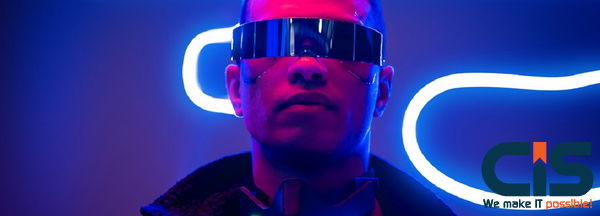
As a data scientist, you have likely encountered the term "flying blind" when working with your team. This refers to operating without well-defined KPIs (Key Performance Indicators). We're essentially shooting in the dark without clear KPIs since we don't know what to aim for.
An absence of KPIs compromises data science's integrity and makes other fields dependent on subjective performance evaluations. How can one objectively judge data visualization dashboards or machine learning models without quantifiable KPIs? KPIs for data teams provide not only performance indicators but also serve as growth and survival indicators of their company. KPIs ensure we remain strategic assets rather than cost centers while helping align the team's efforts with organizational goals.
In this post, we'll explore all of the essential KPIs to assess effectiveness, ranging from stakeholder satisfaction and project completion rates to data correctness rates and response times - everything needed for practical data team assessment. Now, let's get started.
Comprehending Data KPIs: 10 Essential Reasons
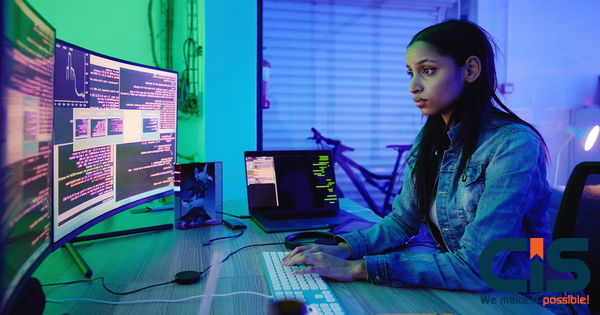
Key Performance Indicators, or KPIs for short, are quantitative measurements used to track and gauge data's efficacy, influence, and value within an organization. Data governance, quality management, analytics, and data-driven decision-making are just some of these indicators' uses. KPIs developed by our data team are intended to give organizations practical information that will assist in streamlining processes and reaching goals efficiently and successfully.
Key Performance Indicators (KPIs), devised by modern organizations' data teams to measure critical aspects of data's success across industries and business scenarios, should be emphasized. KPIs are invaluable in measuring effectiveness, caliber, and significance across many business operations. Here are several compelling arguments supporting the value of data KPIs.
- Competitive advantage
- Increased data quality
- Better decision-making
- Demonstrating ROI
- Objective measurement and accountability
- Identifying areas for improvement
- Resource optimization
- Strategic alignment
- Risk management
- Customer satisfaction
Let's explore each step in detail:
Competitive Advantage
Businesses can achieve a competitive edge by efficiently measuring and acting upon KPI data. Improved data analytics can result in improved market analysis, giving companies an edge against their competition.
Increased Data Quality
KPIs that measure data quality - like timeliness, correctness, and completeness - help organizations maintain high-quality information. Top-quality data is critical for effective analytics, reports, and making informed business decisions.
Better Decision-Making
Data science metrics for effective decision-making empower decision-makers with the essential metrics required for making more informed and strategic choices. KPIs are a quantitative basis when selecting which projects or tools should take precedence or invest in something new.
Demonstrating ROI
Projects including data can demonstrate their return on investment (ROI) by tracking KPIs related to data initiatives and showing their measurable advantages through costs and effort analyses. Organizations can confirm this with data KPIs, which also help quantify those advantages measurable against costs involved with data initiatives.
Objective Measurement And Accountability
KPIs provide governance, analytics and data management projects on an unbiased basis to measure their efficacy. Teams and individuals within an organization who manage data will become more accountable.
Identifying Areas For Improvement
KPIs can assist with pinpointing areas for improvement by helping identify inefficiency in data management or usage as well as performance gaps or deficiencies. Step one in taking corrective actions and streamlining processes is identifying key problem areas.
Resource Optimization
Organizations can optimize resource deployment by monitoring KPIs to identify where personnel, money, and time should best be applied. Automating or restructuring processes could be appropriate if data processing operations take a long time to complete.
Strategic Alignment
Data KPIs help organizations ensure their data-related activities align with their larger business goals. Businesses should ensure their data strategies are successful and targeted toward meeting key company goals by setting and monitoring key performance indicators (KPIs).
Risk Management
KPIs related to data governance can help facilitate risk management by monitoring compliance with data-related legal and regulatory obligations. Providing this level of data protection can be especially important in industries where information management procedures must adhere strictly to government regulations, such as healthcare or banking.
Customer Satisfaction
Certain KPIs could have an indirect or direct effect on customer happiness. Accelerated data processing may lead to faster customer service response times, while improved analytics can produce tailored customer experiences.
Data KPIs are indispensable for analyzing how an organization handles and utilizes its data. Their implementation helps companies align their strategy with overarching business goals effectively and efficiently and effectively align their data strategy with measurable KPIs.
Read More: Data Science for Software Development - Worth the Investment?
5 Key KPIs For Any Data Team
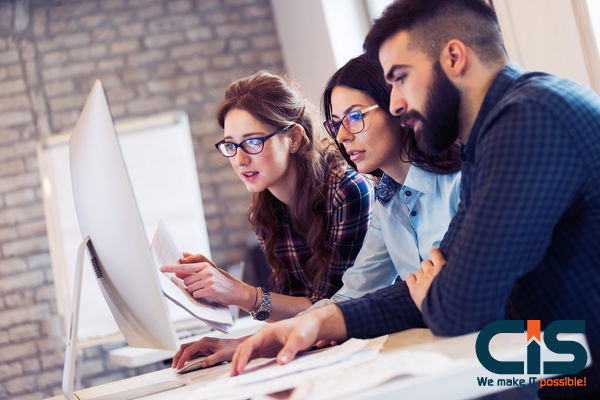
There is no universal definition of "5 key KPIs," as each business, institution, or situation requires unique KPIs for success. KPIs often reflect business goals and strategies employed within an organization.
Here are five KPI categories that are often seen as essential components for data teams:
- Revenue-based KPIs
- Customer KPIs
- Operational efficiency KPIs
- Employee performance KPIs
- Marketing and sales KPIs
We will examine each type in greater depth:
Revenue-Based KPIs
- Examples: Customer Lifetime Value, Year-over-Year Growth, and Monthly Recurring Revenue (MRR)
- What it measures: These KPIs track your organization's revenue generation directly.
- Why it's crucial: Understanding sales data is essential to evaluating a company's financial status and prospects for expansion.
Customer KPIs
- Examples: Customer Retention Rate, Net Promoter Score (NPS), and Customer Acquisition Cost (CAC)
- What it measures: These KPIs assess how successfully a business draws in, pleases, and retains clients.
- Why it's essential: Long-term business success depends on maintaining and increasing customer happiness.
Operational Efficiency KPIs
- Examples: Inventory Turnover, Efficiency Ratios, and Operational Costs
- What it measures: Operational efficiency KPIs for streamlined processes evaluate how effectively an organization utilizes its financial resources, time, and personnel.
- Why it's essential: Competitive advantages and higher profit margins are two benefits of streamlined operations.
Employee Performance KPIs
- Examples: productivity metrics, employee engagement levels, and employee turnover rates.
- What it measures: These key performance indicators evaluate workers' job satisfaction and performance.
- Why it's important: An engaged and effective crew frequently sets successful businesses apart.
Marketing And Sales KPIs
- Examples: sales cycle length, conversion rate, and cost per lead.
- What it measures: These KPIs assess how well sales and marketing initiatives work.
- Why it's important: Gaining insight into these indicators enhances sales tactics and optimizing the marketing budget for increased return on investment.
Some KPIs may be significant depending on objectives, sector considerations, and other relevant variables. Measures such as Monthly Recurring Revenue and Customer Churn Rate are critical metrics for SaaS (Software as a Service) companies, while manufacturing firms may focus more heavily on operational efficiencies and inventory turnover rates.
KPIs For Data Science Consultant
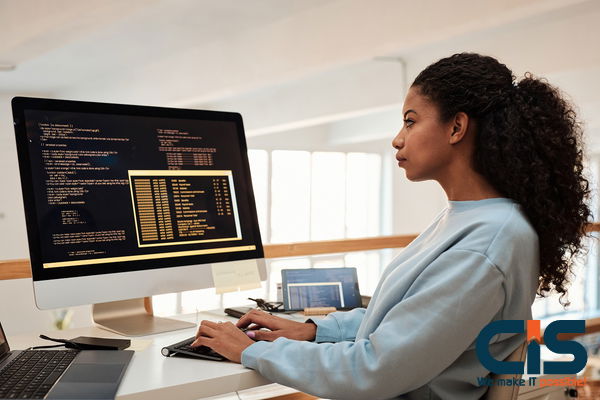
Data science teams play a vital role when harnessing data for business goals such as customer engagement and operational efficiencies. Key Performance Indicators (KPIs) objectively measure team performances, accomplishments, and contributions toward corporate goals. Below are the critical KPIs your data science team should keep in mind.
- Code quality metrics
- Stakeholder satisfaction
- Time-to-model deployment
- Business impact metrics
- Data latency
- User adoption rates
- Error rate
- Model accuracy
- Model retraining frequency
- Data coverage
Let's delve further into each topic individually.
Code Quality Metrics
- What it measures: Variables such as the number of errors per line of code, cyclomatic complexity, and code duplication.
- Why it's essential: Higher code quality frequently results in fewer errors and more straightforward maintenance, which lowers the overall expenses of data science initiatives.
Stakeholder Satisfaction
- What it measures: Comments or satisfaction ratings from internal or external stakeholders who work with or rely on the data science team.
- Why it's essential: High satisfaction means that the data science team is performing up to or better than expected, which will probably lead to more resources and support in the future.
Time-To-Model Deployment
- What it measures: The time between the start of a data science project and the model's deployment in a real-world setting.
- Why it's essential: Realizing commercial value may occur faster with faster deployment times.
Business Impact Metrics
- What it measures: The direct effect of the data science project on important business indicators like client retention rate, cost savings, or revenue growth.
- Why it's important: It provides stakeholders with a quantified ROI and highlights the importance of the data science team.
Data Latency
- What it measures: The amount of time that passes between the creation of data and its availability for modeling or analysis.
- Why it's important: More real-time analysis and decision-making are possible with reduced latency.
User Adoption Rates
- What it measures: The pace at which data science products (such as tools, dashboards, and models) are adopted or used by internal or external consumers.
- Why it's essential: High adoption rates typically signify the value and significance of the data science team's product.
Error Rate
- What it measures: The rate or frequency at which data models produce errors, inconsistencies, or false positives or negatives.
- Why it's important: A lower error rate indicates more dependable outcomes and increased confidence in model outputs.
Model Accuracy
- What it measures: A machine learning model's accuracy rate regarding classifications or predictions.
- Why it's essential: The efficacy of business decisions that depend on an accurate model is frequently closely correlated with that effectiveness.
Model Retraining Frequency
- What it measures: The frequency of model updates or retraining.
- Why it's essential: Model retraining frequency in dynamic data environments is crucial for ensuring the sustained accuracy and relevance of models. Regular retraining becomes particularly essential, especially when dealing with dynamic situations.
Data Coverage
- What it measures: A model or analysis's coverage % of the data landscape.
- Why it's important: More precise and reliable insights are typically possible with extensive data coverage.
Every KPI provides a framework to assess how well your data science team is doing, but when tailored specifically for your organization and its goals and business objectives, they come alive. Periodic evaluations must ensure KPIs remain compliant with ever-evolving business needs to keep KPIs aligned.
Conclusion
For data science to achieve maximum business impact, Key Performance Indicators (KPIs) must be strategically deployed. KPIs offer more than statistics; they're tangible insights supporting ongoing optimization, skill enhancement, and overall business success. Consistent observation ensures data teams not only evaluate their value proposition but also discover opportunities for innovation and growth, strengthening their role as dynamic assets within an organizational environment.