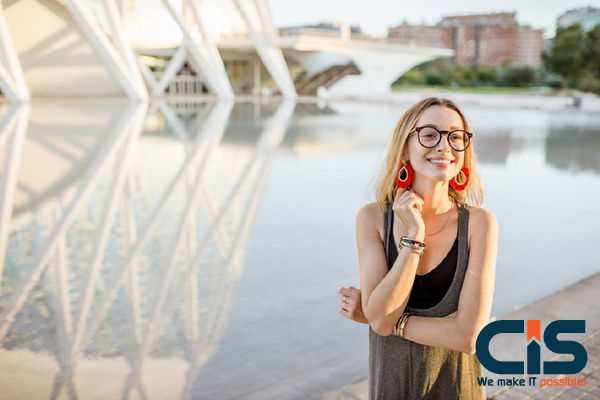
Artificial Intelligence (AI) has revolutionized both cancer research and therapy through its ability to recognize complex patterns within medical data sets and offer quantitative evaluations of clinical situations. AI can offer tremendous potential to transform traditional data analysis techniques in order to access all of the medical data that now exists.
Oncologists use AI algorithms for image analysis and radiology, making early cancer identification and precise diagnosis possible. Furthermore, AI ensures eligibility criteria are evaluated using data-driven techniques, which allows more inclusive trial designs while upholding patient safety precautions - thus making AI an indispensable element in radiation therapy treatments. Here in this blog post we explore AI's Role In Radiation Therapy.
Artificial Intelligence
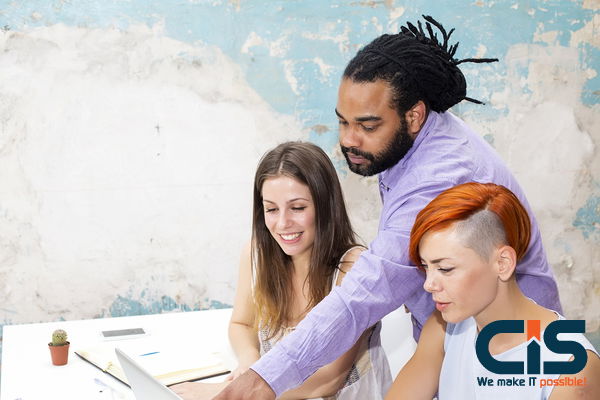
What is Artificial Intelligence? Artificial Intelligence is a branch of computer science which deals with creating intelligent agents capable of performing tasks that typically require human intelligence - including problem-solving, speech recognition and decision-making among others.
Artificial intelligence refers to an umbrella of technologies that enable computers to perform advanced tasks, including visual perception, language comprehension and translation, data analysis, question answering, artistic creation, video generation, and many others. Artificial Intelligence is the backbone of modern computing; for example, it effortlessly transforms text prompts into high-quality images, facilitates autonomous driving in vehicles, and engages customers via AI chatbots - to name a few examples.
AI is an interdisciplinary science with several approaches. AI systems may follow predetermined rules or use machine learning algorithms to adapt their operation based on environmental changes. Still, machine learning algorithms provide AI systems with greater adaptability as they learn from data sets, making their intelligence even more versatile and capable of handling unforeseeable scenarios.
AI And Cancer Detection
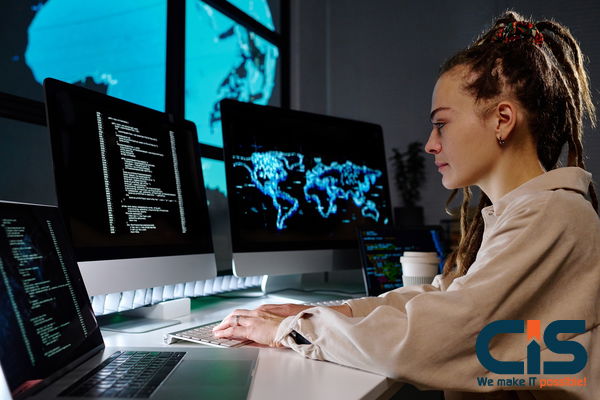
Early and accurate cancer diagnosis has long been difficult; AI offers hope in significantly increasing cancer detection, as evidenced by over 20 FDA-approved AI models for mammography screening by 2024.
Technology and medicine combine to transform oncological treatment. Traditional cancer diagnosis relied heavily on biopsy, histological examinations under microscopes, imaging tests such as MRI, CT Scan, as well as specific diagnostic procedures which could be invasive or uncomfortable - these traditional approaches could vary among professionals in their interpretation of results, and specific diagnostic procedures can sometimes even cause discomfort to the patient.
AI systems employing deep learning can analyze medical images with striking precision, making their analysis far superior to human eyes. When trained on vast public cancer data sets, these AI programs can identify minute anomalies often missed by human eyes while decreasing false negatives. Furthermore, by mining large sets of patient records for potential risks for specific forms of cancer, such as breast and skin cancer, due to family history, obesity, or workplace hazards that pose additional threats, early screenings might become feasible and allow early interventions.
AI holds great potential to revolutionize cancer detection. In collaboration with the USA National Health Service, Google's project produced an AI system that outwits human doctors at spotting more than 50 eye diseases using 3D scans, making the implications of similar technology immense regarding CT or MRI tumor spotting capabilities. PathAI employs AI pathology platforms to diagnose diseases, including cancer. It helps pathologists detect patterns more effectively so that all that can be identified are detected successfully.
Radiotherapy Planning
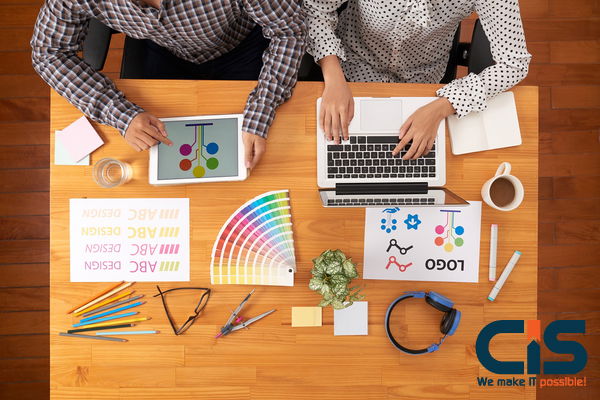
AI has long been utilized in radiotherapy planning. Second-generation AI systems utilizing an expert system approach that responds to conditioned reflexes by teaching AI knowledge via rules have made IMRT planning much more widespread than before. Treatment planning for IMRT often relies on reverse planning techniques that involve optimizing dose distribution (or fluence map) calculations to ascertain how multi-leaf collimators work and to calculate an exact final dose distribution.
However, optimizing requires repeated trial-and-error by the treatment planner, with hours spent planning. Furthermore, its quality may depend on their skill level as it impacts overall treatment planning quality. Knowledge-based treatment planning (KBTP), an intelligent machine learning model, has been widely implemented within commercial TPSs since 2024 and continues to gain acceptance today. Knowledge-based treatment planning (KBTP) is a system that uses past planning data with TPS to generate semiautomatic treatment plans in hours rather than days - significantly cutting planning times from days.
Digital linear planning (DL) recently emerged as an innovative new solution to treatment planning. Over the last five years, 257 reports and reviews on dose linear optimization. Dose linear methods learn contour and dose distribution inputs into CT treatment planning software before automatically creating dose distributions by entering new contours into it; their methods have also been reported as producing plans comparable to knowledge-based planning methods, making DL an attractive way of fully automating planning from segmentation through optimization in hours, minutes or even seconds.
Quality Assurance And Control
Once IMRT treatment planning is completed, dose verification requires quality control (QC). Dosimeter measurements or multidimensional detector measurements typically take several hours for staff. Recently, research was done using machine learning technology to predict results of gamma analysis using 2D detector measurements, shortening the time spent performing quality assurance using deep learning as part of radiotherapy therapy QA. Results indicate this approach is a promising future direction in clinical radiotherapy practice.
Fulfilling The Plan
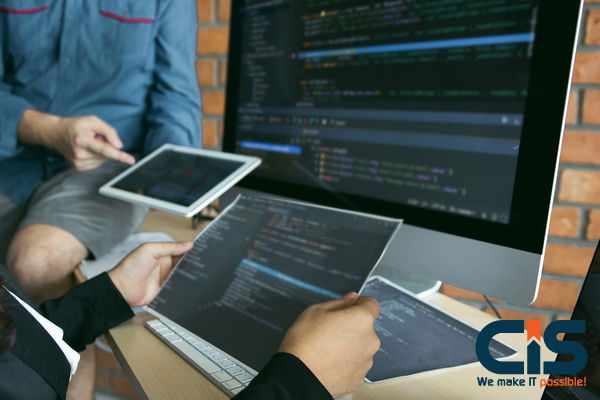
Radiation Therapy Guided By Images
AI has also been researched in radiotherapy treatment rooms. Before being exposed to radiation with a treatment beam, patient positions are verified using image-guided radiation therapy (IGRT). Cone-beam computed tomography (CBCT) images used for radiation oncology treatments tend to exhibit lower soft tissue contrast and an elevated noise ratio compared to CT images, compromising accuracy during image registration. Since 2024, researchers have investigated U-Net and CycleGAN technologies to improve image quality.
Generating CT-like images from CBCT by learning the conversion between treatment-planning CT images and CBCT images has been proposed, while dynamic tumor tracking irradiation may also be employed when moving tumors such as lung or liver cancer are detected on scans. At present, irradiation has either been carried out using an observer to observe a gold marker close to the target area or a correlation model created from external markers placed on the abdominal wall.
However, these methods have some drawbacks that hinder accuracy, such as being invasive and not always correlating an external marker with tumor positioning. Therefore, research efforts are underway to develop real-time image tracking with no external markers and improve tumor prediction using AI that compensates for the time delay of devices.
Adaptive Radiotherapy
Finally, an emerging technology known as adaptive radiotherapy--online adaptive radiotherapy, to be specific which automates image acquisition, treatment planning (modification), and delivery of radiation therapy while the patient remains on their treatment bed, is now being deployed commercially.
Initial implementation of this method involved using an MR-Linac, an apparatus capable of accurately providing images for tumor and soft tissue imaging prior to treatment. However, CT data is indispensable when it comes to dose calculation; thus, AI technology has been created and implemented in clinical settings to generate virtual CT images from MRI scans. Although limited in scope and imperfect execution, this technology shows promise as an advancement tool.
On the other hand, in this latter method, CBCT is employed for adaptation; however, dose calculation cannot be directly performed on acquired images due to limitations such as limited field of view, incorrect CT value, or increased image artifact. The current CT image generation system utilizes mutual information to deform pre-treatment planning CT and CBCT images. AI supports segmentation and adaptive planning workflow to reduce the time needed to generate virtual CT image data. Over the last five years alone, 257 papers, 15 reviews included, have been published regarding this subject area, showing high hopes and high hopes for further advancement.
AI-Based Prognosis Prediction
Reports regarding radionics and prognostication have increased dramatically over recent years as more publications in radiotherapy have been made public. While studies focused on lung, head, and neck cancer were done for overall survival (OS), rectal cancer has received the most significant number of publications.
As part of this strategy, patients diagnosed with locally advanced rectal cancer typically undergo both pretreatment and posttreatment MRI scans that analyze pathological results; over time, this will allow researchers to predict complete response at surgery from pre or post-CRT images alone.
Artificial Intelligence And Pharmacologic Therapy
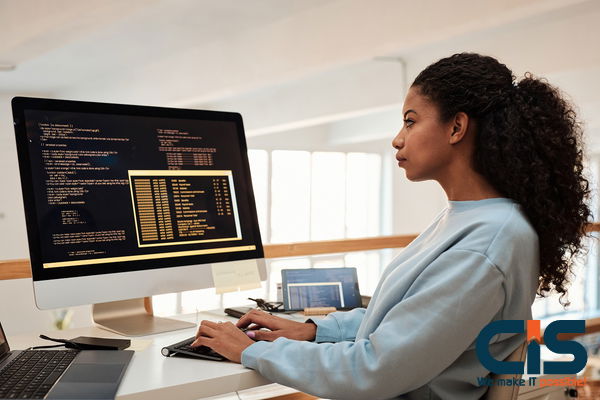
Artificial Intelligence and Machine-Based Learning (MBL) have proven capable of accurately predicting which cancer treatments a patient might respond best to, according to research & data from 48 cell lines as well as 53 cancer cell models and 36 primary cases of AML. MBL can assist in prioritizing drugs by supplementing clinical analysis and mutation analysis.
The use of electronic systems for chemotherapy prescriptions has resulted in the accumulation of vast quantities of patient data for analysis. MBL, for instance, can predict individual risk for neutropenia. Compared MBL against logistic regression in creating a web app to predict associated risk predictions.
This provided valuable insight that will aid efforts in creating drug combination treatments, potentially improving treatment outcomes, and breaking drug resistance patterns. Study findings among 20,506 cancer patients revealed that MBL interventions could significantly enhance cancer care delivery, leading to significant increases in serious illness conversations for high-risk individuals and reducing end-of-life systemic therapy in outpatients with cancer.
AI's And Cancer Treatment's Challenges
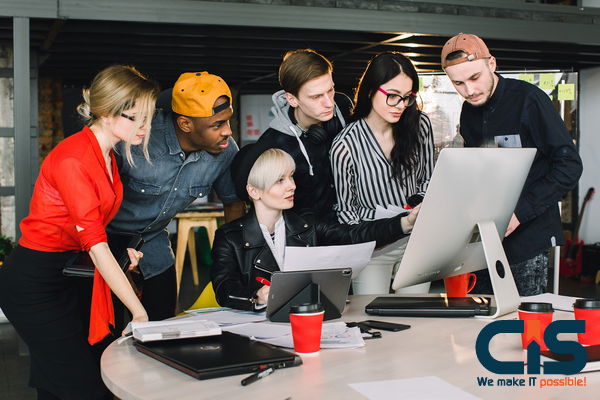
Regulation challenges of AI in cancer treatment must be tackled to ensure AI technology can be safely used within health care, with the FDA's efforts at creating monitoring frameworks for AI technologies proving an essential first step towards reaching this goal. By considering ethical and practical considerations when developing solutions using AI for cancer therapy, AI could become one of the most valuable weapons against cancer.
AI's true value does not lie in its potential to replace human expertise; health care providers possess invaluable experience and intuition that AI simply cannot duplicate. An ideal scenario would involve collaboration where AI assists physicians instead of taking over.
However, AI tools do raise regulatory concerns. According to research, over 60 medical devices or algorithms that use AI were approved by the FDA as of 2024. MBL algorithms continue to adapt as new data arrives; therefore in response the FDA created an artificial intelligence monitoring framework.
Impact Of AI On Radiotherapy
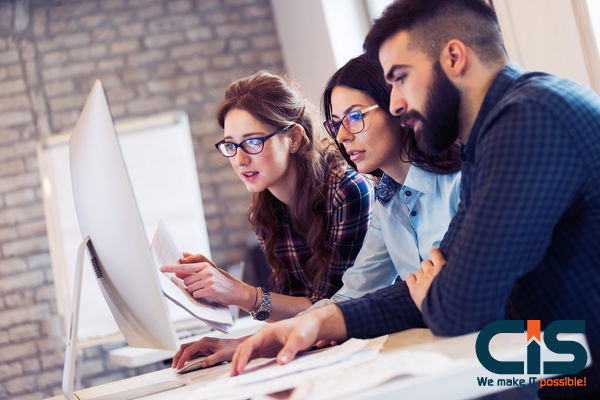
There is much Impact of AI on Radiotherapy, AI's use has extended beyond optimizing clinical trials to aid patient recruitment by aligning trial criteria more precisely and optimizing recruitment through real-time monitoring and integrating electronic health records for targeted recruitment of participants in clinical trials. Notably, precision medicine has become an international trend; precision medicine refers to selecting medical treatments based on vast amounts of medical information such as genome information; it then uses this process for selecting optimal therapies - providing customized patient management through AI technology.
Future Perspectives Of Artificial Intelligence In Radiation Oncology
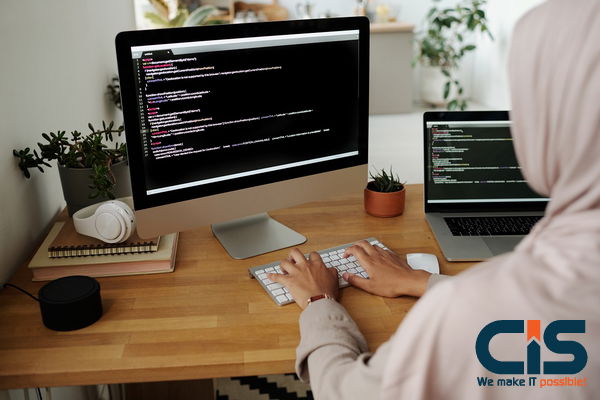
AI's ability to rapidly accelerate segmentation-planning workflow is unquestionable; however, efforts still must be undertaken to address interobserver variations in tumor segmentation, as this remains an area for potential improvement. Utilizing AI for OAR segmentation can be an immense asset when applied daily; more significantly, AI plays a crucial role in standardizing treatment when conducting large clinical trials.
In-depth analysis of data from the RTOG0617 trial to assess how radiation dose escalation affects survival among inoperable non-small cell lung cancer patients, using data from this clinical trial designed to test this theory. Studies comparing cardiac segmentation between patients enrolled in clinical trials and auto-segmentation using the DLR algorithm found that cardiac dose calculations derived via auto-segmentation tend to be higher and correlate more strongly with OS.
The use of MRI in treatment planning not only reduces radiation exposure but also allows for precise contouring of the rectum and uterus, which is difficult to isolate with CT scans while providing precise contouring. Unfortunately, CT-MRI conversion using AI may be required due to a simple conversion table between electron density and signal intensity for images acquired with an MRI scanner. Various challenges must still be faced; however, bone densities vary between individuals, which will need to be overcome as part of any solution.
However, as research on MRI to CT conversion advances, clearly delineating soft tissues like rectal from subtle differences in density in CT may become possible, providing a similar level of segmentation even in countries without access to an MRI scanner and must use CT for treatment planning instead. Integrating AI into radiotherapy could eventually become standard worldwide cancer care practice.
Want More Information About Our Services? Talk to Our Consultants!
Conclusion
Though Artificial intelligence solutions used in radiotherapy still present numerous issues for consideration, its introduction into treatment represents an important step toward standardizing it. Auto-OAR segmentation has nearly reached completion, and Deep Learning may soon fully automate planning from segmentation through optimization in short order.
AI now assists patients during adaptive radiotherapy treatments as it guides patients with essential information. By delegating tasks related to planning, delivery, and data collection to AI assistants, more time can be dedicated to patient care, allowing us more meaningful conversations resulting in improved treatment outcomes.