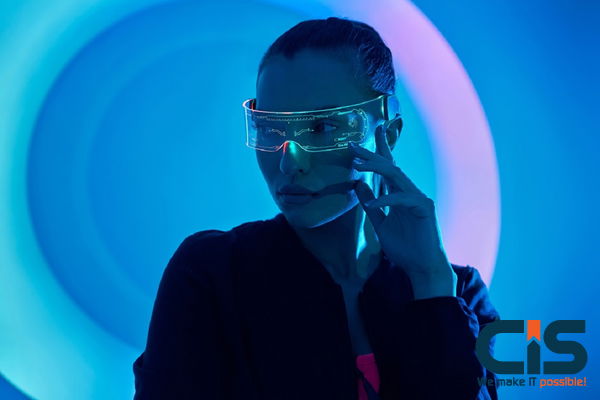
Data mining first debuted in business analytics during the 1930s. However, its widespread implementation didn't gain popularity until the 1990s, when businesses tried to make sense of massive amounts of information generated degree programs.
Data mining allows businesses to gain valuable insights, predict future outcomes, mitigate risks, and uncover new opportunities. In this article, we'll explain data mining and its relevance in business analytics.
What Is Data Mining?
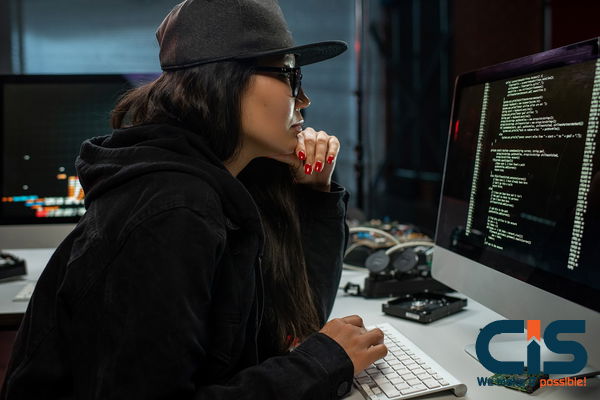
Data mining is turning raw data into actionable intelligence for businesses. Utilizing programming languages like R and Python, data miners use this process to discover patterns in their data and gain more insight into their organization's customers, products, processes, and operations. All organizations should employ data mining; it enables businesses to make smarter decisions by comparing sets of information. Data mining allows companies to develop strategies, increase sales volumes, and market more effectively while helping create plans.
Data mining can easily be confused with data analysis or machine learning, yet the two processes are fundamentally distinct.
Both data mining and machine learning utilize analytics and patterns. Still, data mining primarily operates already present practices, while machine learning uses that same data to predict future outcomes. Data mining's "rules," on the other hand, are unknown at the start, whereas machine learning involves giving variables or rules to help it understand data better; data mining relies heavily on decisions by human intervention, while machine learning can learn itself independently; both processes often overlap as machine original reporting learning can be utilized within data mining to automate processes.
Data mining and Analysis should not be confused; However, data analytics often uses mining and should not be seen as interchangeable terms. Analytics involves diving deeper into information to gain more knowledge; data analysis includes inspecting, cleaning, and transforming this information before providing insight that allows forming conclusions and taking actions based on it.
Overview Of The Data Mining Process
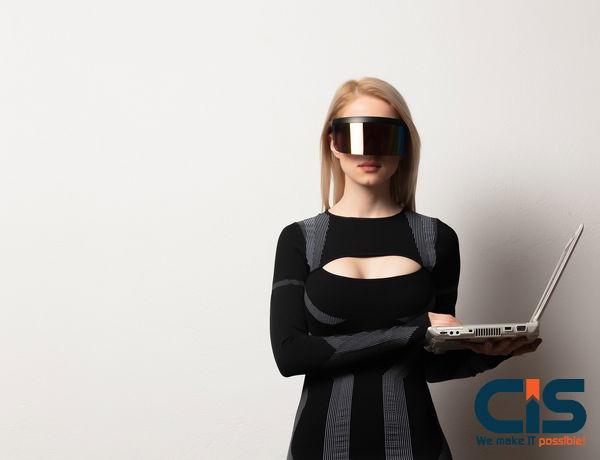
Almost all businesses use data mining. Knowing how data mining works and what it can do for a company is essential.
Business Understanding
To be successful at data mining, it is first necessary to understand your business goals and translate these into an action plan and problem for data mining. Without knowing the overall goal of your business's data mining activities - for example, a supermarket may use data mining to find out more about its customers, such as what items they buy most frequently - creating successful business decisions algorithms will become impossible.
Data Understanding
Once you've established your business's requirements, the next step should be collecting data. Data can be collected, organized, managed, and stored using any number of methods; data mining involves familiarizing yourself with this data - familiarizing yourself with issues, gaining insights, or observing subsets - for instance, a supermarket might use rewards programs that allow customers to enter their phone numbers when making purchases allowing additional cost them access to this valuable source of data.
Data Preparation
Preparing data is part of preparation. Data mining specializes in this task by translating computer-language data into something people can easily comprehend and quantify, thus making this step essential in modeling. Transforming and cleaning up data before use are other crucial steps involved here.
Modeling
Mathematical models are utilized during the modeling phase to detect patterns within data. Various techniques may be employed when dealing with one set of information; modeling often takes multiple attempts before reaching its desired career path goals.
Evaluation
At this stage, the model must be carefully evaluated, including all steps taken during its creation, to ensure it fulfills business objectives. A decision will then be taken regarding data mining outcomes; for example, in a supermarket situation, data mining would yield a list of customer items - precisely what the business exciting career desired.
Deployment
Data mining can be straightforward or intricate depending on its desired end goal, from creating a regular automated data mining process to more complicated endeavors like simulating real-life weather events.
Outlier Detection
Data mining is an analytical technique that detects anomalies within patterns found in datasets. Outlier detection is essential for maintaining secure databases; businesses often rely on it to detect fraud or suspicious account activity that might indicate theft, as it highlights any outlier data points that differ significantly from the rest of the information set and could indicate theft or fraud. Understanding anomalies within production or distribution lines could also help pinpoint advanced degree bottlenecks or blockers.
Classification
Companies of all industries can utilize data mining techniques like classification. While it could be considered a form of clustering, its primary use lies in comparative analyses and categorizing large groups based on demographic or other criteria - an invaluable way for businesses to gain comprehensive insights from big data. Financial institutions frequently employ classification for financial data mining purposes by categorizing consumers based on variables to project business leaders credit card risk or offer new loans.
Data Visualization
Business stakeholders that aren't data analysts or engineers can quickly grasp data by visualizing it using charts, graphs, and diagrams. Businesses widely utilize data visualization as it effectively conveys the results of data mining activities to decision-makers allowing companies to make better decisions with a clear presentation of their information.
Data Cleanup
Data cleaning is the practice of preparing data for mining, consisting of removing duplicate and corrupted records and filing any null values with correct values. Once clean data has been processed for mining analysis purposes, incorrect descriptions defeat the purpose of analytics, harming businesses regardless of their career option sophistication.
Association
Data mining is discovering relationships among variables in a database and finding correlations or associations among data points. This process identifies unique relationships among variables within it. Primarily used for marketing strategy decisions, governments frequently employ associative techniques when planning public services or doctors diagnosing medical conditions more accurately.
Want More Information About Our Services? Talk to Our Consultants!
What Is Data Mining, And How Does It Inform Business Analytics?
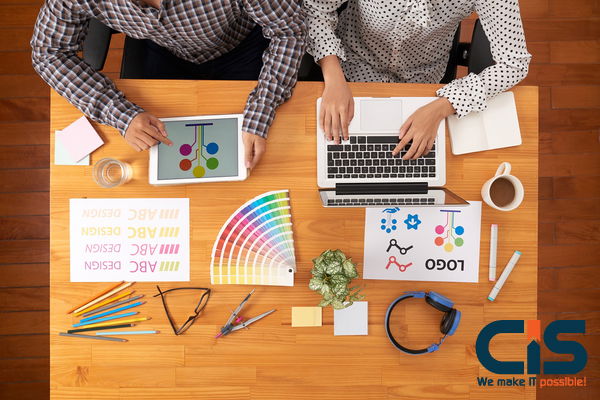
Why is Data Mining Crucial for Businesses? Businesses that employ data mining can gain a competitive edge, achieve greater customer insight, streamline operations, and acquire customers faster - creating new opportunities in various industries. Data analytics offers various advantages across industries; some seek ways to attract new customers, others look for marketing strategies, and others look to improve systems. Data Mining allows businesses to analyze information and make decisions more quickly and accurately about mining equipment.
Clustering
Clustering groups data points according to similar characteristics, then breaks them into subsets for informed decision-making. Business analysts gain access to information regarding broad demographics as well as behavior. Retailers use clustering as a way of categorizing customers based on products purchased so that ads targeted towards each cluster are seen by customers within each one.
Data Mining Techniques In Business Analytics
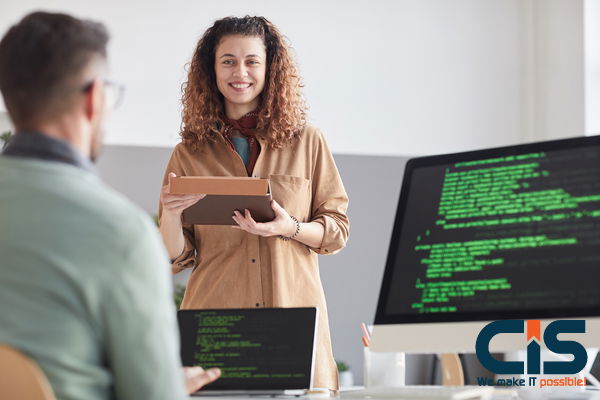
After you've learned why data mining matters, it is beneficial to understand how data mining functions in business settings.
- Classification: Classification is a more advanced data mining technique that utilizes attributes to group information into categories and draws inferences from them. Supermarket data mining employs this strategy by categorizing grocery items customers purchase, such as meat, produce, and bakery goods - providing more insight into customer habits, outputs, and outputs.
- Clustering: Clustering is similar to classification in that it chunks data based on similarities. Cluster groups tend to be more adaptable and flexible than classification ones and thus easier to use when data mining - in a supermarket scenario, for example, they could contain food and non-food items without needing specific classes as the basis.
- Association Rules: In data mining, association involves tracking patterns based on linked variables. For example, customers who purchase one item are likely to buy others. Stores might group food products, or online shopping sites might display "people also purchased this" sections for customers' convenience.
- Analysis of Regression: Regression can be used to model and plan by identifying the probability of particular variables. A supermarket can project price points by analyzing available products, consumer demand, competition, and more - data mining becomes more accessible alongside regression, which helps identify relationships among variables.
- Anomalous/outlier Detection: Observing overall patterns may not be enough to achieve maximum benefit from data mining; data must also identify and understand outliers. For instance, if most shoppers at a supermarket tend to be female, but one week in February sees male shoppers taking precedence, you should explore this outlier to understand its cause.
Data Mining Techniques
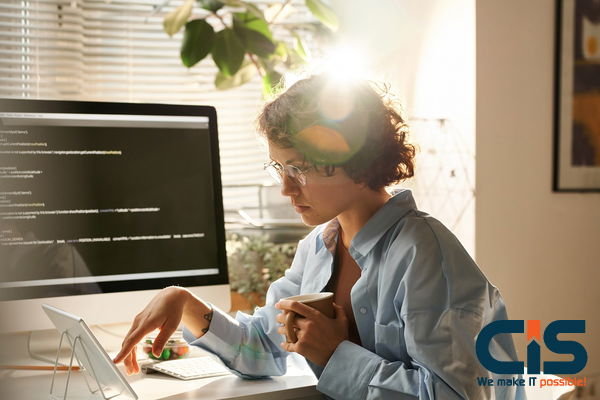
Data mining is a technique that uses algorithms and other methods to transform extensive data collections into helpful output. Data mining methods can be categorized as follows:
- Association Rules (also called Market Basket Analysis) is a search for relationships among variables. By connecting data sets and building on this relationship between variables, Association Rules create value within them. For instance, association rules could analyze sales records to see which products were purchased most frequently together so retailers can plan, market, and forecast more efficiently.
- Classification assigns objects to predefined classes representing common characteristics between data points or describing items. This data mining technique allows data to be neatly summarized and organized across similar features or products.
- Classification and clustering are synonymous processes; clustering identifies similar objects and groups them based on their similarities. While classification might produce categories such as "shampoo," conditioner," soap," and "toothpaste," clustering could instead produce groups like "hair care" and "dental hygiene."
- Decision trees can help predict or classify outcomes based on the criteria listed. A decision tree gathers input by asking cascading questions, which sort responses according to responses provided by its user base. It creates a visual tree that includes feedback and allows deeper investigation of data sets.
- K Nearest Neighbor (KNN) is an algorithm for classifying data based on its proximity to other data sets. KNN operates under the assumption that closer items will tend to share similarities; therefore, this non-parametric supervised technique predicts group characteristics using individual data points.
- Neural networks use nodes connected by inputs and weights to process data, much like neurons in our bodies do. Data is then mapped using supervised learning - similar to how your brain connects - while threshold values can be set to determine this model's accuracy.
- Predictive Analysis uses historical data to create visual or numerical models that predict future outcomes, similar to regression analyses, to give insight into an unknown factor based on current evidence.
The Data Mining Process
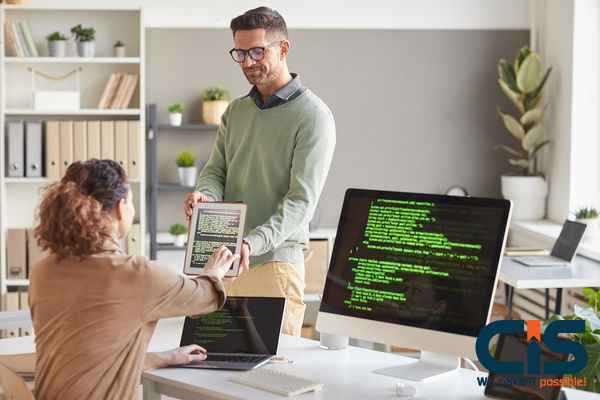
Data analysts can be most efficient when they adhere to a specific sequence of tasks during data mining. Otherwise, they risk running into mid-analysis issues that would have been easily preventable if they had prepared beforehand. Data mining typically comprises three steps.
Step 1: Understand the Business
Before touching or extracting data from its source, we must deeply understand who lies behind it. What are their goals in mining data mining; What is their business; What result of an S&T analysis will occur at its conclusion? Successful mining starts by defining success at its endpoint.
Step 2: Understand the Data
Once the problem is clearly defined, it's time to focus on data. It is crucial to assess all available sources, what will occur with them, and the best ways of collecting and storing information. Furthermore, you will want to determine any limitations or restrictions to data mining that could impede its progress.
Step 3: Prepare the Data
Data is collected, downloaded, extracted, or calculated before being cleaned, standardized, checked for errors, assessed, and limited to identify outliers. They should also be checked for size at this stage, as too much data could slow down computations and analyses.
Step 4: Build the Model
Now it's time to crunch some numbers! Data scientists employ various forms of data mining to search patterns, trends, and associations within historical information. Furthermore, their analyses may also serve as input into predictive models, which allow them to predict how this might translate into future outcomes.
Step 5: Evaluation of Results
Data mining's data-centric component culminates in evaluating its results, such as models created. Once this step is completed, the analysis results can be aggregated, interpreted, and presented to decision-makers who have been mainly left out of the process up until now. Findings may also inform organizational decisions.
Step 6: Change the and Monitor it
After data mining is completed, management will take action based on its findings. They could decide that there wasn't enough data, the results weren't relevant, or shift their strategy in response. Either way, management evaluates its impact on future activities. It creates new data mining loops to identify organizational problems or opportunities.
Read More: Utilize Analytics And Data Mining Technologies To Drive Business Decisions
Data Mining Applications
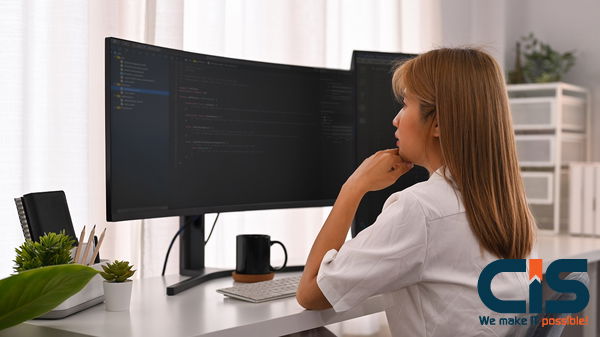
Data mining applications are an indispensable asset that can benefit almost every department, company, sector, and industry in today's information age.
Sales
Data mining can help businesses increase revenues through more intelligent and effective use of capital. Consider your local coffeehouse's point-of-sale system. Each sale records the date and time and what products were purchased, allowing the shop to use this data to design its product range strategically.
Marketing
Once a coffeehouse has determined its ideal menu, the next step should be making necessary changes. To maximize its marketing efforts and increase success, the store could utilize data mining to gain insight into customer interactions with ads, the best demographics to target, digital ad placement methods, and to align campaigns with promotional offers, cross-sell offers, cross-sell programs, etc.
Manufacturing
Data mining is an essential asset to companies that manufacture their products, helping to analyze how much raw materials cost, which materials are utilized most efficiently during production, how long manufacturing takes, and any bottlenecks or delays that might impact it negatively - ultimately helping ensure uninterrupted deliveries of goods.
Fraud Detection
Data mining involves uncovering correlations, patterns, and trends within data points to find correlations and outliers that shouldn't exist - for instance, a company might use data mining to detect unexpected cash transactions into unanticipated accounts - this process may then help uncover correlations that were otherwise impossible to notice before. A firm might use Cash Flow analysis to discover transactions made out of sequence - for instance, an unknown account might show up on Cash Flow analysis as regular payments without explanation, or an investigation into unexpected reports may occur unexpectedly.
Human Resources
Human Resources departments possess numerous data sets that can be processed. These include retention rates, promotions, and salary ranges of employees. Furthermore, Human Resources also contain data regarding employee benefits usage and employee satisfaction surveys that can be utilized to understand better why employees leave and what attracts new hires. All this data can be combined for Analysis to gain a deeper insight into why employees leave and attract new ones.
Customer Services
Customer satisfaction can be affected (or destroyed) by various factors. Consider working for a shipping company; customers might not be pleased with shipping time, quality of shipping, or communication issues; they might be frustrated with long phone waits or slow email responses. Data mining efficiently gathers operational information on customer interactions. It summarizes them to highlight weak spots while emphasizing what the company excels at doing well.
Data Mining Benefits
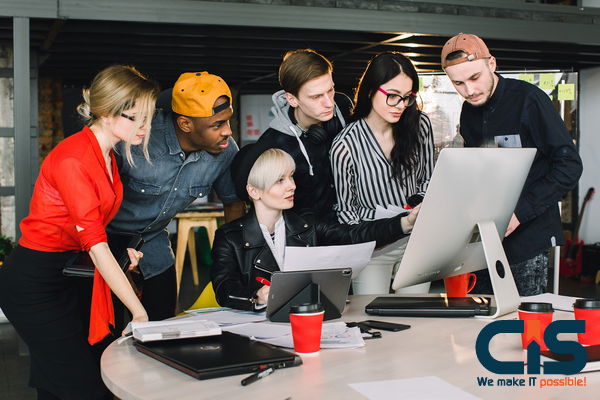
Data mining is an effective strategy to ensure a company collects and analyzes reliable information. Data mining follows a structured procedure to identify an issue, gather the appropriate data, and seek solutions. Data mining can help companies become more efficient or profitable.
Data mining processes remain consistent across applications yet may take on different shapes depending on whether they are new or existing. Data mining provides a means to gather almost any type of information. Furthermore, it can help solve business issues that need quantifiable evidence, as evidenced through quantitative measurements.
Data mining serves to identify whether there is any correlation or cohesion among raw data sets, giving companies the chance to use information they otherwise wouldn't.
Limitations In Data Mining
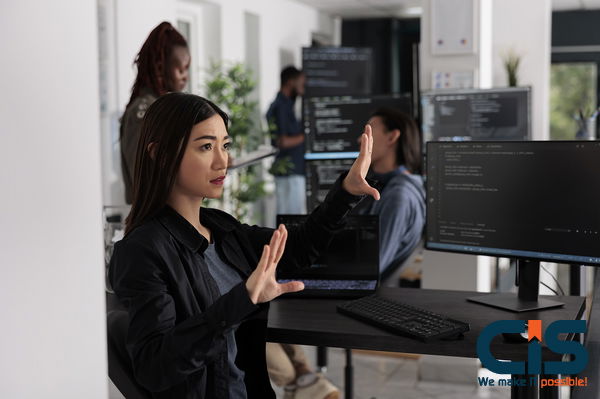
Data mining's complexity is its main drawback; data analytics requires extensive technical skills and software tools that may be too much of a barrier to entry for smaller companies.
Data mining does not always bring positive outcomes. Companies can utilize it for statistical analyses, concluding strong data sets, and making changes without experiencing tangible benefits from data mining. Furthermore, data mining should never be seen as a guarantee of success. Instead, it can lead to inaccurate conclusions, market shifts, or model errors that compromise success altogether.
Data mining comes at a price, too. Some tools require costly subscription fees, while obtaining certain information may prove challenging. Although additional IT infrastructure may be expensive, different IT security solutions can alleviate privacy and security concerns. Data mining works best with large data sets which must be stored.
Data Mining and Social Media
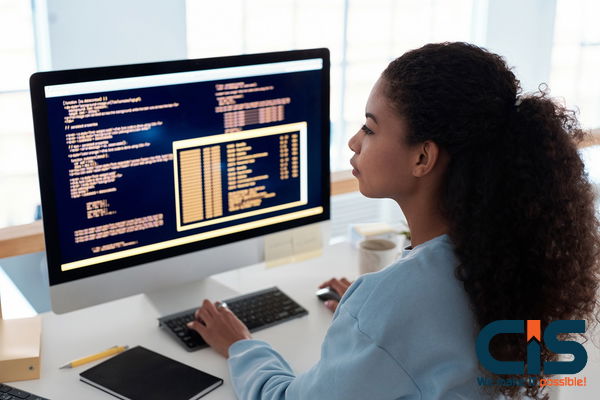
Social media companies have taken to data mining with great success. Platforms like Facebook, TikTok, and Instagram collect extensive user information through online activities like visiting web pages or posting comments.
This data can provide the basis for making inferences about individual preferences, enabling advertisers to target their messages at those who seem likely to respond positively.
Data mining on social media platforms has become increasingly contentious, as numerous investigations have demonstrated its invasive nature. Users may unwittingly agree to terms and conditions for sites without realizing that their information will be collected or sold.
Want More Information About Our Services? Talk to Our Consultants!
Conclusion
This article discusses various aspects of data analytics. We focused on its application within businesses, the benefits associated with business analytics data mining techniques, and free tools that may assist in harnessing all that business-generated information.
Business intelligence encompasses data mining, data analysis, and artificial intelligence processes, which assist companies and organizations with making better decisions, better understanding customers and their outcomes, and increasing service quality.