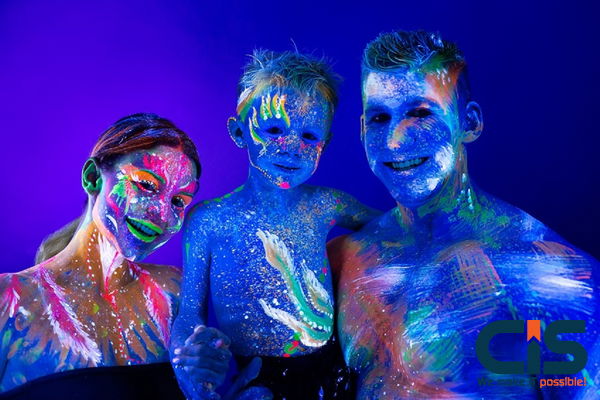
Artificial Intelligence (AI) has had an astounding effect on various fields, from driverless vehicles and language models like GPT-4 to sophisticated language models like NLP. Deep Learning is an integral component of AI; therefore this blog seeks to outline an organized method for learning deep learning - ultimately becoming an expert at it in an organized fashion.
Deep learning expands on basic machine learning concepts while reflecting more specialized expertise within this field than basic machine learning concepts do. Therefore, we suggest starting your journey by reading our blog post on why you should Learn Deep learning, Tips and Deep Learning Packages & Tools before venturing into deep learning waters.
Deep Learning
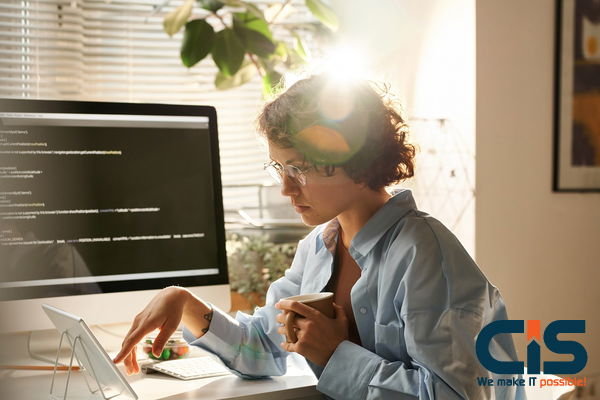
What is Deep Learning? Deep learning, an emerging branch of machine learning that mimics how the human brain processes information and forms patterns to help make decisions, is at the forefront of cutting-edge applications such as speech recognition, computer vision processing and natural language processing. Deep learning models serve as powerful AI development tools as they are capable of processing large volumes of data while understanding complex structures with neural networks featuring multiple layers - something human minds cannot.
Frequently Used Deep Learning Cases
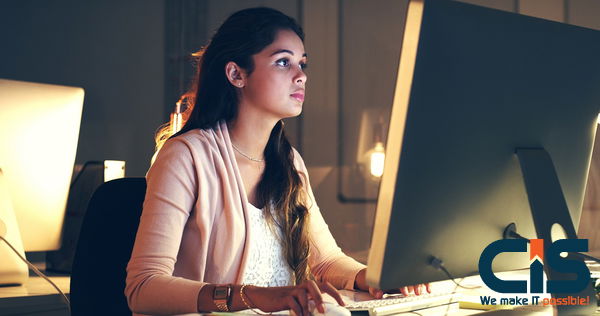
Deep learning technology powers many applications that impact our daily lives and industries. Here are some notable examples:
- Deep Learning Algorithms: Deep learning algorithms excel at recognizing patterns and objects within photos and videos, making them ideal for applications such as autonomous vehicle navigation and facial recognition.
- Natural Language Processing (NLP): NLP encompasses both producing and comprehending human language as well as technology facilitating its production, such as chatbots, virtual assistants, language translation services or language learning algorithms such as those found within ChatGPT (which use deep learning algorithms as their foundation).
- Finance: Deep learning's main applications within this domain include algorithmic trading, risk management and fraud detection.
- Voice Recognition And Generation: These systems are responsible for understanding and producing human speech, making up the backbone of voice-activated assistants that allow real-time translation between languages.
What Are Computer Vision And Image Recognition?
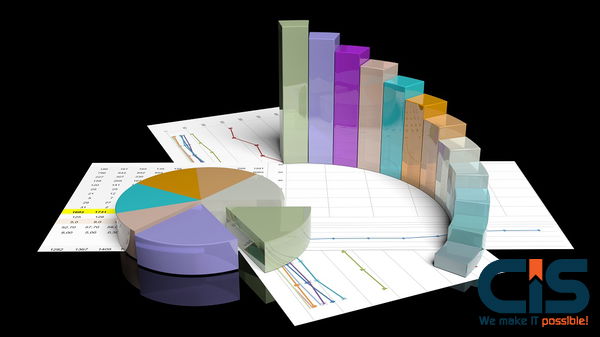
Image recognition: It refers to the practice of assigning photos to one or more predetermined classifications so apps and software dedicated to image recognition can identify objects within photos to ensure proper categorization and distinguish one object from the next.
Computer Vision (CV): Computer vision (CV) is the branch of study which seeks to give machines visual capabilities, image classification being one such task that forms part of this field and serves as the cornerstone for solving various CV issues such as those mentioned herein.
- Image classification with localization involves classifying an image while also pinpointing an object within it by drawing a bounding box around its location in the picture.
- Object detection refers to the process of classifying various objects within an image using bounding boxes to specify each one's position in relation to other items within it, much like image classification; it involves many object localization tasks as part of its overall goals.
- Object (semantic) segmentation refers to identifying which pixels in an image correspond to each object, in contrast with object detection, which involves drawing bounding boxes around every detected object.
- An instance segmentation technique involves distinguishing among objects of one class that belong to different instances within that class (individually in groups).
Researchers use deep learning models to help address computer vision challenges. Deep learning, an approach to machine learning centered on teaching computers by doing, often employs neural network topologies as its teaching method. Researchers often refer to deep learning models as deep neural networks because a majority of deep learning techniques employ such topologies for training purposes.
Why Should You Learn Deep Learning?
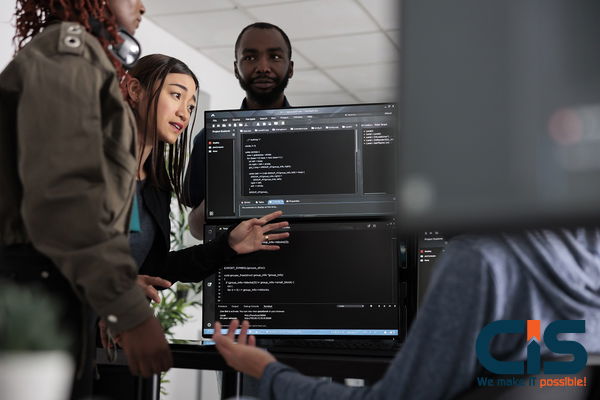
There are numerous benefits to mastering deep learning, regardless of your level of experience. Here are three explanations for this:
- Demand For Jobs: Deep learning skills are in high demand among job-seeking employers across industries; machine learning positions, in particular, have seen significant investments from several of these. When it comes to innovative and cutting-edge AI initiatives, such as robotics or AI translation services, deep learning knowledge is indispensable; therefore, deep learning skill sets have become crucial tools of career progression due to companies investing heavily in AI initiatives from all sorts of backgrounds.
- Attractive Salary: Due to the complex skill sets and knowledge bases required for deep learning professions, deep learning careers rank among the highest-paying careers within tech. An engineer specializing in deep learning typically earns between $15K-$21K annually, with potential total compensation reaching up to an astounding total compensation figure of up to 181K.
- Intellectual Challenge: Working on deep learning algorithms and processing capacity advancement is always thrilling; working in this subject area could bring revolutionary technologies that pave the way to future achievements.
How Much Time Does Deep Learning Require To Learn?
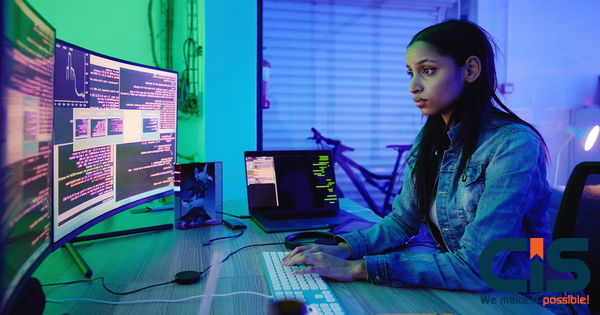
Depending upon your experience, level of commitment and choice of learning materials, there can be significant variations in the time-line required to become a deep learning master. We advise beginners starting off by learning machine learning instead - as machine learning forms the core aspect of deep learning.
If you already possess proficiency in machine learning, three to four months should be enough time for you to achieve expert status in deep learning. This estimate assumes a regular learning schedule with project-based application of acquired abilities, but deep learning's rapid advancement requires continual study and adaptation in order to remain up-to-date.
How 2024 Will Teach Us About Deep Learning
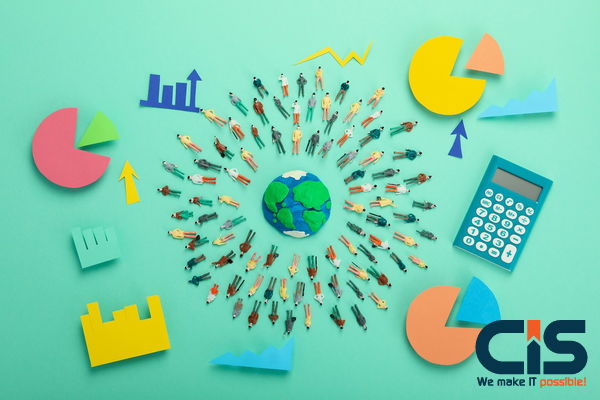
Starting the process of studying deep learning necessitates a calculated approach that combines theoretical understanding with real-world application. In 2024, you can study deep learning by following these steps.
Brush Up On The Prerequisites
Before delving deeper, a foundation must be laid. Here are the areas you must cover for successful learning:
- Basic Mathematics & Statistics: In order to fully appreciate deep learning algorithms, one needs a solid grasp of probability theory, statistics, linear algebra, and calculus.
- Data Structures & Manipulation: Working with large datasets is essential to deep learning; thus, possessing expertise in data management and processing is necessary for its successful execution.
- Machine Learning Theory & Applications: Before diving deep into deep learning, one should possess an in-depth knowledge of machine learning principles such as supervised and unsupervised learning.
Develop Your Deep Learning Skills
Deep learning requires expertise from various fields; therefore, here are the top resources and their relevance to deep learning.
- Statistics: Deep learning relies heavily on statistical principles in order to effectively interpret data, understand how algorithms behave under various scenarios, and make informed choices regarding model design and parameter values.
- Mathematics: Deep learning models, especially neural networks, utilize linear algebra, calculus and optimization as central concepts of their mathematical underpinnings. By understanding these domains fully, one can build and comprehend complex models more quickly and easily.
- Programming: Deep learning model implementation and experimentation require programming languages like Python. As libraries like TensorFlow and PyTorch abstract much of the complex mathematics involved with deep learning models, programming becomes essential as part of deep learning model deployment and experimentation.
- Deep Learning Theory: Building effective models and understanding how they learn requires having an in-depth knowledge of deep learning theory, including its operation across varying neural network topologies.
- Deep Learning Application: Preprocessing data, selecting an ideal model architecture, training models efficiently and then assessing their performance are essential skills needed for successfully applying deep learning to practical issues.
Read More: Deep Learning vs. Reinforcement Learning: Which Offers the Biggest ROI?
Discover Crucial Deep Learning Packages & Tools
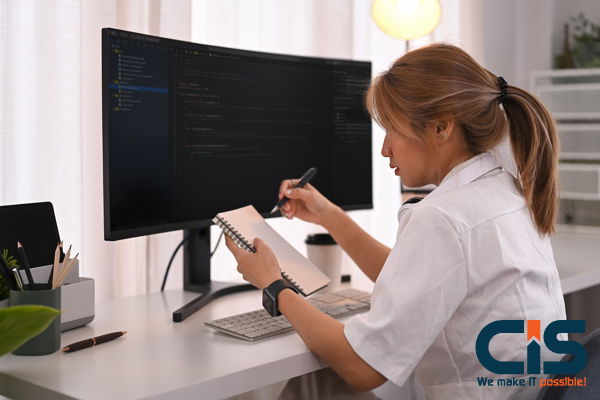
Here we have outlined the important deep learning packages and tools:
- TensorFlow: Google created TensorFlow as an open-source machine learning and deep learning framework, widely acclaimed for its adaptable training of intricate neural networks as well as providing support for an array of deep learning applications.
- Keras: Written in Python, Keras is an open-source neural network API compatible with TensorFlow, Theano or Microsoft Cognitive Toolkit (CNTK). Keras prioritizes user friendliness and versatility when designing this API, making prototyping deep learning models straightforward and swift.
- PyTorch: Created by Facebook's AI Research division, PyTorch's efficient memory utilization and dynamic computational graph make it particularly well suited for projects involving neural networks with complex structures.
- MXNet: Apache MXNet's deep learning framework was designed to be both flexible and effective, offering imperative and symbolic programming approaches to increase productivity and efficiency when creating models.
- Caffe2: Created by Facebook, Caffe2 is an easily adaptable deep learning framework with modularity that's lightweight yet scalable - offering developers and businesses alike an economical method for deploying deep learning solutions across platforms.
A Deep Learning Learning Plan Example
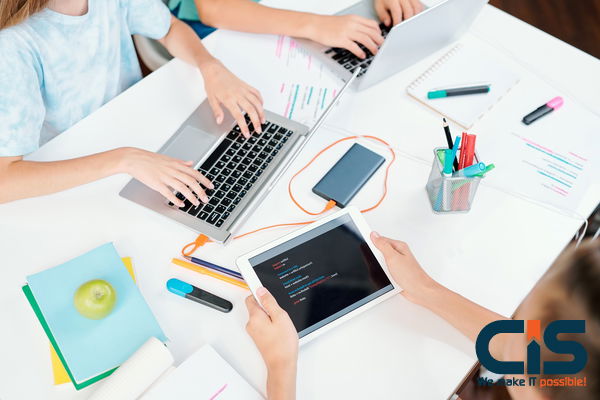
This deep learning learning plan is intended for individuals who have prior knowledge of machine learning concepts and want to enhance their skills in this specialized field. We suggest laying a strong foundation before delving into deep learning if you're just getting started or require a more basic understanding of the subject first.
Enhance Your Math, Statistics, And Deep Learning Theory In The First And Second Months
Begin by honing your mathematics abilities and broadening your understanding of deep learning theory. Recognizing and comprehending its many complexities requires dedicated time. Pay particular attention when reading about deep learning models and algorithms such as:
- Linear Algebra: Understanding these essential tools in deep learning requires knowing about matrices, vectors, eigenvalues and eigenvectors - essential in representing and manipulating data sets in this manner.
- Calculus: Pay close attention to partial derivatives and integrals when learning differential and integral calculus - these concepts will prove key for understanding optimization in neural networks.
- Probability And Statistics: Model inference and decision making depend upon an understanding of probability and statistics concepts like probability distributions, statistical significance measures and Bayesian thinking.
- Optimization Methods: Gain knowledge of key optimization techniques used in deep learning model training, such as stochastic gradient descent and gradient descent.
- Understanding Neural Network Architecture: Gaining knowledge of neural networks such as feedforward, convolutional and recurrent networks will allow you to comprehend their function better when applied to various problems.
Enhance Your Preparations In Months Three Through Six
After developing an effective foundation during your first two months, selecting a specialization should be your next step on the deep learning journey. Focusing your deep learning efforts in one domain could enable greater innovation and challenging real-world problems; consider selecting any of these topics:
- Learn The Fundamentals Of Natural Language Processing (NLP): Explore methods for sentiment analysis, text analysis, language translation and chatbot creation - with many applications such as creating intelligent AI assistants to enhance customer support services.
- Computer Vision: Investigate picture production, object identification and recognition tasks within computer vision to provide innovative security, healthcare, and driverless car applications.
- Speech Recognition: Speech recognition studies the intricacies of human speech, translating spoken language into textual forms, and building voice-activated technologies is essential to improving user interfaces and creating accessible technology products.
- Generative Models: Discover variational autoencoders (VAEs) and generative adversarial networks (GANs), two computational techniques used for tasks including picture production and style transfer, among many others. Generative models make creative possibilities in entertainment, design and the arts possible through them.
- Reinforcement Learning: Examine the principles behind reinforcement learning, which has applications across robotics, optimization issues and gaming AI. Agents learn through trial and error how to make decisions by being exposed to various levels of reward or punishment.
The Best Tips To Learn Deep Learning
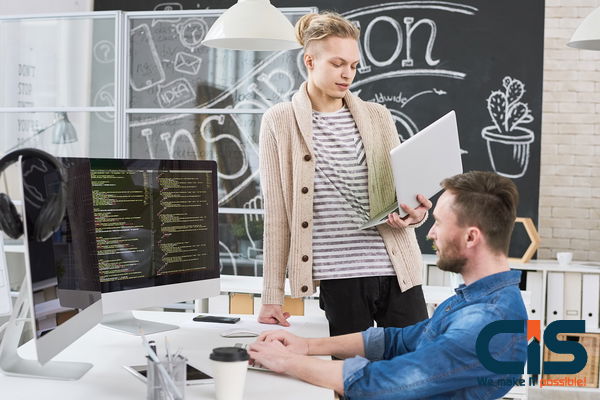
Here we have outlined the crucial tips to learn Deep Learning:
- Avoid Being Distracted By Math: While having a strong mathematical foundation is vital to successful deep learning models, it can quickly become overbearing if not managed carefully. Instead of getting bogged down with computations that seem pointless or arcane, focus on understanding ideas behind deep learning models rather than getting bogged down with computations themselves.
- Utilize Your Abilities On Projects: Utilizing what you know on practical assignments will strengthen both comprehension and abilities while producing tangible results that you can demonstrate to employers or partners.
- Engage With Communities: Connecting with communities can offer inspiration, motivation and fresh viewpoints that you might otherwise miss. Make use of discussion boards, seminars or gatherings - work together with others on improving their education - and collaborate in order to expand it further.
- Continue Iterating: In a rapidly developing field like deep learning, continual learning is vital. Don't be scared to venture into uncharted waters while reviewing fundamentals - learning iteratively will keep your abilities current and sharp.
Want More Information About Our Services? Talk to Our Consultants!
Conclusion
Beginning a deep learning journey can be both fulfilling and taxing, so making use of appropriate AI development services and adopting a practical approach will significantly shorten the learning curve. Also, keep in mind that subject matters change constantly, so to remain both current and creative in deep learning, it's best to adopt lifetime learning as part of your daily routine.