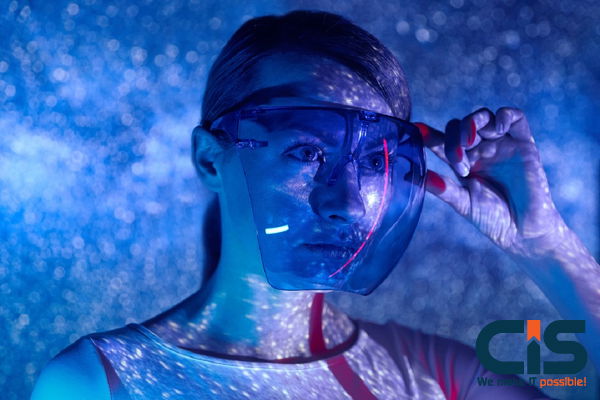
Companies lacking quality-assuring governments miss opportunities to use data while wasting precious resources. Processing and cleaning up can consume over 50% of an analytics team's time (even among highly paid data scientists! ). This makes scaling more challenging while frustrating employees; per the Global Data Transformation Survey, respondents reported spending 30% of total time doing tasks that do not add value due to poor quality or availability.
Data governance provides enormous value that's difficult to put a price tag on yet can still be measured indirectly. Leading firms have found it effective at eliminating millions of costs from their data ecosystems while creating digital and analytical use cases worth millions and even billions - evidence of its fundamental economic significance! Proper data governance distinguishes firms that capture value from those that fail. Investing enough money in administration reduces regulatory risks at your organization - an additional consideration.
It Is Essential To Build A Solid Foundation For Good Governance
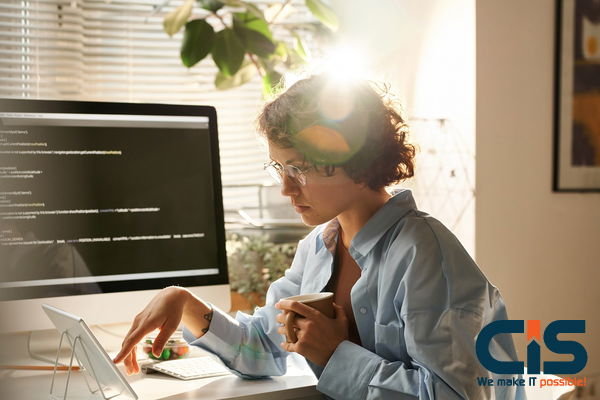
Some organizations have successfully scaled their data governance effectively, while others struggle. A leading retailer with IT managing its data governance was having difficulty extracting value from its information for several years before transitioning into enterprise analytics, so as part of a transformation program, it invested in training and engaging its entire executive leadership in data governance responsibilities; each executive leader was assigned various domains or subject areas related to consumer transactions or employee information that covered multiple functions or areas within business operations.
These leaders quickly saw the value of data governance once they understood its benefits, becoming champions for it themselves once they understood its necessity. When selecting domain owners and stewards within each domain, they directly connected data governance efforts with priority analytics use cases, working in sprints according to their ability to deliver value, checking in every couple weeks with the senior leadership team for progress review meetings with our senior leadership team based on delivery; ultimately this effort allowed priority domains to be created more rapidly than in years; reduced time that data scientists spent cleaning data faster speeding delivery of analytics use cases more quickly thereby growing this program over time.
Effective data governance demands organizational retooling. A typical governance structure typically comprises three elements.
- Central data management (DMO) is typically headed by a Chief Data Officer (CDO) and has a data strategy with governance leaders that set standards and the organization's direction.
- Governance roles are organized according to the data domains where day-to-day work occurs.
- A data council is a group of domain leaders, the DMO, and other stakeholders who work together to align the DMO's data priorities and strategy with the company strategy. They also approve funding and deal with issues.
The structure is the basis for data governance. It balances central oversight with proper prioritization and consistency while also ensuring the data management of the people who create and use the data (Exhibit 2)
Want More Information About Our Services? Talk to Our Consultants!
Data Governance Excellence: Six Ways To Achieve It
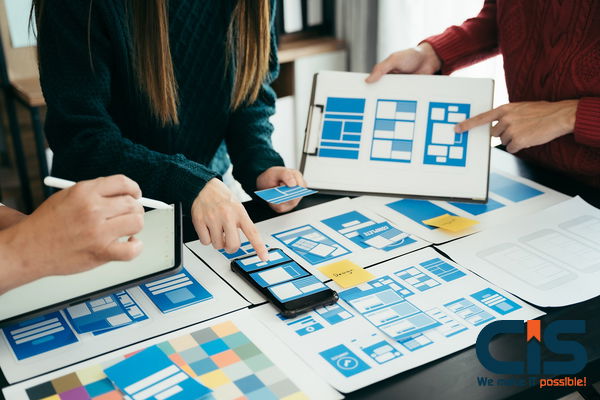
However, the foundation of an organization alone is not sufficient. Data governance solutions must be based on six critical practices.
Get The Attention Of Top Management
As shown above, success in data governance depends heavily on securing buy-in from business leaders. First, the DMO must consult with C-suite members to understand business requirements better, identify challenges facing data and the organization itself and understand all pitfalls with data. Once that process has taken place successfully, senior management (which may include C-suite leaders) is often convened into an advisory council of data governance which guides strategy towards meeting business requirements while overseeing and authorizing initiatives driving improvements such as designing enterprise data lakes or similar activities.
Once this step has been accomplished, the DMO should collaborate with Governance Council members to select business leaders of each data domain. They are accountable for the day-to-day governance of data by setting quality standards and outlining elements; time should also be devoted to familiarizing these new roles, which may have been added onto existing responsibilities of leaders; they must possess skills sufficient for successfully fulfilling those responsibilities.
Top-down buy-in from business leaders is necessary to prevent role clarification and empowerment issues from emerging. At the same time, data stewards will recognize this is a priority for their organization and set aside enough time and energy to address it (perhaps by changing performance metrics or adapting their other responsibilities). Furthermore, this approach allows immediate resolution of data ownership conflicts.
Leaders within their industries develop concrete ways of measuring progress and creating value. For instance, leaders may utilize time spent by data scientists searching for, curating, or creating necessary data to support meaningful use cases or quantify losses related to poor data quality or business mistakes as impact metrics for top management to remain attentive and ensure continued support of such issues.
Integrate Primary Transformation Themes
For practical governance efforts to generate value, ensure they connect directly to ongoing transformation efforts that already receive CEO attention, such as digitization, omnichannel enablement, or enterprise resource planning modernization - these typically depend on having sufficient data available and its quality.
Linking governance with transformation themes helps senior leaders be convinced more easily of organizational transformation initiatives, changing its structure more drastically. Instead of leaving the government to run itself, these initiatives transfer responsibility for data and governance directly onto product teams for seamless integration at production and consumption points.
European retailers embarked on a digital transformation of their core business and rapid online presence expansion, necessitating significant redesign of all e-commerce platform components, including the back end. A DMO and Data Council were established to assist with creating an ecosystem strategy and data domain structure along with goals related to data management.
Lead product owners were instrumental in leading several digital transformation squads within some functional regions and became data leaders within their scope of responsibilities. Data domains were assigned to product owners - for instance, those driving process improvements for in-store checkout may take on ownership for sales and payments data domains as part of their remit. Essentially, this structure ensured data governance met business requirements, with those creating and using it actively managing it themselves.
Data Leadership: Prioritize Assets, And Focus On Them Accordingly
Many organizations approach data governance from an organizational perspective, taking an all-inclusive approach. Such an expansive view may result in slow progress and risks not directly connecting to business requirements; prioritization should occur at two levels: domains and individual assets within each field to maximize success with data assets.
Data councils, with assistance from DMOs, must prioritize domains based on transformation initiatives, regulatory needs, and any other inputs to create a roadmap for domain deployment. The next step for organizations must be rapidly deploying priority domains - starting by quickly rolling out at least two or three before striving to operationalize each one within several months fully.
The North American retailer set itself an ambitious goal of using advanced analytics to transform its company in three years, realizing quickly its existing data was holding it back and setting up both Data Management Offices (DMO) and domains to increase governance. They prioritized deploying two environments; transactional (logging in-store purchase information) and product information (creating hierarchies of details and products). These processes helped expedite priority use cases related to inventory and assortment management.
Prioritize data assets across each domain by assigning each a criticality level (and associated care). Most organizations limit criticality levels (and related care) for purchases to 10-20 percent of criticality data assets, although for elements used less frequently for analytics, reporting, or business operations such as customer degree tracking, an ad hoc monitoring of quality without search is sufficient; this significantly lowers governance efforts while keeping focus on relevant information.
Use The Correct Level Of Governance
Data governance programs vary considerably across organizations and sectors. Successful organizations take an approach tailored to their specific needs, selecting an ideal level of sophistication for governance purposes before tailoring its class according to data analytics.
Data governance is a relatively novel concept. Banks first popularized them due to BCBS 239 1 regulations that required sophisticated governance models for banking clients under pressure from BCBS 239 1. Most other organizations and industries do not face as much regulatory pressure. Therefore, programs designed should reflect data complexity and individual regulations; organizations with multiple distinct business units or regions requiring governance should tailor solutions more precisely; organizations involving large amounts of data change and reduced technological automation create even further complexity in program designs.
An effective data governance model at a large bank would consist of an active data governance council led by C-suite executives and high levels of automation, and metadata stored in an easily searchable data dictionary with lineage to its source.
An effective data governance program for a technology firm in one region might include an informal data council that meets less frequently but includes:
- C-suite executives.
- Metadata tracking using Excel or similar.
- Limited search of lineage.
- Starting small to ensure prior use cases receive attention.
Not only should an organization establish appropriate governance across its entirety, but it should also adjust and tailor it according to each data set. Legacy data standards often place restrictive access and quality restrictions across the board - this reduces risks but can stifle creativity as well. Leaders balance risks against opportunities when setting governance for individual data sets.
Organizations may apply light governance practices when data are only used in exploration settings and within their team's limits for purposes such as exploration. As there may not be a need for fully integrated or complete data sets for exploration use within groups, masking data with NDA agreements might suffice in protecting privacy. Still, once used more broadly - for customer interactions, for instance - more robust principles must be in place.
An Asian financial institution used these principles to approach data release aggressively. First, they agreed upon its level of sensitivity before freeing each set. As a result, access to susceptible information, like personally identifiable data, was severely restricted.
As organizations advance and develop their governance and technological capacities, the scope becomes less relevant as organizations expand. When data governance activities become automated by tools like Octopi or Erwin or more established platforms like Informatica Collibra or Informatica, they will continue to reduce cost while expanding coverage - this has already begun with Octopus Erwin being released with capabilities that automate metadata harvesting and lineage creation capabilities.
Focus On Iterative Implementation
Iterative data governance principles provide an ideal solution for rapid delivery. Integrating governance into business goals, prioritizing use cases and domains, and applying needs-based command alone isn't sufficient - you must adopt iterative governance principles to get the maximum benefit out of data quality issues that have already been identified and prioritize them accordingly to deliver value fast to business objectives.
Even if your solution is imperfect, you should strive to enable priority use cases quickly. Once the value has been demonstrated, development for production-ready (i.e., integrating with core customer-relationship-management and operational customer master data) should occur over the medium- to long term. To enhance customer campaigns more quickly, combining all available enterprise data may not be necessary; instead, an integrated approach within an individual platform might suffice - supported and enhanced through data governance which addresses issues related to availability, quality, and master data management.
Generate Excitement For Data
People are more willing to ensure data quality and safety when they believe in the data-enablement vision. Leaders invest in change to convert skeptics and build advocates for data. It can be the hardest part of the project because it involves motivating staff to use the data while encouraging the producers to share the information (and improve the quality at source).
To achieve success, organizations combine interventions that encourage the correct behavior. They can be role models from senior executives, the recognition of high-quality, responsive sources, and examples of new uses. Some companies offer data-related training, qualifications, and certifications, usually as part of an academy. They also communicate about the career options available in this field. Some organizations have created excitement through events, publications, or data art.
How To Develop A Data Governance Strategy
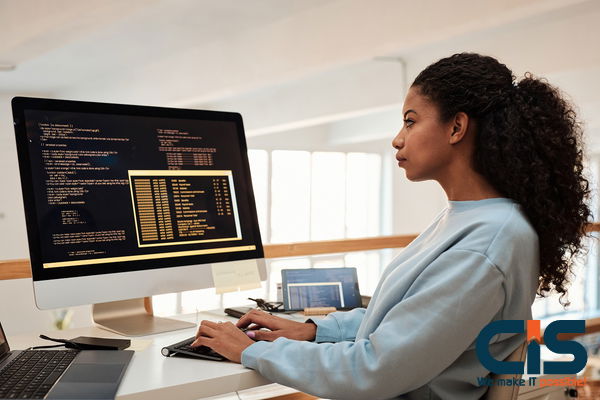
What goals is your company trying to meet through its data? For successful implementation, your data strategy must be easily understandable and communicated; otherwise, it risks only one data scientist understanding it at any one time - so governance of that strategy becomes paramount as soon as it's drawn up.
What industry are you operating in? Data strategies of companies operating in industries with stringent regulatory standards, like financial services or healthcare, tend to be defensive. The focus may be protecting sensitive health information while passing audits; governance ensures vital processes are appropriately documented for future audits. However, even within these industries, CDO success increasingly relies on positive results; therefore, defensive strategies need to take a backseat role when prioritizing results-focused CDO approaches.
Retail, for example, is less heavily regulated and therefore free to experiment with more aggressive strategies using data offensive strategy. A data offensive could involve using it to increase sales at newly opened locations or gather intelligence on rival products for product launch purposes. Governance processes will then help shape these situations: how insights are gained and which critical decisions must be made.
Personal data regulations have put these industries in defense mode; their emphasis should now be more on meeting defensive requirements than value-added initiatives. Data governance must first be in place before data can be utilized as part of an overall strategic plan. Here are seven steps for successfully implementing data management:
Find And Prioritize Existing Data
A company must know the data it has to implement a strategy for data governance. To begin this process, an organization must:
- Inventorying Data: Completely record all information resources and their metadata
- Sorting data by categories: Classify data based on their structure and content.
- Data cataloging and metadata management: Curating knowledge and data
Select A Metadata Storage Option
In the past, each department within an organization had its database for managing metadata. It has resulted in siloed information, which restricts the reuse and sharing of metadata assets. It is essential to choose a storage solution that centralizes the metadata.
- Collecting data across multiple platforms
- Reuse of Metadata for productive purposes
- Data History is Visible
- Governance and Stewardship: Effective governance
The centralization of metadata allows for the flexibility and scalability needed in analytics. This helps departments to understand how valuable data lineage is.
Prepare Metadata And Transform It
It is a time-consuming step. This requires returning to raw metadata and reformatting, correcting, and combining data sets into catalogs. Three primary tasks are involved:
- Cleaning and validating the data: Filling in missing data, standardizing data, hiding sensitive entries
- Transforming: Updating formats or values so the entire organization understands all data
- Create templates. You can create templates for business glossaries, data dictionaries, and metadata. It will allow you to organize your data vocabulary and keep track of how many terms or data assets you have uploaded within your network.
Create A Governance Model
There is no one size fits all data governance model. In the past, organizations used frameworks that were passive and compliance-oriented. They defined the way users stored, maintained, and disposed of data. To take full advantage of analytics, businesses need to adopt modern data governance systems that include:
- They can adapt to different styles of communication and contexts.
- Encourage Innovation
- Offer a dynamic, flexible strategy for the enterprise and its ecosystem
- Include rights to distribute decisions relating to value
- Manage risk actively by taking an active approach
Choose an effective governance model - either centralized or decentralized, depending on the organization's needs: Model of Centralized Governance: When adopting this method of data governance, one group establishes rules about it - this group determines what data elements and terms need approval while discussing any core processes every member must abide by. When multiple groups manage data together, a federated model comes into effect that works well when different departments require access.
Create a Distribution Process
Data governance policies are only effective when they're followed. For this reason, policies work best when integrated into people's typical workflows and tools. Organizations should take into consideration the following to mitigate their regulatory risks effectively:
- Appropriate employee onboarding
- Employees are trained on usage guidelines and policies
- Encourage knowledge sharing between employees
- Create processes to request and make changes
Recognize Potential Risks
Security laws and requirements for compliance are constantly evolving. Companies are required to implement appropriate security measures under the California Privacy Rights Act and General Data Protection Regulation.
Data governance strategies should consider the following risks:
- Access to data fields should be limited: no one should have more access than is necessary for their work.
- All sensitive storage areas must have appropriate security measures in place. This will prevent hackers from gaining access to or stealing the data.
Read Also: Developing a Robust Framework for Data Management
Constantly Adapt Your Data Governance Framework
Data strategies and business models change as well. Data governance is a process that companies must continually adapt to and improve. They can then respond quickly to emerging issues, such as the growing privacy risk of data. Automated tools are needed to help track and evaluate the success of business strategies.
- Check policy compliance
- Measure data usage
- Enforce consistent data quality
- Analysis of curation
Through The Process: Promote A People-Centric Approach
Data Catalog automatically assigns assets stewardship based on actual usage patterns to foster data governance and encourage accountability of assets within it. Stewards or people knowledgeable in data are crucial when adding new assets; identify these experts through creating and adding to a catalog, to maintain governance standards over the future purchases added by creating Catalog of data assets.
Encourage employees to act by rewarding them for their efforts with certifications and badges or recognize employees with monetary bonuses, gifts, and additional time off. Your approach encourages users to take ownership of their knowledge source, thereby giving confidence in it, thus giving users more leverage.
Want More Information About Our Services? Talk to Our Consultants!
Last Thoughts
Data governance processes can be an indispensable asset when supporting strategic initiatives. Gaining community support for data stewards' work is essential; people need to know why and how their departments use information from an organizational point of view and have automation technologies (machine learning, crowdsourcing) available to maintain processes.
Data production at organizations continues to accelerate rapidly. Citizens need solutions for data governance that enable them to enhance and enforce processes continuously. Monitoring and measurement tools play a significant role in helping improve processes by allowing users to compare curation with policies and standards.
An effective data governance program requires individuals to take ownership. Businesses need to devise strategies quickly adopted by teams and implemented into practice. approach to data management puts people first. Companies can launch organic data governance based on what people already do; once a practical framework has been established, businesses can develop iterative processes for continuous improvement iteratively without interfering with day-to-day operations or disrupting routine procedures. connects technology, people, and strategy; it empowers its participants by giving access to an expert group.