to improve their performance at specific tasks over time by analyzing data. Machine learning uses models and statistical algorithms to help machines recognize patterns, classify information, and predict the future.
Data science can be defined as an umbrella term covering various techniques used for extracting insights from data.
The process includes tasks like cleaning the data, analyzing it and creating predictive models - with machine learning as one tool within data cleaning itself. Machine learning may even train machines in data science to recognize certain patterns within data.
What are The Types of Machine Learning?
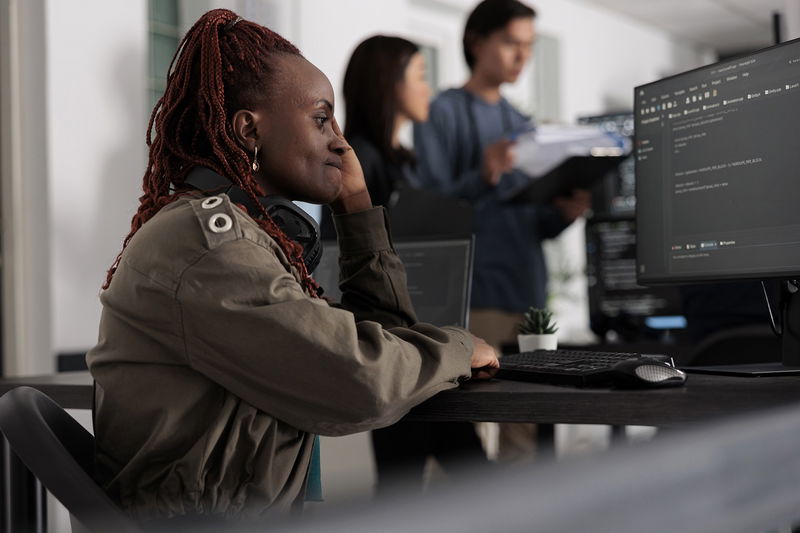
Machine learning is divided into three types, each suitable for a specific task or application. Let's discover.
Learners Are Supervised During Their Learning
The first type of machine learning involves training the algorithm using already labeled data with the desired output.
It is then measured how accurate the algorithm's predictions are against the test data.
Image classification, speech detection, and spam filtering are examples of supervised learning.
Unsupervised Learning
Unsupervised machine learning is a type of unsupervised training where the algorithm uses unlabeled input data. This means that there are no outputs.
The algorithm learns how to recognize patterns in data without any guidance. The output of the algorithm will be a clustering or grouping of data.
Unsupervised learning is a useful tool for anomaly detection, market segmentation, and recommendation systems.
Reinforcement Learning
Machine learning involves an algorithm that makes decisions based on environmental feedback received. This machine learning type aims to maximize reward functions through actions that produce positive outcomes while minimizing any possible negative ones.
Reinforcement learning has great potential in robotics and the control of autonomous vehicles.
Other types of machine learning include semi-supervised analysis, where data are labeled or unlabeled, and deep machine-learning algorithms, which utilize artificial neural networks to extract insights from analyzed datasets.
What is Machine Learning Algorithms (MLA)?

Computer programs called machine-learning algorithms allow machines to self-improve by learning from experience without being explicitly programmed.
Machines can automatically improve their performance using these algorithms; data collected is processed into predictions or decisions based on this learning. To introduce some types of machine-learning algorithms:
Learners Are Supervised During Their Learning
A machine-learning algorithm of this type involves training a model using labeled data. Labeled data is simply data that has already been labeled correctly.
This algorithm uses the learned data to predict new data that is not labeled.
Unsupervised Learning
A machine-learning algorithm of this type involves training a model using unlabeled datasets. This means the algorithm must identify the patterns and relationships within the data.
This algorithm type is used to perform tasks like clustering and anomaly detection.
Reinforcement Learning
A machine-learning algorithm of this type involves training the model by trial and error. This algorithm is designed to learn through both positive and negative feedback to maximize a reward.
Machine learning algorithms (ML) are important in many technologies today. They are also becoming increasingly popular as companies seek ways to use data for competitive advantages.
We will discuss machine learning algorithms later. They are applied in many applications, including fraud detection, image recognition, natural-language processing, predictive maintenance, and fraud detection.
Also Read: What Is Machine Learning? Different Fields Of Application For ML
What's The Difference Between Deep Learning And Machine Learning?
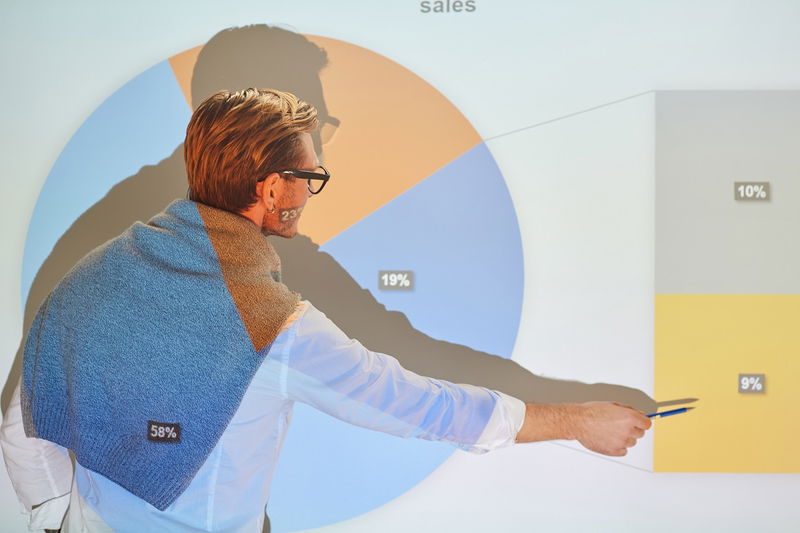
A subset of Machine Learning, Deep Learning involves several neural network layers. Both machine learning and deeper learning involve using algorithms to train on data to predict or make decisions.
However, they differ significantly.
- Complexity: Deep Learning models have many layers and interconnected nodes. They can be used to learn abstract features from input data.
- Features Engineering: Traditional machine learning models require feature engineering by hand, which includes selecting and transforming input data to enhance the model's performance. On the other hand, deep learning models can learn to recognize features automatically from raw data. This saves time while improving accuracy.
- Requirements for Data: Large datasets are required to train deep learning models effectively. Traditional machine learning models can perform better with smaller datasets.
- Performance: Deep Learning Models are more accurate on difficult tasks, such as natural language processing or image recognition, than machine learning models. However, they may need more computing resources for training and running.
Deep learning, in essence, is an advanced form of machine learning used to achieve greater accuracy when performing complex tasks.
While its implementation still requires additional computational and data resources for optimal training.
What is NLP Machine Learning (NLP)?
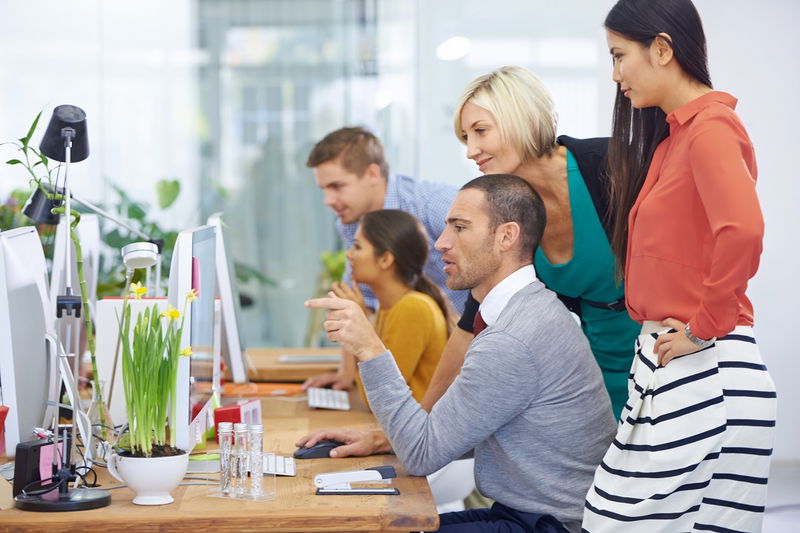
Natural Language Processing Machine Learning (NLP-ML) is an artificial intelligence subfield that seeks to equip computers to interpret and create human languages.
NLP Machine Learning involves creating algorithms and models to process natural language data such as speech, images or texts into information useful for translation tasks, sentiment analysis, classification or information extraction.
NLP Machine Learning Models are typically trained using large amounts of text from articles, books, social media posts and other sources such as text from social media posts or articles published within them.
Models learn to recognize patterns within that data before applying that knowledge to generate or predict new language structures or expressions.
NLP Machine Learning finds applications across industries. Examples of its uses are customer service chatbots and voice assistants for customer interactions and sentiment analysis of social media postings for monitoring purposes.
What Is The Role Of Machine Learning In Business?
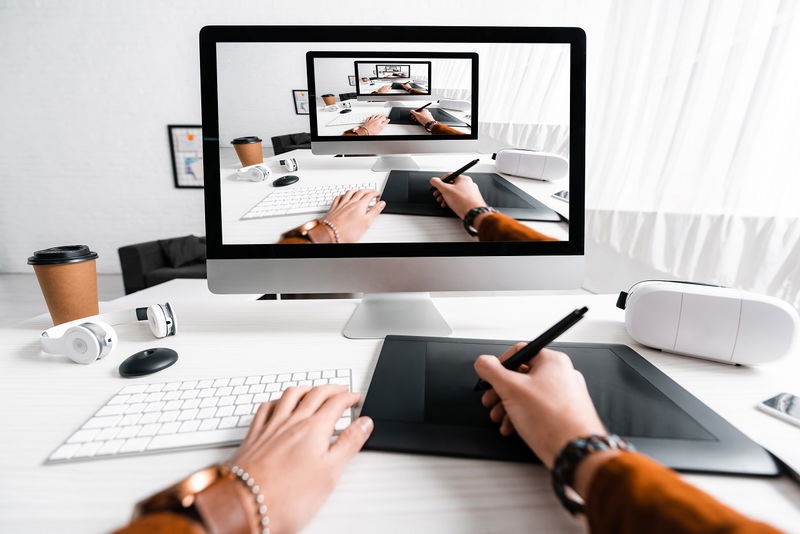
Machine learning has quickly become an indispensable resource for businesses of all kinds - from marketing and customer service, risk assessment and forecasting, all the way through to customer relationship management (CRM) systems.
Amazon utilizes machine learning to optimize prices, predict demand and recommend products. At the same time, Google employs it for personalized emails and search suggestions based on previous search data.
Machine Learning Benefits for Business
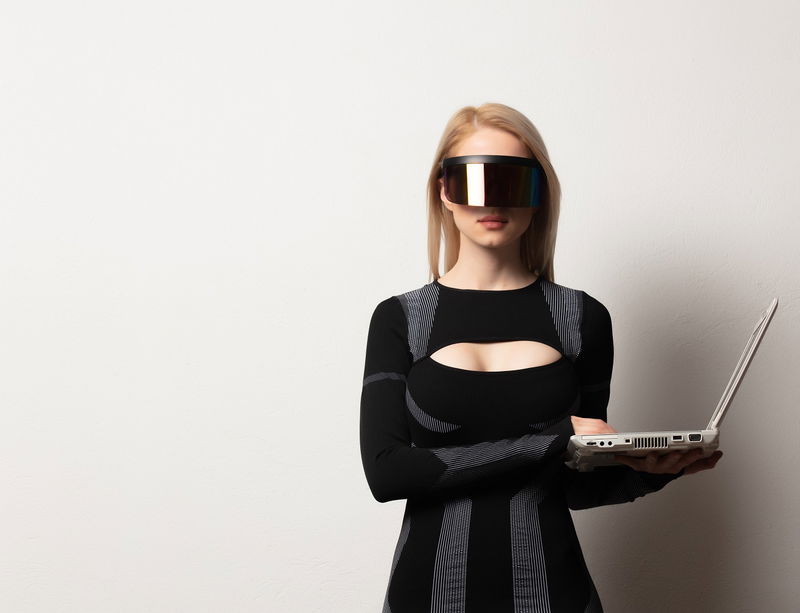
It is impossible to overstate the benefits of machine learning for business. Machine learning can improve business efficiency and reduce costs.
It also enhances customer service.
Machine learning has many benefits for businesses. Some of these are listed below.
- Productivity and Efficiency: Machine Learning algorithms help automate time-consuming and repetitive tasks. This allows employees to concentrate on more valuable tasks. It can improve efficiency and productivity in various business functions.
- Cost Savings: Increased efficiency is a great way to save money. Machine learning can help businesses reduce costs by automating processes and identifying patterns within data. This is especially useful in marketing and operations.
- Customer Experience: Machine Learning can personalize customer interactions and deliver a superior customer experience. Machine learning algorithms, for example, can be used by a company to suggest products and services based on a client's previous purchases or browsing history.
- Accuracy & Quality: Machine Learning can improve accuracy & quality in such areas as data entry and analysis. It can reduce mistakes and boost overall performance.
- Predictive Analysis: Machine Learning algorithms are used to forecast future trends, which can provide businesses with insights and help them make better decisions.
- Fraud Detection: Machine-learning algorithms detect data patterns that indicate fraud, allowing businesses to prevent and detect fraudulent activity.
- Optimization of Supply Chain: Machine Learning can optimize supply chains by optimizing inventories, predicting demand and identifying improvement areas.
You can see that machine learning can be a great asset to businesses. It can improve operations, lower costs and give them a competitive edge.
Look below at some of the common applications for machine learning in business.
Customer Service Machine Learning

By improving customer service efficiency, machine learning can provide a personalized experience to customers. Machine learning can be used to improve customer service in several ways.
Chatbots
Chatbots can be trained to respond instantly to customers' inquiries using machine learning algorithms. Chatbots can handle large volumes of inquiries simultaneously, allowing businesses to offer 24/7 customer service.
The chatbot can be programmed to respond with personalized answers based on the customer's past interactions.
Analyze of Sentiments
Algorithms based on machine learning can be used to analyze customer feedback and determine the sentiment. It can help companies better understand their customer's needs and preferences and enhance their product or service accordingly.
Personalized Recommendations
Machine-learning algorithms can recommend services and products to customers based on their previous purchases or browsing histories.
It can improve customer satisfaction and loyalty.
Predictive Analysis
Algorithms based on machine learning can identify customers at high risk for churning, allowing businesses to respond to their concerns in a proactive manner.
The machine learning algorithms can predict what products and services the customers are likely to purchase.
Voice Recognition
Using machine learning algorithms to understand and recognize customer voice commands can improve accessibility and allow hands-free interactions.
Machine learning improves customer service by providing quicker and more personal responses. Businesses can use machine learning to understand customers' needs better and provide better service.
Machine Learning in Business Operations
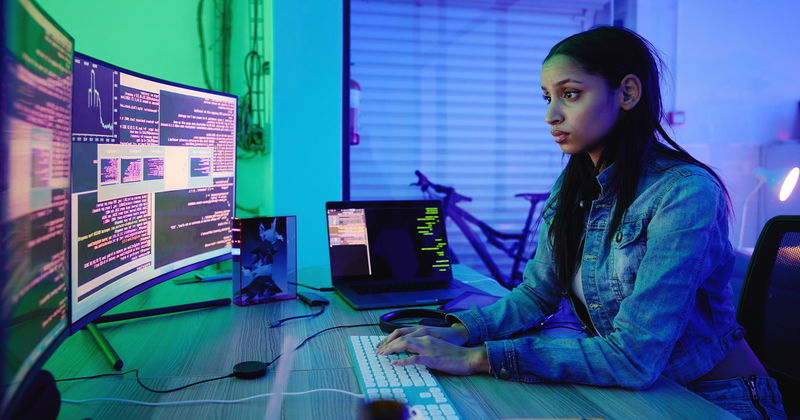
In operations, machine learning can also reduce costs and improve efficiency. Machine learning can be used to improve efficiency and reduce costs.
Fraud Detection
Algorithms based on machine learning can identify data patterns indicative of fraudulent behavior. Businesses can use this to detect and prevent fraud on credit card transactions and insurance claims.
Supply Chain Management
Machine learning algorithms can improve supply chain operations by predicting customer demand, optimizing stock levels and identifying improvement areas.
It can reduce business costs while improving customer satisfaction.
Predictive Maintenance
Using machine learning algorithms allows businesses to plan maintenance before equipment breaks down. It can reduce maintenance and downtime costs and increase the efficiency of a business.
Businesses can increase their customer satisfaction by integrating machine learning into operations.
This will improve efficiency and reduce costs. By providing insights and forecasts that are difficult to get through conventional methods, machine learning helps businesses make better business decisions.
Machine Learning in the Business Process Automation

In many business processes, machine learning simplifies repetitive tasks that consume much time. Using machine learning, you can automate your daily, weekly, and monthly tasks without human involvement.
Customers Churn: Predicting Customer Churn
To avoid losing customers due to churning, identify and target the ones who are most at risk. The machine learning algorithms use historical customer data, demographics, and previous market behavior to determine the probability of customers leaving in the future.
It helps businesses deliver customized offers to keep customers happy and prevent churn.
Improve Sales Productivity
Machine learning allows us to gain knowledge from multiple data sets instead of a single one. This allows us to discover hidden patterns in the data, which would otherwise be impossible for humans to detect by simply reviewing every experience separately.
It is particularly useful when making sales predictions. Machine learning and predictive data analytics can help companies personalize marketing, target the best prospects and increase ROI.
Personalized Marketing
Digital product personalization is considered one of today's business's most important growth opportunities. Companies can tailor marketing campaigns by leveraging the customer data stored in CRM databases.
Companies can use machine learning algorithms to identify customer behavior patterns and create different predictive models that will give them an edge over their competitors.
As the algorithm gains more knowledge, these predictions become even more accurate.
Forecasting Demand
Today, many businesses have difficulty predicting future demand. This often leads to stock-outs that result in significant revenue losses.
Retailers must forecast accurate demand using product sales from the previous year to maintain adequate inventories to prevent stock-outs. Suppose a business sells a million units in one quarter, and the item is popular with customers. In that case, it must order 2 million pieces of inventory to prevent stockouts.
Machine learning algorithms can consider various factors, such as past sales, demographics and weather conditions, to predict demand accurately.
Identify Fraud Patterns
Machine learning is a key tool in fraud detection. Machine learning algorithms identify anomalies that may indicate fraudulent activity, like credit card fraud and money laundering.
Machine learning algorithms can, for example, be used in credit card fraud to create a predictive model that will predict whether a particular transaction is fraudulent by analyzing past data on how customers use their cards. Money laundering is a case where algorithms identify irregularities in large transactions. This can be used as a way of identifying possible criminal activities.
Risk Management
Machine learning is a powerful tool for making decisions. Machine learning algorithms can identify possible business risks by analyzing data that is difficult to understand by humans, like millions of scientific reports and papers, technical details about products, claims for insurance, and weather forecasts.
Predictive Maintenance
Machine learning is a powerful tool for predicting equipment failures and machine breakdowns. Machine learning algorithms help businesses understand the way equipment is used and the environment in which it operates.
They can then analyze data to identify what could trigger equipment failure. These algorithms will help to plan maintenance ahead of time and avoid equipment failures. This can increase productivity through a reduction in downtime.
Machine learning algorithms help businesses make sense of their data, improve the customer experience, and create new opportunities for business.
As they are easy to implement and require little human involvement, machine learning systems allow organizations with limited IT expertise and resources to do so. The systems constantly learn from the data they receive and generate insights that can be applied in different business cases.
Machine Learning Projects: Challenges for Business
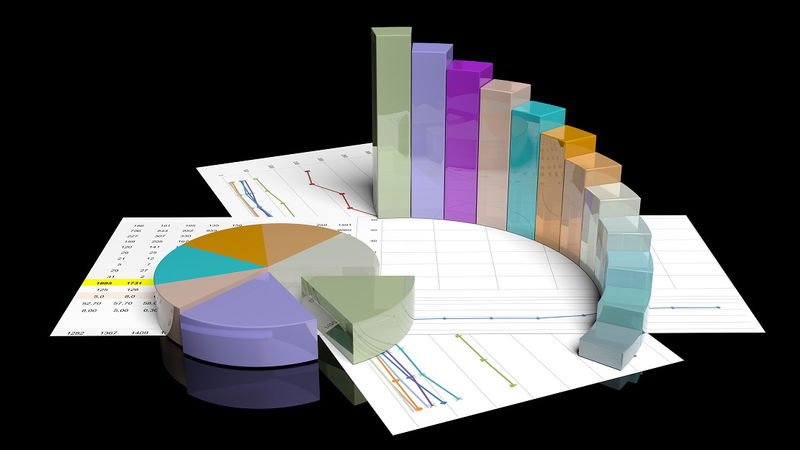
Before companies can adopt machine learning, there are various hurdles they must navigate first. One such hurdle is data privacy issues - to avoid legal fines, businesses must ensure their data collection complies with industry regulations.
Data quality and volume pose further obstacles for machine learning projects, with machine learning algorithms needing vast quantities of information to learn from data.
Businesses require assistance collecting, organizing and storing their datasets. At the same time, machine learning projects may use inaccurate or incomplete information, compromising algorithm accuracy.
Businesses using machine learning must ensure the security of the data used within it. To safeguard sensitive information against theft or unintended access, businesses should implement safeguards, including encryption, audits, and controls on access.
Machine learning projects necessitate data science, programming, statistics and data analysis expertise from data professionals; unfortunately, many businesses find it challenging to retain skilled individuals capable of developing or maintaining these projects, leading them to delay or abandon them completely.
Machine learning algorithms can be complex to comprehend and integrate into existing systems and processes, leading companies needing assistance scaling their machine-learning projects as data volumes expand and complexity increases.
As companies struggle to keep pace with demand for these projects.
Cost and return on investment present another challenge to machine learning projects. The costs associated with creating and managing machine-learning initiatives can be high as it requires significant investments in hardware, software and human talent to develop them successfully.
Furthermore, businesses must demonstrate a return on investment for machine learning initiatives to secure stakeholder support and justify investments.
Conclusion
Business process automation can be an incredible asset to businesses; when combined with artificial intelligence technologies like artificial neural nets (ANNs), automation becomes even more effective, and your business could achieve new heights.
Artificial Intelligence will now form part of over 40% of new enterprise software implemented by service providers, meaning machines will now be responsible for its creation! Machine learning's potential applications for businesses are endless - faster predictions can be made using it. In contrast, better decisions are made as machine learning provides businesses with a competitive edge over time.