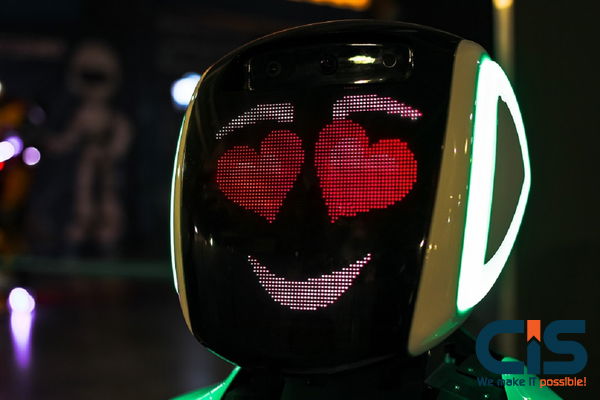
While many companies consider LiDAR to be an integral part of the collection of sensors for self-driving vehicles, Tesla asserts that LiDAR is not all that important. In fact, LiDAR is not featured in any of Tesla's vehicles. Tesla vehicles make use of other features like maps, GPS, radar, other sensors, etc. In addition to Tesla, it seems that there are other people too who are of the same opinion. Researchers at the Cornell University, in their recent discovery, have inferred that the usage of LiDAR isn't too necessary. The researchers used two cameras, which were inexpensive, one on each side of the windshield of a vehicle. The researchers found that detection of objects was possible this way, with an accuracy that was almost as good as that of LiDAR and at a cost that was significantly less than that of LiDAR.
Additionally, researchers also discovered that the accuracy had more than tripled when the images that were captured were analyzed through a bird's eye view, thus demonstrating that stereo camera was a more economical alternative to LiDAR.
So, what does this all mean for self-driving cars? It means that building such cars without LiDAR could be possible. In LiDAR, the three-dimensional maps of the surroundings are created via lasers. LiDAR relies on the light's speed to calculate the distance of the objects. On the other hand, stereo cameras determine depth using 2 perspectives. While critics may say that stereo cameras offer low accuracy in detecting objects, the researchers at Cornell University have stated that data captured using stereo cameras were on par with LiDAR in terms of preciseness. It was during the analysis of the data of the stereo cameras when a gap was observed in the accuracy.
With camera images, taking the frontal view into consideration is quite appealing. However, this could be problematic because if the objects are viewed from the front, then the manner in which these objects are processed deforms the objects, the shapes of the objects are deformed and the objects are blurred into the background.
In self-driving cars, the analysis of the data which is captured through sensors or cameras is done with the help of convolutional neural networks. According to the researchers at Cornell University, while convolutional neural networks are excellent when the task is object identification for photographs in standard colors, any three-dimensional data can be distorted by these networks, if the data representation is a frontal representation. Here again, the accuracy had more than tripled when the frontal representation was changed into a bird's eye view.
In the current scenario, we assume that machine learning algorithms will be able to extract the necessary information from the data we provide to the algorithms, regardless of the data representation. But the results of the research indicate that it may not actually be so and that data representation is an area that we need to do more thinking in.
Because of the fantastic range accuracy provided by LiDAR, the industry of self-driving cars has been quite keen on using LiDAR despite it being expensive. But the bird's eye view of the data of the cameras, that greatly improves range accuracy and detection, could be the revolution that changes things in the industry.