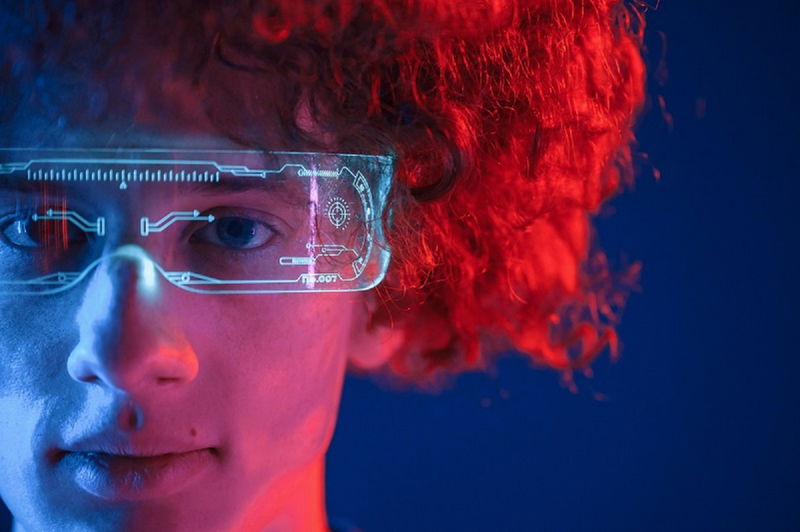
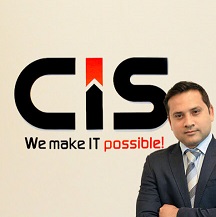
Contact us anytime to know more β Kuldeep K., Founder & CEO CISIN
But can we trust algorithms when it comes to detecting fraudsters attempting to take over our company or understanding machine learning? Read this post for all of your answers.
What Is Fraud Detection with Machine Learning?
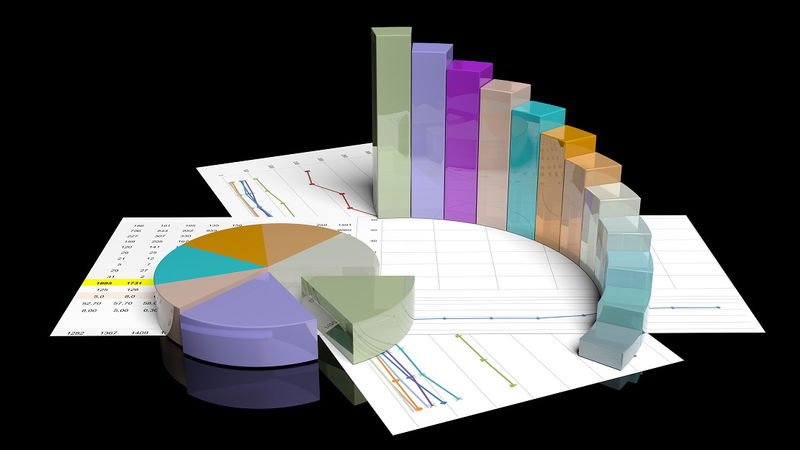
Machine learning in online fraud detection and prevention refers to an artificial intelligence algorithm that utilizes your past data in order to produce risk rules that may help block certain actions by users - for instance, suspicious logins or transactions as well as identity theft or fraud - by applying those custom rules against suspicious logins, transactions or identities of potential fraudsters.
To minimize false positives and enhance the precision of risk rules, it's critical to identify prior cases of fraud rates.
Running more algorithms increases the precision of rule suggestions.
Are There Any Differences Between Machine Learning & Artificial Intelligence (AI)?
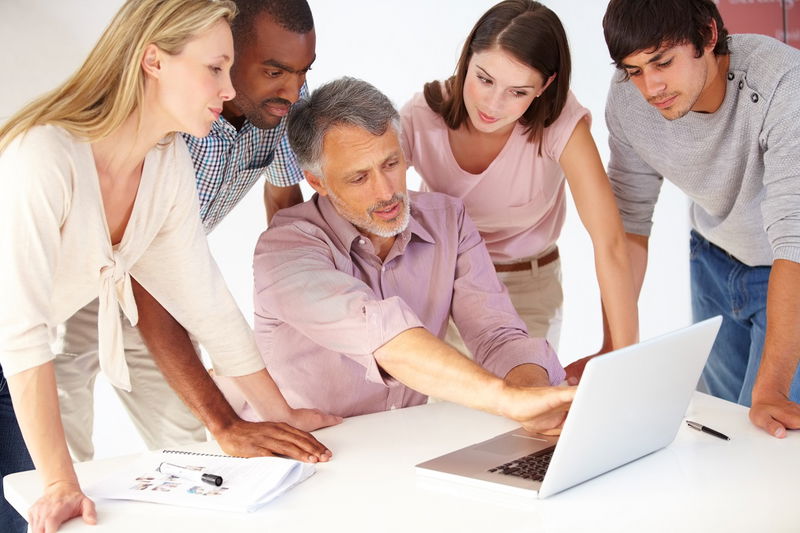
Although these terms can often be used interchangeably, all forms of machine-learning fall under AI's purview; not all AI is founded upon machine learning, though.
Artificial intelligence and machine learning is an umbrella concept with the objective of building machines capable of mimicking human thought and simulating our mental processes.
AI entails developing machines capable of simulating our thinking patterns.
Deep Learning is an approach to machine learning in which algorithms and structures are derived from brain imagery.
Machine Learning and Fraud Management
Machines excel at processing massive data sets more effectively than humans can, which allows them to handle enormous quantities of information with relative ease.
What this means:
- An advanced and more efficient detection system. It quickly recognizes suspicious patterns and behaviors which would take human agents months or even years to spot on their own.
- Reducing Manual Review Time: Machine-based data analytics tools can also dramatically cut back the amount of time that's spent manually reviewing information.
- Large datasets can lead to improved predictions: as you provide machine learning engines with more data, they become increasingly efficient at making predictions. Although large datasets make it more challenging for humans to recognize patterns within them, AI systems have the capability of doing just the opposite.
- Alternative to hiring additional RiskOps agents. You only require one machine learning system, which will process all your data regardless of its volume - an ideal solution for companies experiencing seasonal increases or decreases in traffic, signups or checkouts. Machine learning systems offer powerful support when scaling businesses without increasing risk management costs.
Algorithms don't need breaks, vacations or sleep. Even experienced fraud managers can face an overwhelming backlog when arriving at work each Monday morning, but by using machines for sorting cases that clearly constitute fraudulent activities from those that should not, this process can be expedited considerably.
Machine Learning and Fraud Detection: Disadvantages
Even with its many advantages, the manual review may still be preferred in certain instances.
- Keep in mind that black box machines may make mistakes without anyone ever realizing.
- False Positives: An action marked as fraudulent that goes undetected can significantly undermine an entire system. A machine-learning engine that has not been calibrated properly could create a feedback loop whereby results become less accurate due to false positives going undetected for too long.
- Psychology can provide valuable insight when trying to comprehend why certain user actions seem suspect; use it as an aid when seeking answers for suspicious activity from potential clients or users.
Blackbox Machine Learning and Whitebox Machine Learning: Differences
Machine learning has quickly become one of the cornerstones of fraud prevention companies, yet not all solutions provide equal levels of protection.
There is an important distinction between black-box and white-box machine learning approaches that need to be considered before choosing which to invest in.
- Blackbox machine-learning: This system makes decisions automatically and transparently, perfect for smaller businesses that prefer not to deal with changing risk rules manually.
- Machine Learning Whitebox: When applying whitebox machine learning, you will receive clear reasons as to why risk rules were proposed, making identifying risks much simpler while giving fraud managers greater scope to adjust their strategy accordingly.
Each system comes with its own set of benefits and drawbacks; we chose one with an open "white box", giving risk managers more freedom and the ability to test and refine each rule as they see fit.
Want More Information About Our Services? Talk to Our Consultants!
Machine Learning Fraud Prevention: How To Use It

Machine learning may seem complex at first, but getting started can be straightforward. Here's an easy example that focuses on decreasing chargebacks (and thus transaction fraud):
Input Data Feed
Starting an AI system or machine learning model from scratch requires data. Transactional records could include information like this.
- Transaction Value
- product SKU
- Types of Credit Cards
We plan on including information regarding customer activity when accessing our website.
- Data protection
- Device type
- Tor, VPN or proxy usage
With more data at your disposal at the outset, your results should improve exponentially. This is particularly important if your fraud prevention program does not support custom fields; otherwise, you could miss important pieces of info!
Creating The Rules
Machine learning reveals two classes of rules.
- Heuristic or single-parameter rules are also called single-parameter rules. An example would be to block the traffic if IP is X.
- Multiple parameters: complex rules
The accuracy thresholds allow trigger conditions to be modified accordingly.
Rule names have been written with descriptiveness in mind to allow quick understanding. All rules were developed with the objective of understanding how customer login could influence amounts lost due to fraud.
Re-examining and Activating a Rule
Filter rules by data points such as type or predicted accuracy - calculated via an intricate confusion matrix.
Machine-learning suggestions by default are disabled, making the ON/OFF button the fastest and simplest way to activate them quickly.
Furthermore, threshold adjustments allow for custom trigger rules.
The Algorithm For Training
Feedback data is central to improving accuracy and refining rules, with two strategies for labeling actions and providing feedback:
- The GUI is a visual, simple way to indicate actions
- Label API: You can programmatically mark actions via API calls
Mark an action as either approved or reviewed to indicate its completion.
Every day, our algorithms are retrained using 180 days' worth of historical data and made available 24/7 in both the backend (where risk rules are managed) and the scoring engine.
Test Rules Using Historical Data
An effective fraud prevention program should enable you to look back over past cases and see whether rules could have been more effectively applied with software offering on or off switches for each rule and measuring their efficacy.
This test creates a confusion matrix from previous transactions over the time period selected and displays an accurate estimate for any rule or regulation applied by using them.
A confusion matrix (also called an error matrix or failure matrix) is a table that visually represents an algorithm's performance and allows you to calculate its accuracy over any date range from just today or last year.
Fraud managers now have full control of their risk management strategy and can take direct measures to decrease risks while monitoring, testing and adapting results as desired.
Outsourced Machine Learning Fraud Detection vs On-Site
Machine learning models can be created on your own, though doing so might require a significant investment of both effort and funds.
- Hire data scientists, machine learning specialists, and engineers in order to build models.
- Prep/clean raw data - it could take 60-80% of your time before being ready to provide risk rules
- Outsourced machine-learning engines may benefit from data shared among multiple clients, although this doesn't equate to applying rules equally across competitors; vendors could leverage industry insight to formulate highly precise rules.
- Integral Solutions Aren't Universal: Machine learning integration into risk management strategies can be both time-consuming and expensive, requiring careful planning.
Read More: Artificial Intelligence vs Machine Learning and the role of AI
Five Use Cases for Machine Learning in Fraud Detection

Artificial intelligence development powered fraud detection technologies don't belong exclusively to one industry sector or another; all they require for proper functioning is data, so this type of tech can be found across various vertical markets.
Online Stores and Transaction Fraud
Analyzing data from thousands of transactions is no simple task, which is why fraud managers at large eCommerce sites employ machine learning techniques in order to determine why certain transactions were not detected as potentially suspicious by the system.
Anti Fraud protections have never been more critical. Juniper Research predicts that online retailers could lose as much as $50.5B due to fraud by 2024, making these issues all the more pressing.
By running an ML simulation, you will discover which items fraudsters most want, the type of shipping data which poses the greatest risks and which cards should be blocked due to high chargeback rates.
Financial Institutions and Compliance
Fintech firms, traditional financial institutions and insurance companies must abide by stringent regulations in order to avoid fines for engaging with fraudulent users and protect genuine users by being sure that only genuine users interact with them.
To remain competitive, businesses must work quickly if they hope to remain successful and avoid fraudulent profiles from passing unnoticed.
Many can gain valuable insights by employing machine-learning systems.
iGaming Bonus Abuse and Multi-Accounting
Casinos, betting sites and online gaming platforms all share responsibility for making sure those playing are genuine players.
New customers are frequently awarded high-value prizes that entice newcomers. Unfortunately for fraudsters, however, such rewards force them to open multiple accounts at the same time while engaging in collusive behavior and fraudulent conduct - thus opening multiple accounts simultaneously and receiving signup bonuses without taking an honest stance with regard to customer accounts, and bonuses claimed - making fraudulent activity increasingly likely.
TransUnion recently reported an alarming 43% surge in online gambling fraud during 2021, showing the necessity of more stringent measures being put in place than ever.
Machine learning systems use data analysis techniques to detect suspicious activities in real time, providing the perfect way to spot poker bots or cheating players.
BNPL (Account Takeover) and BNPL Attacks
Accounts that allow for payments later are quickly evolving into digital wallets that fraudsters use to purchase goods and services illegally if they gain access to one of these user accounts; ATO stands for "gaining unauthorized access".
Understanding how your users access the platform is critical to protecting their accounts, which may vary significantly based on market dynamics, seasonality and other considerations.
A machine-learning engine could further strengthen this protection for online accounts.
Payment Gateways and Chargeback Fraud
Timely transactions must be processed through payment gateways in order for human agents to review them manually.
Machine learning engines can serve as an invaluable analytical tool in the fight against fraud. Once trained, these engines can identify potentially fraudulent transactions which would otherwise incur charges.
Combining Machine Learning and Manual Reviews
The manual review becomes impossible when time is of the utmost importance; payment gateways must process as many transactions quickly as possible for human agents to manually review them all.
Machine learning engines can serve as indispensable analytical tools in the fight against fraud. Once trained, these engines can identify transactions that may involve any form of misconduct and alert authorities quickly enough for action to take.
Unfortunately, though, no algorithm will always help as much.
Whitebox machine learning systems allow for suggested solutions and data enrichment services that give a human the final say, providing greater transparency of issues and possible solutions while giving your analysts access to historical data for testing as they tweak results in a Sandbox environment.
By using both AI-driven systems that detect fraud as well as human intelligence to review suggested solutions, you can ensure you get the best of both worlds.
Machines excel at processing information and memorizing facts, but human minds still excel in applying that knowledge more efficiently.
You believe combining machine learning and insights to detect fraud online is the most effective way to combat bad actors who would cause charges otherwise.
AI Fraud Prevention and Detection Strategies

Combining AI Models That are Supervised and Unsupervised
Due to the ingenuity and adaptability of organized crime, defense efforts that rely on one analytic method won't suffice; each use case requires customized approaches for anomaly detection that best suit its unique circumstances.
Under next-generation fraud tactics, both supervised and unsupervised models should be integrated.
Machine learning's most prevalent form is known as "supervised learning", in which models are built using many "labeled transactions".
Every transaction either falls under two categories - fraud or not - so models must be trained to identify patterns that best represent legal activity by using vast quantities of transactional data.
Model accuracy can be directly tied to the amount and quality of training data utilized when creating a supervised algorithm.
Models without supervision are created to identify abnormal behaviors when transactional data labels are limited or absent, necessitating self-learning methods in order to discover patterns missed by traditional analytics.
In Action: Behavioral Analytics
Machine learning techniques are employed in behavioral analytics to predict and understand behavior at every aspect of a transaction, from users and merchants to accounts and accounts themselves.
Data is utilized to develop user and merchant profiles that illustrate each person's habits over time.
With each transaction, profiles can be updated in real-time, allowing analytic functions to be constructed that provide precise forecasts of future behavior.
Profiles provide both financial and non-financial transaction records. Non-monetary activities could include changes of address, duplicate card requests or password reset requests.
Financial transaction data can help create patterns that show individuals their average spending velocity, preferred hours and days for transacting, the distance between payment locations as well as preferred transactions locations.
Profiles can provide a valuable snapshot of an activity's ongoing nature and may prevent transactions from being abandoned due to false positives.
An effective corporate fraud solution includes various analytical models and profiles that offer insight into real-time transaction trends.
Develop Models with Large Datasets
Research has demonstrated that data and variety play more of a key role than algorithms when it comes to machine-learning models' success, providing the computer equivalent of human experience.
Therefore, expanding the data set used for creating features of a machine-learning model could increase its prediction accuracy.
Consider this: doctors have the training necessary to treat many different patients successfully, and with this level of Learning or expertise, they are capable of accurately diagnosing within their area of specialization.
When it comes to detecting fraud, models can take advantage of their experience in processing millions of transactions.
To effectively identify fraud, it's necessary to examine large volumes of data. Doing this will enable you to recognize risk on a more personal basis and accurately estimate it.
Self-Learning AI and Adaptive Analytics
Machine learning offers consumers an effective means of protecting themselves against fraudsters, which makes account protection more challenging for consumers.
Fraud detection experts should use adaptive solutions which sharpen judgments and reactions - particularly marginal ones - and increase performance by sharpening them further.
These transactions either fall just slightly above or just below the threshold threshold.
Accuracy is of utmost importance when distinguishing between false-positive events (legal transactions that scored high) and fraudulent ones that scored lower, as accuracy helps determine a balance.
Adaptive analytics provide a better understanding of potential risk vectors within an enterprise, providing greater distinction.
Adaptive analytics systems are more responsive to fraud trends by adapting themselves automatically according to recent proven cases disposition.
This ensures an accurate identification and differentiation between frauds.
Once an analyst verifies a transaction as legitimate, this alerts the system.
Analysts can accurately represent the fraud landscape they face, even if that includes new tactics or dormant patterns of fraud which have yet to emerge.
With adaptive modeling's automated model updates feature, model changes occur without intervention by analysts.
This adaptive modeling method automatically adjusts predictor characteristics within fraud models to enhance detection and protect from new attacks, making this an efficient means to detect fraud as early as possible.
Fraud Detection Using Machine Learning Algorithms And Models

There are three types of machine learning algorithms:
- Learning under supervision
- Unsupervised Learning
- Semi-supervised Learning
- Reinforcement learning
Learning Under Supervision
Supervised Learning has quickly become the go-to approach to machine learning implementation, particularly useful for fraud detection, deep Learning in FinTech, and labeling all input data as either good or bad in supervised-learning models.
Predictive data analyses rely on training data provided; any accuracy limitation from such models depends on this training data set - meaning they cannot detect fraud that wasn't included during its training phase. Nonetheless, their implementation presents one major downside - the inability to detect new frauds not already present within its dataset training set!
Unsupervised Learning
Unsupervised learning models provide an efficient means for detecting anomalous behavior where transactional data is limited or nonexistent.
Unsupervised models constantly analyze new information, adapt their models based on their results, learn patterns from these data sets and determine whether or not they belong in legitimate operations - this form of deep Learning is commonly utilized as a fraud detection technique.
Semi-Supervised Learning
Semi-supervised or unsupervised Learning falls somewhere in between; it can be applied when labeling data would either be too costly or impossible, necessitating expert expertise instead.
Semi-supervised algorithms designed for deep learning fraud detection store group parameters even when their membership remains unknown on the assumption that patterns discovered may prove useful to fraud detection efforts.
Reinforcement Learning
Reinforcement learning algorithms help machines identify ideal behaviors within specific circumstances. As they adapt to their environment, reinforcement learning algorithms constantly look out for actions that maximize rewards while minimizing risks; for this model to adapt its behavior effectively, it needs a reinforcement feedback signal to do this effectively.
Want More Information About Our Services? Talk to Our Consultants!
Bottom Line
With artificial intelligence vs machine learning fraud detection strategies in place, digital organizations can detect automated and complex attempts for fraud quickly and efficiently using both supervised and unsupervised machine learning techniques.
As long as card-not-present transactions remain commonplace online, Banking and Retail industries face numerous fraud allegations and associated risks.
Data breaches can result from various crimes, such as email phishing and financial fraud, identity theft, document falsification and fraudulent accounts.