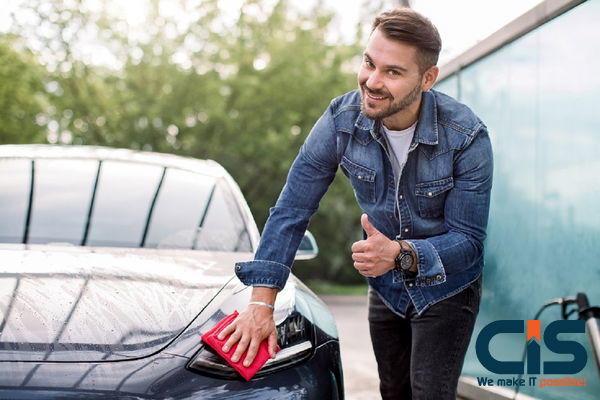
Let's define our terminology first. Neural networks are used in deep learning and machine learning to identify data patterns. It is utilized in various processes, including image recognition, natural language processing, recommendation systems, and more. On the other hand, reinforcement learning is machine learning that teaches agents how to make decisions to accomplish a certain goal.
While reinforcement learning may be goal-driven, deep learning is data-driven. One of the direct contrasts between deep learning and reinforcement learning is this. Deep learning enables algorithms to learn independently while evaluating vast amounts of data. Contrarily, reinforcement learning depends on agent feedback to determine which behaviors will result in the intended result.
This post will teach you a lot about deep learning and reinforcement learning. We'll go over their differences as well as some of their uses. We'll also discuss how to employ them most effectively in your company. For more details, see The Complete Beginner's Guide to Machine Learning.
What is Deep Learning Vs. Reinforcement Learning?
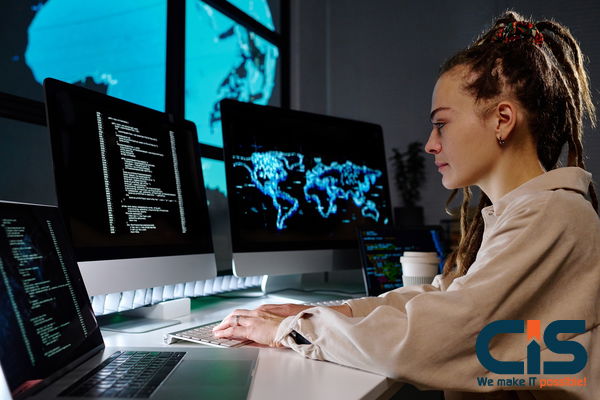
Deep learning is a vast neural network that can spot patterns in data. This concept was influenced by how the brain functions. The output of the final layer predicts the result. The network's layers each learn a separate subset of the data.
Currently, supervised learning is the foundation of deep learning models. Therefore, each data point has a label. Deep learning models need a lot of computational power because they may be applied to a lot of fresh data at once and learn from a lot of data.
This complexity used to mean that businesses had to hire data scientists and computer science specialists with skills such as Python and set up their GPU clusters. Any business user can easily build and deploy these models using no-code tools such as No-Code Tools. Deep learning is not a skill that data scientists can only use.
Deep learning improves processes in various fields, including speech recognition, self-driving automobiles, and facial identification. Large deep-learning networks frequently need a lot of data to train properly. Because of this, they are so well-liked in business applications like Siri and Google Assistant.
Deep learning also greatly influences the most recent developments in computer vision and natural language processing. These developments have led to a greater acceptance of Artificial Intelligence in the business world.
Because more data-driven AI models are more potent, this is the case. Businesses increasingly turn to deep learning to make sense of the growing amount of data generated each day.
AI of the reinforcement learning variety teaches machines to gain knowledge from their experiences. AI that uses reinforcement learning penalizes agents or computer programs for poor decisions and rewards good ones.
Deep learning requires supervision; reinforcement learning does not. Although reinforcement learning is largely unsupervised, it can produce "labels" through trial-and-error interactions with its surroundings.
Play Atari board games or train robots with this technique. Tasks that are challenging to plan for benefit greatly from reinforcement learning. The computer can make mistakes and grow from them by changing how it behaves.
AlphaGo, developed by Google, is an example of reinforcement learning in action. AlphaGo defeated the best Go player in the world by playing against itself millions of times and studying the outcomes. AlphaGo was initially taught using data from countless human Go matches.
AlphaGo was created by the DeepMind team at Google utilizing the Q-learning reinforcement learning algorithm. This value-based approach rewards the algorithm for performing the right tasks. Model-based and policy-based learning systems are just a couple of the many more reinforcement learning techniques that are accessible.
Although it is not widely understood, reinforcement learning has numerous commercial and industrial uses. Reinforcement learning defeated an experienced F16 human pilot in a series of dog boots. Robots are currently being taught how to move objects with them.
As the algorithms improve, we expect to see more practical and impressive reinforcement learning applications.
Read More: Machine Learning And Deep Learning Are Becoming Increasingly Important For Businesses
What Can You Do to Apply this Principle in Your Business?
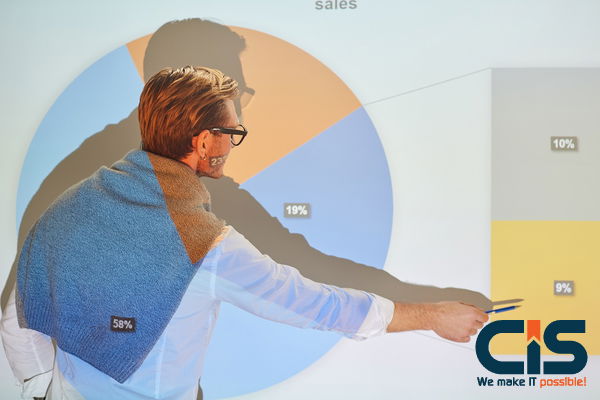
Large neural networks are used in deep learning, a technique for machine learning, to discover the best way to carry out a task.
Another kind of machine learning is reinforcement learning. It enables robots to determine how to maximize particular results, like profit or engagement. Machines can experiment with various activities to observe how they affect the result.
Let's examine these methods and discuss their significance.
Deep Learning
You are familiar with deep learning, a subset of machine learning that uses artificial neural networks. Layers of nodes that can be connected and can spot patterns make up these networks. Deep learning networks can recognize complex patterns in training data (text, images, and sound).
Finance
Deep learning is employed in the financial sector to forecast stock values, identify fraudulent transactions, develop trading techniques, and enhance decision-making in various contexts.
Deep learning is used in the promising application of sentiment analysis to find positive and negative attitudes in text data. This can be applied to forecast stock prices or make investment decisions.
Machine learning is used by a renowned investment firm that has unprecedented annual returns north of 70%, to enhance its capacity to forecast stock movement.
Machine learning is undoubtedly an important factor in the Fund's success, which is referred to as a "$74 Billion money-making machine unlike any other." Given their strong profits, it is not unexpected that other investment companies desire to adapt methodology.
This is the only business, though, that has had relative success. That has more access to data and more sophisticated computers than any other corporation, besides having world-class data scientists on staff. They have access to machine learning in a manner that other businesses do not.
Healthcare
Numerous aspects of healthcare, such as diagnosis, treatment planning, and patient monitoring, can benefit from deep learning. One of the most potential applications of deep learning in healthcare is cancer treatment and diagnosis.
Researchers are employing deep learning algorithms to identify trends in cancer data. This can be useful for prognosis, treatment planning, and diagnostics. In one study, deep learning was used to create a model that, with 99 percent accuracy, could distinguish between several forms of breast cancer. Another group also used deep learning to develop models that could forecast how long cancer patients would live.
Algorithms for deep learning can also help patients. They can monitor the patient's vital signs and let them know if something is amiss that aids in doctors' early detection of issues.
Drug discovery can also be enhanced with the help of deep learning systems. A deep learning algorithm was employed in one study to find new medications.
Traditional drug discovery is expensive, time-consuming, and frequently yields unsatisfactory outcomes. Deep learning promises to expedite and enhance process accuracy. Real-world repercussions could include patient access to potentially life-saving medications being sped up.
Deep learning in drug development has enormous potential, particularly when novel medicines are required and healthcare expenses rise.
Marketing
Deep learning can be applied to consumer segmentation, prospect identification, and the creation of predictive models that assist companies in anticipating the demands of their clients. Marketing automation platforms can also employ deep learning to improve the precision of their forecasts.
Subscription services are always seeking new strategies to keep customers. Deep learning could be used to prior customer behavior to ascertain which consumers are most likely to abandon their subscriptions. These data might be utilized to prevent customers from canceling to produce customized marketing communications.
Customer lifetime value can also be predicted with the aid of deep learning. These models can prioritize prospects, and marketing budgets can be optimized.
Marketing teams are already utilizing deep learning to enhance internet advertising. They can forecast which ads will convert the most effectively using clickstream data. Based on past behavior, deep learning can target particular clients with advertisements.
Deep learning is an effective marketing technique. With the correct data and tools, businesses can use deep learning to create unique marketing campaigns, generate revenue, and anticipate client demands.
Reinforcement Learning
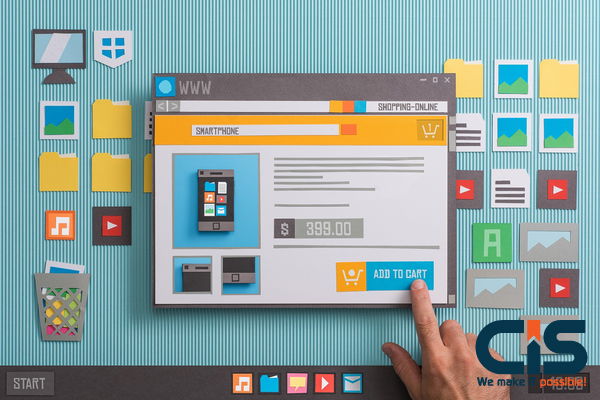
Reinforcement learning is a useful tool for businesses for several reasons. It can save time and money by automating repetitive operations. Additionally, it can aid in process optimization and detecting significant commercial prospects. Additionally, it can offer insightful information and useful facts to enhance decision-making.
Champion recently employed reinforcement learning to create a more effective boat with ideal hydrofoils, which helped it defeat its rivals.
Reinforcement learning is used to enhance data center cooling algorithms. Reinforcement learning was used by one company to cut the energy consumption of its data center by 15%.
Firms are being pressured to find ways to decrease their carbon footprint, as irreversible climate change is becoming a more pressing global problem. This goal can be achieved by using reinforcement learning.
It is also used in many other industries, such as:
-
Recommend products when preferences and behaviors change rapidly
-
Forecasting under dynamic conditions
-
Complex, dynamic logistic problems solved
Reinforcement learning is often used in the advertising industry to improve campaigns. They might examine previous customer behavior to determine which advertisements are most likely to convert clients. After that, they refine and improve the adverts using reinforcement learning. They can maximize the return on their advertising investment in this way.
Reinforcement learning is also useful in the field of finance. It has developed a reinforcement learning technique to aid stock pickers in improving their decisions. This program looks for patterns by analyzing many data points, such as previous stock prices, earnings, and analyst ratings. Investors may gain knowledge from it that will enable them to choose equities they should buy or sell more wisely.
Deep learning is based on real-world data. Reinforcement learning relies on synthetic data. Agents interact with the environment and receive feedback based on their actions.
Difference Between Deep Learning And Reinforcement Learning
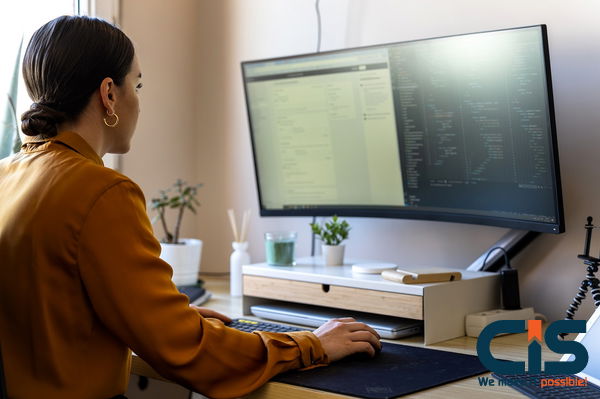
Deep learning and reinforcement learning are the two systems that learn. The difference between these is that deep learning is learning from a training group and then applying that learning to a new data place. In contrast, reinforcement learning is dynamic learning by adjusting activities based on constant feedback to optimize a reward.
Deep learning and reinforcement learning aren't mutually exclusive. You might use deep learning from a reinforcement learning approach known as deep reinforcement learning.
Takeaways
Reinforcement learning and deep learning are two of the most popular varieties of Artificial Intelligence. Computers can learn from vast volumes of data thanks to deep learning. Machines can learn how to behave in a given environment to maximize reward via a type of learning called reinforcement.
Deep learning and reinforcement learning each has advantages and disadvantages. While reinforcement learning can assist you in choosing the most effective path to reach a goal, deep learning can identify patterns in data. Deep learning systems might be more complex and challenging to implement. Reinforcement learning is less complicated but less successful at identifying patterns.
No code AI can be used to implement these systems and other kinds of AI. Without programming knowledge, users of this platform can develop and apply machine learning models. It makes AI accessible to everyone, regardless of programming ability or expertise. To get started, create a free account right away.