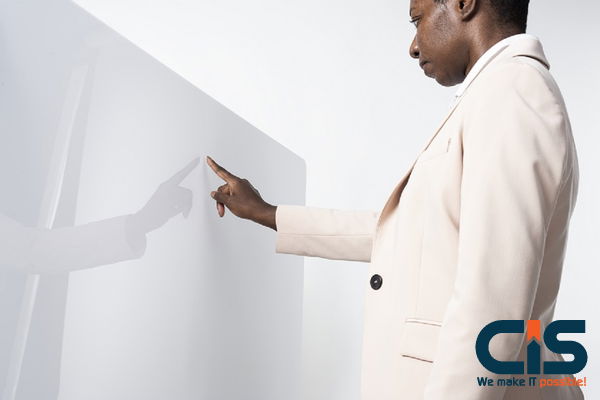
AI technology has quickly become widely adopted within society, and this use has contributed to its increasing popularity. Applications range from self-driving cars like Tesla to facial recognition and fraud detection applications; their benefits are tangible, but they remain challenging in practice. All these benefits do not equate to artificial Intelligence being without risk; however, challenges exist nonetheless.
What Will Be the Biggest Challenges for Artificial Intelligence in 2023?
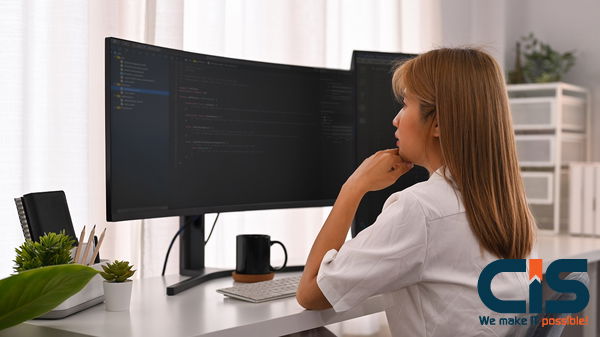
By 2023, artificial intelligence (AI), which has gained significant attention recently, will have achieved major advances that drive innovation across various industries. Even with all this progress made towards AI integration, however, integration will present its own set of difficulties requiring strategic thought to overcome any stumbling blocks along the path toward full adoption of this revolutionary technology. As part of our examination of AI challenges in this section, we will address various complexities that impede the widespread adoption and integration of AI systems. By understanding more deeply these obstacles organizations face when harnessing their true potential, we may gain greater insights.
Lack of Understanding
AI is still a relatively young field, and we still have much to discover regarding its inner workings, leaving many who need to be made aware of what makes an accurate and reliable AI system possible. Companies can invest in research efforts designed to increase understanding of various language models, algorithms, and techniques. At the same time, collaboration initiatives and knowledge-sharing platforms facilitate information flow between members of the AI community.
Privacy Concerns
AI systems depend heavily on data to operate efficiently and can contain sensitive and personal information, which raises privacy concerns. Companies should implement stringent privacy measures in order to address such worries, such as anonymizing and secure storage; additionally, they must adhere to any relevant data protection regulations as well as have transparent policies in place regarding data usage in order to build trust among their target population and reduce privacy worries.
Processing Power Requirements
AI systems are computationally intensive and demand considerable processing power in order to complete complex tasks efficiently, leading to high infrastructure costs for AI apps that demand many resources. Hardware innovations, like dedicated AI chips or distributed computing systems, can assist companies in meeting artificial intelligence challenges more easily. In contrast, google cloud computing offers flexible yet affordable access solutions to computational resources.
Data Shortage
AI systems rely heavily on vast datasets and diverse data sets for training purposes and optimal performance. Yet, not all industries possess enough volume or quality data for AI to thrive. Artificial Intelligence solutions to address such data shortages include collaborations and partnerships that provide access to pertinent datasets; techniques like data augmentation, transfer learning, and synthetic data are also effective ways of helping address limited availability issues.
Unreliable results
AI systems may produce unreliable results due to various factors, including incomplete or biased datasets, algorithmic limitations, or task complexity. To address these problems with artificial intelligence systems, businesses should focus on conducting rigorous tests and validating processes when developing AI solutions; monitoring and refining are also crucial in order to provide consistent, accurate outcomes from artificial intelligence solutions.
Lack of Trust
Skepticism of AI systems often stems from a need for more understanding of their functioning. Therefore, transparency and explanation of AI algorithms and decision-making processes are crucial in building trust between AI users, stakeholders, and companies. By providing easily accessible explanations on how their conclusions were reached by their AI systems, companies can build more confidence. Likewise, encouraging ethical AI practices by complying with standards and regulations also fosters trust and helps create lasting results.
Uncertain Goals
Companies often need to work on setting clear AI objectives within their organization and creating effective AI systems without clearly defined goals and KPIs that align with business strategies. Companies should conduct an in-depth evaluation of business processes where AI may add value. Companies must then assess where AI could add the most benefit before setting objectives aligned with KPIs that facilitate implementation initiatives related to artificial intelligence (AI).
Technical Difficulties
AI systems must face numerous technical obstacles, including data storage and security concerns. Investment in infrastructure capable of handling AI-related data volumes is necessary in order to build user trust; similarly, data protection must also be addressed throughout their lifecycle and expanded as needs or expansion arise. Scalability needs should also be kept in mind from its inception so as not to limit future expansion opportunities of these AI solutions.
Bias in Algorithms
AI algorithms may inherit biases from their source data and produce unfair or discriminatory results, creating unfair or discriminatory outcomes. This challenge becomes especially pertinent as AI systems continue to play an increasingly significant role in decision processes across diverse domains.Companies should create strategies to encourage inclusiveness and fairness to combat algorithmic biases. These initiatives involve selecting and preprocessing training data carefully to reduce any bias patterns, developing techniques for bias detection and mitigation, performing regular audits to verify fairness within AI systems, and performing regular checks of AI processes for fairness purposes.
Implementation Strategy
AI implementation cannot be approached using one-size-fits-all strategies; every company's needs differ; therefore, an appropriate implementation plan needs to be tailored specifically for it. Assessing current infrastructure, data availability, and organizational readiness is vitally important before undertaking such initiatives.An AI implementation roadmap must be drawn up that clearly details resources, timelines, and steps necessary for its successful deployment. Seeking outside advice can provide invaluable assistance during this stage. Collaborating and consulting AI experts could prove extremely helpful during implementation processes.
AI 4 Challenges for Mastery Heading into 2023
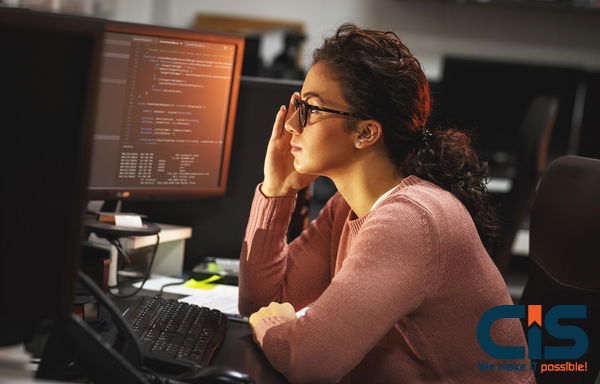
Accenture will present "The Art of Artificial Intelligence" on June 8th, 2022, report on maturation stage usage by companies; only 12 percent used AI. At the maturity stage, usage increased significantly, with 63% using it and attaining superior growth and transformation results. Only 13 surveyed used AI initially, while only 12 percent attained maturity stage usage; that leaves us just scratching the surface!
While AI can present many challenges that impede its adoption into your enterprise AI strategy, four primary obstacles will emerge between now and 2023 that should help companies develop an AI road map and strategy - strategies that separate mastering AI from playing around with it for fun or profit.
AI for Business: Creating a Culture of AI Culture
Companies that excel in using AI to solve real-world business issues will foster an environment that promotes innovation at all levels of the organization, from top management down. A. I am now used by hundreds of thousands of companies around the globe; data scientists and engineers within these firms are responsible for creating machine-learning models that benefit the firm in question. Yet, these teams often need more support or supervision from decision-makers or C-Suite executives. Companies looking to start AI data teams must consult with top executives in order to understand any obstacles facing the business and then use machine-learning models as solutions to address those challenges.
Data scientists who work in organizations without an established AI infrastructure often need help selling projects to executives if the model relies solely on black box results for learning purposes, without them understanding its workings and how its results were obtained.By providing education to all employees - CEOs, IT, marketing/sales staff, and office staff alike - including CEOs, IT, marketing/sales staff, and office staff, you can overcome language and technological barriers and build support and understanding within an organization. Data scientists need help to work alone - rather, they require collaboration across departments.
From Experimental AI Projects into Production
Many organizations need help with implementing artificial intelligence. Experimental projects often turn into production work. Most business challenges can be traced directly back to the absence of an AI culture; therefore, this poses a problem.CEOs frequently need help grasping artificial intelligence (AI), what its capabilities and limitations are, or whether or not AI is even relevant in today's economic environment and impending crisis. AI may help the bottom line via machine learning models or solutions that reduce customer churn or marketing mix modeling. At the same time, other prediction problems may not be possible due to various reasons. Management must also understand how machine-learning models function and the time required to build one. Building machine learning takes time; only 63% of 745 companies surveyed in Algorithmia's "2020 State of Enterprise ML Report" had successfully deployed machine-learning models into production by average, taking longer than one month per company on average to get it out there and live.
Data scientists must work through vast quantities of raw data. They must select which pieces to utilize, consult experts for input, train models, and benchmark results against benchmarking tools. When working against deadlines - like forecasting the impact of a recession in three months - this task becomes even more arduous when taken up alongside developing AI models, which take time. Trying out experimental projects may prove especially challenging to complete successfully.Technically speaking, many experimental projects fail to reach production because they only use part of larger data. An experimental model may perform successfully on its initial subset when deployed and operationalized for live data. However, it could fail or drift significantly. Python code used by data science specialists often needs help with operationalizing models as this language provides only high-level, general-purpose programming language capabilities, which makes changing machine learning code so that production systems can use it time-consuming.
Investing In Talent, Technology and Data Environments
As AI services gained more acceptance, new businesses emerged to serve them. Machine Learning Automation platforms became widely adopted due to their ability to streamline data scientist tasks quickly. New AI solutions bring four distinct advantages. Solutions worth prioritizing include discovering patterns, building data pipes, validating features, and business insight. Data engineers and scientists can use new technologies to find patterns quickly across large enterprise datasets into flat tables for machine learning; data pipelines allow feature stores to be constantly updated with the most up-to-date ML features for faster development times; business insights may also come through from these efforts. Data scientists can expedite development time by validating machine learning features to ascertain their "evaluability" to a particular model. This leads to possible new solutions and potentially new business insights from machine learning's ability to crunch large amounts of data.
Artificial intelligence presents businesses with several challenges, not the least being transparency. Companies could develop complex models for forecasting sales or managing inventory without fully comprehending its inner workings, and this poses serious trust issues when trusting its results or recommendations based on them alone.Automatic feature detection poses another difficulty for companies: some assume AI automation platforms can replace their skilled team and investments in machine learning technologies. On the contrary, these platforms were specifically created for data scientists so as to increase productivity while alleviating development frustrations - doing in weeks what normally took months!
Transparency and Responsible AI
Companies such as Google often promote slogans like AI Microsoft refers to its cloud providers as being for "global good." In contrast, Google refers to it as not doing any harm. However, these appear as just simple slogans but, in reality, hold greater weight than meets the eye.Leading Artificial intelligence companies carefully consider their data when planning how they'll utilize machine learning models. A machine model could cause harm by negatively impacting an organization or failing to meet performance benchmarks. Credit scoring companies that use machine-learning models to assess client credit quickly must choose which data sets to include when creating these assessments, with data scientists considering ethical considerations when using gender, race, or zip code information in creating credit scores.
AI and machine learning models combine both aspects, lacking concepts like responsibility, ethics, or morality in their design; rather, they may discriminate or bias results. Machine learning models do not come without ethical obligations. However - transparency plays a pivotal role when using them ethically: everyone in your company needs to understand its logic as well as any data used for reaching conclusions from such models. Transparency becomes an issue when human beings need help to grasp how a machine-learning model solves complex problems without understanding exactly how or what data it uses in making its predictions or solving complex tasks. "Black box" artificial intelligence models pose numerous difficulties that must be solved through development platforms for artificial intelligence applications that offer transparency and traceability of features; users who did not develop it must understand why its conclusions came to fruition, while traceability is defined as developers being able to "trace back" data elements used for specific predictions or features within models created within these platforms for development purposes.
Machine learning should be open, accessible, accountable, and straightforward for its audience to comprehend. Our future will depend upon how we navigate these four intertwined challenges facing both industry and society today. AI driven by business culture means moving from experiments to production; doing this requires investing in talent, technologies, data environments, and responsible AI concepts, as well as creating an inclusive culture around machine learning - what matters is whether organizations master machine learning early enough.
What is Artificial Intelligence?
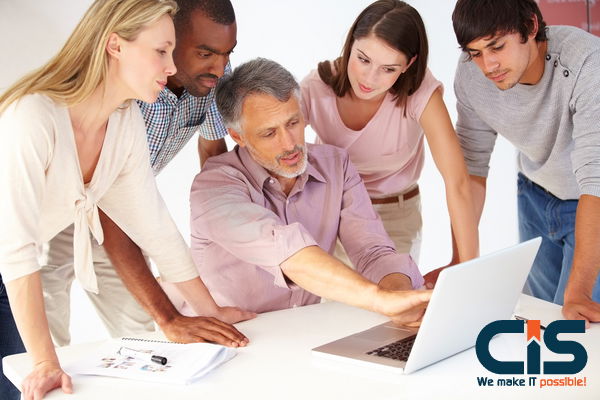
Artificial intelligence can be defined as any computer-controlled robot or digital transformation computer capable of carrying out tasks usually associated with intelligent creatures. generative AI may also include:
- Humans create intelligent entities that perform tasks intelligently without explicit instruction from outside sources.
- Artificial Intelligence can act and think rationally. An average layperson with a limited understanding of technology might associate artificial Intelligence with robots; such an interpretation would liken AI to terminator-type figures that can act on their own.
Artificial intelligence researchers will typically define artificial intelligence as the combination of algorithms that produce results without explicitly being told to. Machine intelligence, or artificial intelligence, is becoming an increasingly hot topic these days, simulating and mimicking human intelligence by machines. AI technologies continue to advance, improving our quality of living as more of these machines learn through a customer experience similar to humans and perform tasks similar to people. Everyone wants some connection with artificial Intelligence, whether as an end-user or as someone pursuing careers related to it.
What is Artificial Intelligence, and What Are Its Types?
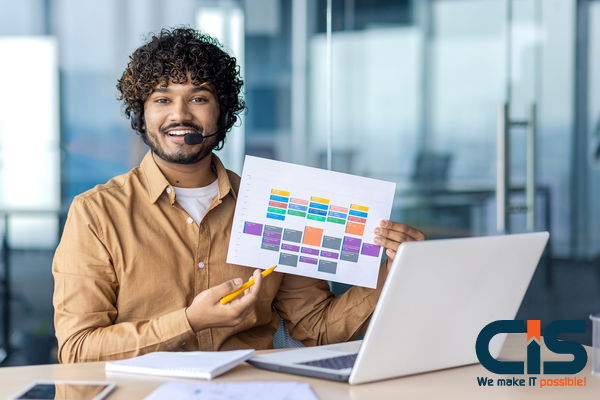
AI only covers some of the above areas simultaneously. Artificial intelligence is built to serve different purposes. AI is classified into Type 1 and Type 2. (Based on functionality). Here is a short introduction to Type 1.
Three Types of Artificial Intelligence
- Artificial narrow intelligence
- Artificial general intelligence
- Artificial super intelligence.
What Does Artificial Narrow Intelligence Mean?
Artificial intelligence systems, more often than not found today on the market, are designed to solve one specific problem and carry it out efficiently and flawlessly. Their scope may include anything from providing product recommendations for an e-commerce user to weather predictions; some AI systems even outshone humans when operating under certain contexts!
What Does Artificial General Intelligence (AGI) Mean?
AGI remains only theoretical. AI with human-level cognitive functions has many domains of application ranging from language processing, image analysis, and computation to reasoning. Unfortunately, we're still far off from building AGI; building such a system would involve thousands of artificial narrow intelligence systems working in concert and communicating in order to replicate human reasoning processes - it took IBM Watson or Fujitsu K forty minutes to simulate one second's worth of neuronal activities! Such complexity underscores our current resource limits when creating AGIs
What is Artificial Superintelligence?
ASI is the next logical step beyond AGI. A system of Artificial superintelligence would surpass human capabilities when it comes to decision-making, rational decisions, and even creating better art or building emotional bonds.
AI systems will quickly advance beyond our wildest expectations, offering capabilities we never could have anticipated. While the gap between AGI & ASI may seem small (some even say as little as a microsecond due to Artificial Intelligence's rapid learning), getting there remains far off - this course teaches how you can start building a career using Artificial Intelligence.
Business Applications of Artificial Intelligence
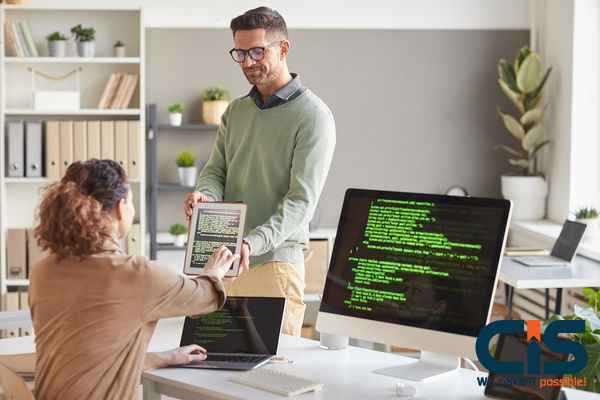
AI can revolutionize many industries. There are endless applications of Artificial Intelligence based upon data processing systems such as those offered by Amazon Web Services; each has the power to enhance business practices across industries. AI's transformative potential lies within its vast capacity for optimization.
Take a look at how artificial intelligence (AI) is currently contributing to various industries.
Healthcare:
- Administration: Artificial intelligence-powered systems assist in routine administrative tasks by eliminating human error and increasing efficiency. Nlp can assist doctors by transcribing medical notes for patient information retrieval.
- Telemedicinein non-emergency situations, patients can reach out to hospital ai systems for analysis of their symptoms by entering vital signs. If medical attention is necessary, medical practitioners are only presented with critical cases, reducing workload significantly.
- AI can now read MRI scans at exponentially higher speed and with a significantly reduced error margin than human radiologists and with a much-reduced margin for error.
- Robot-assisted surgery: Robot-assisted surgery has an extremely small margin for error and can perform surgeries 24/7 without becoming fatigued, with less invasiveness and greater accuracy than traditional methods, potentially shortening hospital stays for patients.
- Vital statistics monitoring: Over time, health can change depending on vital statistics monitoring. Wearable devices have become more prevalent, but this data must still be processed to provide actionable insights that could save a life. Vital signs could predict changes before patients even notice this; this could save their lives!
E-Commerce:
- Better Suggestions: AI has already demonstrated its business applications through better suggestions to users on e-commerce websites - and has resulted in significant bottom line gains for them as a result of using artificial intelligence for product recommendations that match user interests, resulting in more sales for them and therefore an increase in bottom lines overall.
- Chatbots have become one of the more ubiquitous technologies thanks to Artificial Intelligence (AI), chatbots sweeping industries and websites across industries alike. Chatbots now serve customers during odd hours or peak periods without being limited by limited human resources - an incredible boon!
- Filtering fake and spam reviews: Due to Amazon's large volume of reviews, human readers are unable to process all the reviews quickly enough in order to detect malicious material. Artificial intelligence technology such as NLP can detect suspicious activity quickly enough and filter these reviews out so as to enhance buyer's experiences and enhance buyer service levels.
- Optimize Search: E-commerce relies heavily on users being able to locate exactly what they are seeking quickly and effortlessly, and Artificial Intelligence optimizes search engine results using thousands of parameters so as to enable this.
- Supply chain: AI can help supply chain managers predict product demand over different periods and adjust stock to match it.
Human Resources:
- Establishing a Work Culture: AI analyzes employee data to place employees into teams according to their skills and assign projects based on those competencies, collect feedback on workplace environments, and even predict when people may leave their current company.Hiring With NLP AI, employers can rapidly scan thousands of CVs to determine which are compatible quickly. It would reduce hiring cycles while eliminating human biases and errors.
- Robots and AI.Even prior to AI becoming widespread, robotics had already advanced significantly. Artificial Intelligence allows robotics innovators to advance faster by creating efficient robots. AI robots find applications across several industries and verticals, especially manufacturing and packaging industries. Here are a few AI robot applications:
- AssemblyAI and advanced vision systems combined will aid real-time course correction for improved safety.As it's being used, the robot can also identify the optimal route for any process being undertaken.
- Customer ServicesAI-enabled robotics are increasingly being deployed as customer service agents within retail and hospitality environments.These robots utilize Natural Language Processing (NLP) to interact intelligently and naturally with customers, speaking their native tongue.Machine learning systems can gain from interactions with humans as their intelligence increases.
- PackagingAI allows for faster, cheaper, and more accurate packaging of goods.Saving robot motions and optimizing them over time makes installation and relocation simpler, making robotic systems much simpler to deploy and move around.
- Open Source RoboticsOpen-source robots equipped with AI capabilities are now being commercially sold.Users are now able to teach robots customized tasks based on an application.
Why is AI technology so useful?
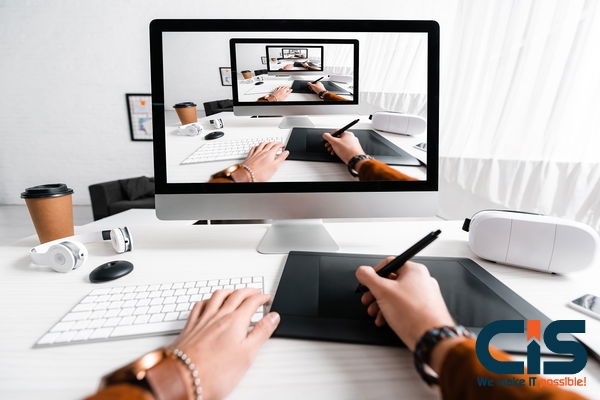
Artificial Intelligence can bring many advantages. Some examples include:Automation AI can easily automate tedious processes/tasks without creating unnecessary fatigue for workers.
Enhancement: AI has the potential to enhance all products and services by improving user experiences and offering better product recommendations.
Accuracy and Analysis: AI analysis can be completed much more rapidly and with greater precision than human analysis can. AI can interpret data for smarter decision-making.AI can assist companies with making smarter decisions and streamlining product and business process improvement efforts.
Career Trends in Artificial Intelligence
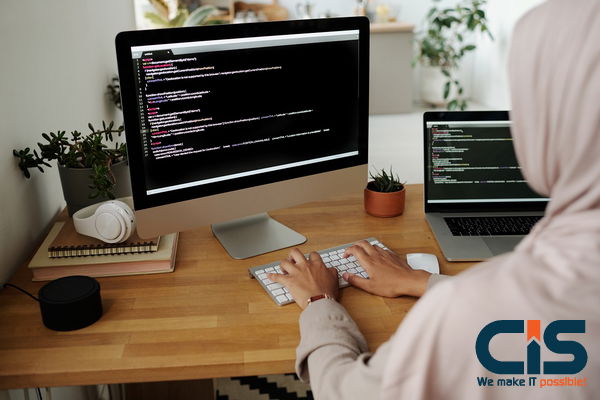
Artificial intelligence careers have grown steadily over recent years and will only increase at an increasing pace. 57% of Indian firms are actively searching for talent who specialize in AI roles to meet market demand; those successfully transitioned have reported average salary increases between 60-70% since transitioning. Mumbai leads in terms of competition among cities like Bangalore and Chennai. At the same time, according to WEF estimates, around 133,000,000 AI jobs will be created by 2020! According to research findings, employment numbers need to catch up.AI can be applied across numerous sectors, such as healthcare, banking, finance, marketing, and the entertainment industry. Ai skills are required in jobs such as deep learning engineers, data scientists, directors of data science, senior data scientists.
Want More Information About Our Services? Talk to Our Consultants!
Conclusion of Artificial Intelligence
Humans have always been drawn to technological innovation. Right now, we live through some of the greatest advancements ever seen - Artificial intelligence software promises to revolutionize technology further. Organizations around the globe are developing revolutionary innovations in machine learning and artificial Intelligence (AI). AI not only has an enormous effect on industries and individuals worldwide; it is also driving forward new technologies like big data analysis and robotics. As evidenced by its rapid expansion rate, AI technology will remain at the forefront for some time to come. Professionals trained and certified can find rewarding careers working on this platform; AI will have an increasing effect on social settings as well as quality of life issues.