In 2025, the fintech world is booming, and more businesses are tapping into this digital transformation. From managing personal finances to offering investment opportunities, fintech apps are changing the way people interact with their money. The dem
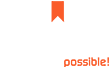
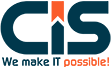