
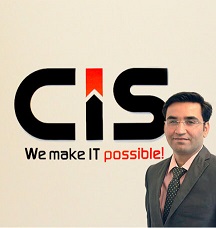
Contact us anytime to know more — Abhishek P., Founder & CFO CISIN
Big data analytics have emerged as an indispensable force in an information society where knowledge is highly prized, providing businesses with tools for success in an ever-evolving technology environment.
Innovations enable businesses to exploit the available information on hand fully; businesses are discovering how much their decisions based on data-driven decision-making pay off in this dynamic business climate.
Thanks to innovative algorithms and inventive methodologies like these helping firms realize incredible successes.
In this blog, we delve into the realm of big data analytics, exploring its benefits, trends, and much more. Keep reading to gain valuable insights.
Big Data Analytics
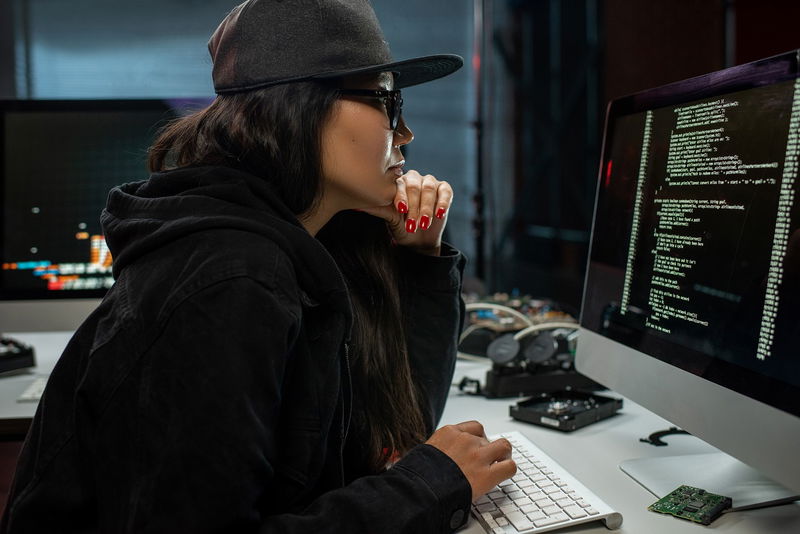
What is Big Data Analytics? Big Data Analytics refers to the practice of examining large volumes of various data sets with cutting-edge analytical methodologies from various sources and sources, ranging in sizes from terabytes to petabytes, which contain structured, semi-structured and unstructured information that must be managed for processing; such datasets constitute huge data.
"Big data'' refers to datasets that exceed conventional rotating databases' capacity of capture management processing - big data processing requires databases with reduced latency, so standard databases will not suffice as big data processing requires.
It defines big data analytics as providing researchers, analysts, and business users the means to take advantage of large amounts of previously unavailable and unusable information to make faster, better-informed decision-making processes.
Businesses use analytics technology to sift through mountains of unstructured and raw data for insights like hidden patterns, relationships that might otherwise go undetected, market trends or customer preferences which will allow them to better serve customers and generate profits.
Big Data Analytics: Four Types
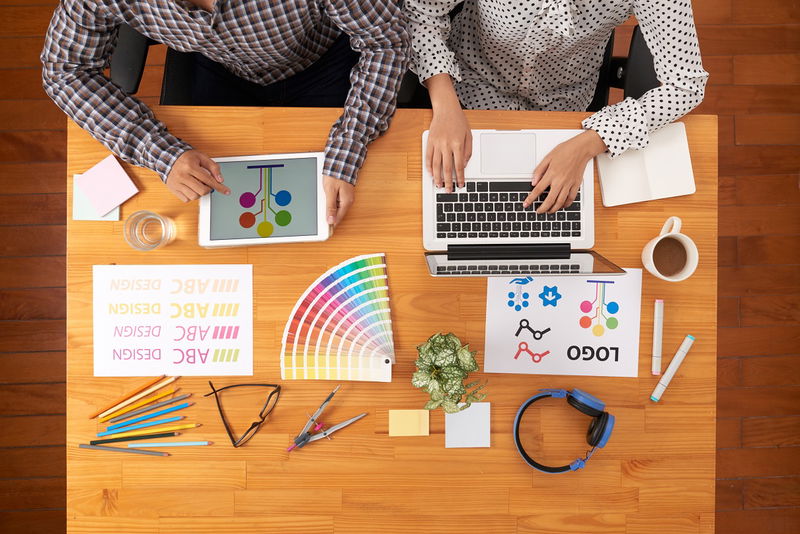
Different strategies are needed for the various types of data. Here we have outlined the important types of Big data analytics:
Descriptive Analytics
One method believed to assist businesses in recognizing trends within a client category is descriptive analytics.
By condensing and organizing historical data in an understandable format, one can gain greater insight into past events and trends while creating reports detailing sales figures, earnings figures and other key performance metrics of an enterprise.
Descriptive analytics includes using association rules in market basket analysis, clustering and summary statistics as examples of descriptive analytics applications.
A Company serves as an excellent example of its use; by employing this practice they increased office and lab space use by using historical data analytics.
Diagnostic Analytics
Diagnostic analytics provide an in-depth examination of an issue or problem and its underlying source, helping data scientists pinpoint its origin.
When searching for events with similar causes, diagnostic analytics methods include drill-down analysis, data mining recovery techniques such as Deep Packet Inspection or CROs as well as customer health score analyses to pinpoint causes.
Diagnostic analytics can be invaluable when used within the context of a company, when investigating factors that contribute to customer churn and usage patterns among your most loyal followers.
An e-commerce business serves as a good use case of diagnostic analytics; when customers add items to their carts sales have gone down significantly.
Diagnostic analytics helps businesses pinpoint the precise cause of any problem they experience and take measures to address them effectively.
For instance, incorrect form loading, expensive delivery costs or limited payment options might all contribute. With their aid, businesses are better able to understand why and address it accordingly.
Predictive Analytics
As its name implies, predictive analytics aims at forecasting future occurrences such as consumer and market trends or events related to markets in general.
Predictive analytics utilizes both historical and present-day data sets in order to predict these future happenings - this type of analytics is being widely implemented within organizations today.
Predictive analytics not only benefits service providers but customers as well. By tracking our actions in the past and making predictions based on them about future ones.
It looks at existing data to project future events using methods like data mining, artificial intelligence and machine learning techniques.
Churn risk, renewal risk analysis and next-best offers are just three examples of predictive analytics used by organizations today.
One such organization using predictive analytics effectively is PayPal (Stripe vs. PayPal), where predictive analysis helps the business decide the necessary actions to prevent fraudulent transactions by its clients and predict them using both historical payment data as well as user behavior data to predict fraudulent activities that arise among its client base.
Prescriptive Analytics
Prescriptive analysis is one of the most useful yet underapplied forms of analytics. As its successor to predictive analysis, prescriptive analysis investigates various options and makes recommendations based on descriptive and predictive analytics performed on specific datasets.
Prescriptive analytics utilizes various business rules and data sets. Prescriptive analytics uses information sourced both externally (such as social media insights) as well as internally (organizational inputs).
Prescriptive analytics helps companies pinpoint the most efficient solution to any given problem or challenge while predictive analytics offers control over future events to mitigate any associated risk.
Prescriptive analytics has proven its use for client retention by employing analyses like next-best action and next-best offer analyses, among other examples.
A Health Care system found prescriptive analytics useful by decreasing readmission rates by 10% and saving over $6 Million; prescriptive analytics can also assist clinical trial patient selection processes such as drug development as well as improving other related processes in healthcare sector settings such as patient selection for clinical trials or drug development programs.
The Operation Of Big Data Analytics
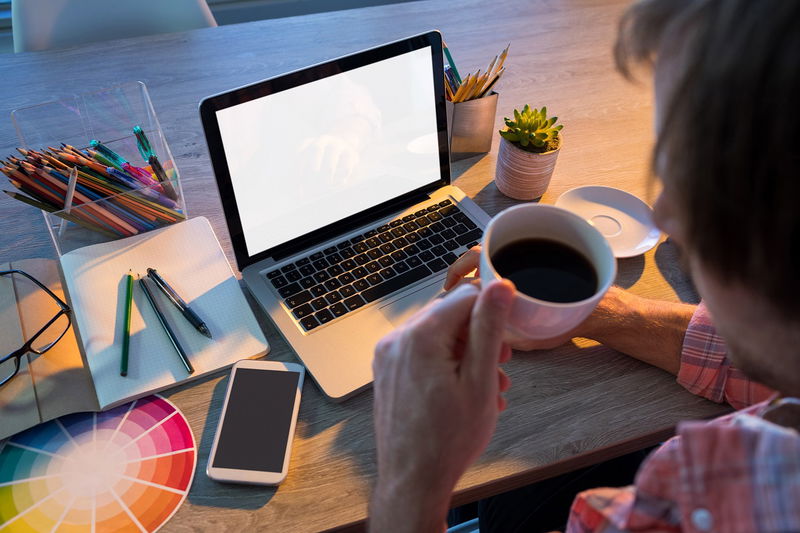
Big data analytics entails gathering, processing, cleaning and analyzing large datasets as part of its process - helping businesses operationalize their big data resources more easily.
-
Collect Data: Companies vary considerably when it comes to how they collect data.
Organizations now collect structured and unstructured information from a range of sources - mobile apps, cloud storage services, IoT sensors installed in stores - while some data may also be kept in data warehouses with easier access by business intelligence tools and applications for later retrieval or stored as unstructured in a data lake with its metadata assigned accordingly.
-
Process Data: In order to produce reliable analytical results from collected and stored data, it should be organized accordingly after collection and storage, particularly if it's vast and unstructured.
Organizations have difficulty digesting this exponentially growing pool of available information; batch processing provides one approach by gradually processing large data blocks until analysis can take place at length; stream processing reduces this turnaround time so decisions can be made more rapidly by looking at small batches all at once - though stream processing tends to be more expensive and complex than its batch counterpart.
-
Clean Data: For maximum data quality and robust results, all data, regardless of size, must be scrubbed to reduce duplicated or irrelevant entries and account for duplicate or misleading ones that might create unreliable insights.
Duplicates or irrelevant items need to be deleted.
At the same time, obscure or misleading records must also be eliminated or properly documented in order to improve data quality and deliver more robust results.
- Analyzing Data: Transforming vast amounts of information into meaningful form requires time and dedication, yet once ready, it may provide invaluable insights through modern analytical procedures.
-
Data Mining: It employs anomaly detection and clustering techniques to search through massive databases for patterns or links that appear.
By drawing upon previous company data for use as forecasting indicators, predictive analytics forecast the future with confidence while simultaneously spotting threats or opportunities that lie within its parameters.
- Deep Learning: It emulates the human learning tendencies by employing algorithms and AI technologies such as machine learning to identify patterns even in data that appears seemingly random or complex, creating layers upon layers of algorithms which identify and uncover them systematically and slowly over time.
Benefits Of Big Data Analytics
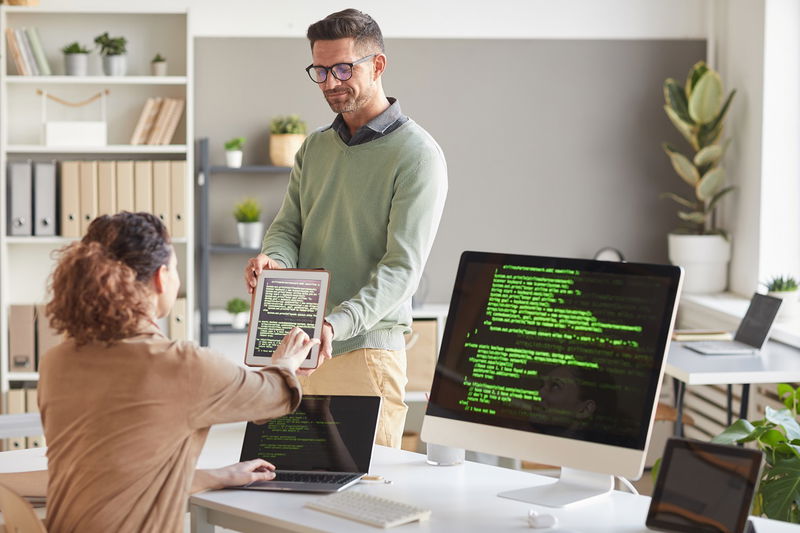
Here we have outlined the benefits of big data analytics:
-
Making Informed Decisions: One of the primary advantages of big data analytics lies in its ability to facilitate informed decisions.
Data-driven insights allow organizations to make better strategic and well-informed choices - particularly helpful when experience and intuition may not provide as reliable results.
-
Enhance Customer Understanding: Businesses can gain more insights about their customers using big data analytics.
Businesses could improve the consumer experience overall, provide personalized product suggestions or develop more targeted marketing campaigns by evaluating customer data - leading to enhanced client happiness and loyalty as a result of such analytics.
-
Increased Operational Efficiency: One key benefit of big data analytics is an increase in operational efficiency.
Through real-time identification of inefficiencies and bottlenecks, organizations may optimize their processes - leading to both cost savings and productivity gains.
-
Competition Advantage: Leveraging big data effectively can give your organization an important competitive edge in today's environment.
Companies who utilize big data analytics well are better able to take advantage of new possibilities, adapt quickly to changing market conditions, and outstrip rival businesses in terms of innovation and productivity.
-
Predictive Analytics: By employing predictive modeling enabled by big data analytics, organizations are able to project future patterns and events more precisely.
This approach has proven particularly advantageous in fields like banking where forecasting market trends could yield substantial returns - such as forecasting market fluctuations.
-
Innovation: It can be encouraged with big data analytics.
Organizations can develop new products and services by using data analysis to gain an insight into client demands as well as trends.
- Healthcare Advancements: Big data analytics has the power to transform patient care in healthcare institutions worldwide, potentially saving lives through early disease detection, therapy optimization and drug discovery.
Read More: Big Data Essentials: Things You Need To Know For 30% Gain
The Top Six Newest Trends In Big Data Analytics
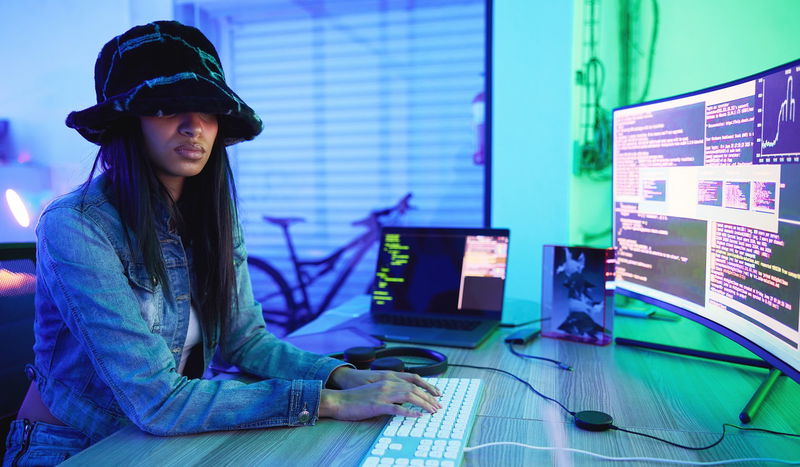
Here we have outlined the important trends in big data analytics:
Natural Language Processing
Natural Language Processing (NLP), an AI technique that allows computers to understand spoken language, allows organizations to gain valuable insights from unstructured data sources like customer reviews and social media posts by employing natural Language Processing technologies such as NLP.
Organizations using these technologies can then extract useful insights by extracting useful textual data for customer assistance processes as well as gain a better grasp on client emotions, using its power for decision-making on data-driven decisions while improving consumer experiences through thorough language processing analysis - revolutionizing their approach towards language analysis!
Real-Time And Streaming Analytics
As business operations increasingly digitize, streaming analytics are an emerging trend within big data analysis.
Organizations who can instantly access an abundance of data can make faster, informed decisions quickly by using streaming analytics to track data as it comes in and identify patterns or abnormalities and react more rapidly when new developments emerge within big data analysis. Streaming analytics solutions empower businesses with streaming analytics capabilities to unlock insightful information for improved operational effectiveness and offer unparalleled customer experiences.
Artificial Intelligence & Machine Learning
Artificial intelligence (AI) and machine learning (ML) technologies represent two significant advances in big data analytics.
AI algorithms facilitate efficient handling and examination of large datasets, spot trends and develop precise forecasts; additionally, they automate decision-making processes so companies can find insightful information to promote expansion and boost productivity.
Businesses can leverage AI and ML technologies to extract key insights from their data, equipping them to make strategic and well-informed decisions that drive success.
AI/ML has revolutionized data analysis. Businesses are taking advantage of this revolutionary tool for competitive advantages, operational management efficiencies, customer experience enhancement and automated decision-making processes to gain a competitive edge and enhance customer experiences by automating decision processes with AI/ML systems.
Edge Computing
Edge computing is an emerging trend in big data analytics that makes it possible to analyze information locally on servers, user devices and Internet of Things devices.
Edge computing does this by moving computing closer to the edge of the network - thus eliminating long-distance connections between clients and servers and eliminating latency-free real-time processing as it permits prompt device responses.
Edge Computing's key advantage lies in its effective use of bandwidth, which enables processing large volumes of data with minimal network resource consumption and thus reduced operational costs for businesses while improving data streaming capabilities.
Furthermore, edge computing's software installation/operation in remote areas reduces operational costs while increasing the flexibility of operations; due to these advantages, edge computing has revolutionized the Big Data analytics space by improving processing abilities without being limited by latency or distance constraints.
Hybrid Cloud Computing
Hybrid cloud computing has quickly become one of the newest and most sought-after big data analytics trends, providing flexibility and optimal data deployment by combining private and public cloud environments.
Organizations typically need an infrastructure consisting of servers, storage devices, local area networks (LAN) connections and load balancers in order to implement hybrid cloud architecture while virtual machine (VM) and container support also requires installing a hypervisor or virtualization layer for proper functioning of hybrid architectures.
Companies must install a private cloud software layer in order to safeguard data transfers between private and public clouds, using hybrid cloud strategies as the optimal way of data deployment.
Organizations can enhance data deployment using such strategies while keeping sensitive information within private clouds and taking advantage of public ones' scalability and affordability. Such strategies provide enterprises with the agility and flexibility necessary for cloud computing requirements.
DataOps' Rise
In today's data-rich environment, DataOps is becoming an indispensable technique that assists firms in managing their data efficiently and making the most out of investments by structuring massive quantities of available information into usable form.
Software as a Service (SaaS) further emphasizes this technique's significance within modern company operations.
Data, analytics and artificial intelligence/machine learning (AI/ML) technologies have rapidly expanded over time and become a central aspect of daily company operations, necessitating data operations (Ops).
Management must now address different aspects of data storage affecting usability and quality. In many ways, this shared responsibility affects all areas within an organization's work processes, and data quality can only improve.
Want More Information About Our Services? Talk to Our Consultants!
Conclusion
Cisin offers unparalleled Big Data Analytics Services to companies of any kind.
Recognized for our vast knowledge and experience in Big Data analysis, Cisin stands out as a premier consulting business offering tailored services from advanced analytics visualization data gathering processing for your unique requirements. Working with Cisin means taking advantage of all that your data offers while creating lasting outcomes in big data analytics.