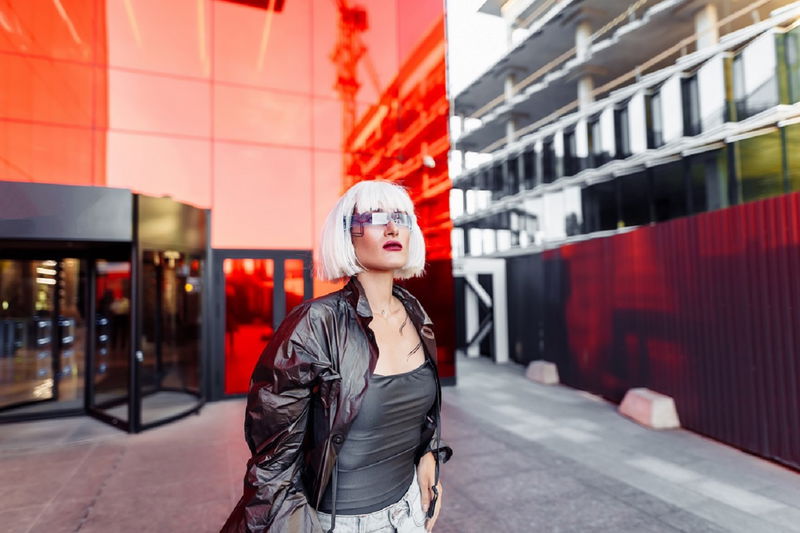
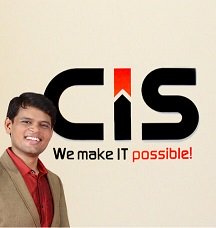
Contact us anytime to know more β Amit A., Founder & COO CISIN
Computer science, data analysis, statistics, hardware, software engineering, and philosophy are just a few of the numerous fields that AI embraces.
A group of technologies that are primarily based on machine learning and deep learning are referred to as artificial intelligence (AI), which is an operational level that businesses may employ. It is utilized for forecasting, object categorization, natural language processing, big data analytics, and forecasts.
Different Types Of Artificial Intelligence
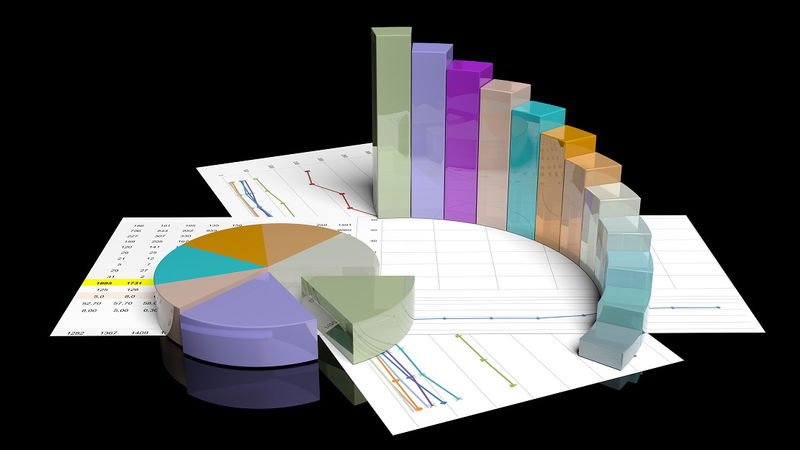
Artificial intelligence can be arranged in various ways depending on the stage of development and the activities being taken.
Typically, AI development goes through four stages.
Machines that react with Limited AI that only responds to preprogrammed stimuli. It has no memory and is unable to learn from new information.
Feeble memory The most advanced AI is believed to have minimal memory. Memory allows it to continuously enhance its performance after training with new data using artificial neural networks or other training models.
A part of machine learning is called deep learning.
Concept of mind: Although AI is not yet available, research into its potential is ongoing. It is an artificial intelligence (AI) with decision-making skills on par with a person's.
This entails being able to identify and recall emotions and reacting to social events in a human-like manner.
Self-awareness: Going beyond AI's idea of mind, self-aware AI is a hypothetical machine that possesses human-like emotions and cognitive capacities and is aware of its existence.
Theory of mind AI is also yet to be feasible, as is self-aware AI.
Artificial intelligence can more easily be categorized based on its capabilities. Because of its training and programming, artificial intelligence is only trained to carry out a finite set of tasks.
Natural language processing will not be a capability of an AI algorithm to classify items. Examples of narrow AI include Google Search, predictive analytics, and virtual assistants.
Machine intelligence that can "detect, think, and act" like a person is known as artificial general intelligence (AGI).
AGI is still improbable. The next stage is artificial superintelligence (ASI), where a machine can perform all tasks better than a person.
Artificial Intelligence Training Models
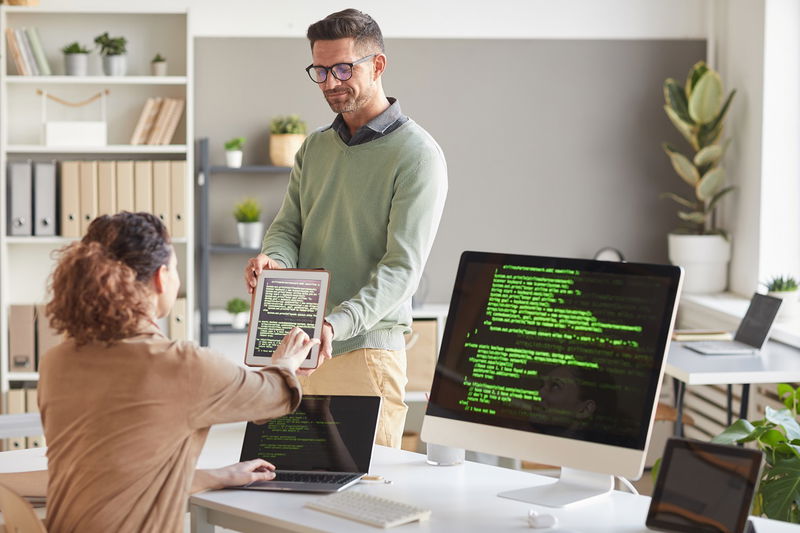
When discussing AI in the workplace, "training data" is frequently used. What does this signify, though? Remember that limited-memory AI can be educated with new data to improve over time.
A branch of artificial intelligence called machine learning uses algorithms to train data to produce outcomes. Machine learning uses three different types of learning models. A supervised learning approach uses labeled training (structured) data to map an input to an output.
Give an algorithm photographs labeled "cats" to teach it to recognize images of cats.
Unsupervised learning is machine learning that discovers patterns from unlabeled, unstructured data. Unlike supervised learning, the outcome of unsupervised learning is not known in advance.
Instead, the algorithm learns from the data and groups it according to its characteristics. Unsupervised learning excels at descriptive modeling and pattern matching, for instance.
Along with unsupervised and supervised learning, semi-supervised learning is also an option. Only specific data, nevertheless, are tagged.
In semi-supervised learning, the outcome is predetermined. Still, the algorithm must organize and arrange the input to produce the required results.
A machine learning model known as reinforcement learning is generally called "learn from doing." The agent uses trial and error (a feedback loop) to learn how to accomplish a task until it reaches the required level of performance.
Positive reinforcement is given to the agent when it performs well, and negative reinforcement when it doesn't. Robotic ball grabbing could be taught via reinforcement learning.
There Are Many Types Of Artificial Neural Networks Types
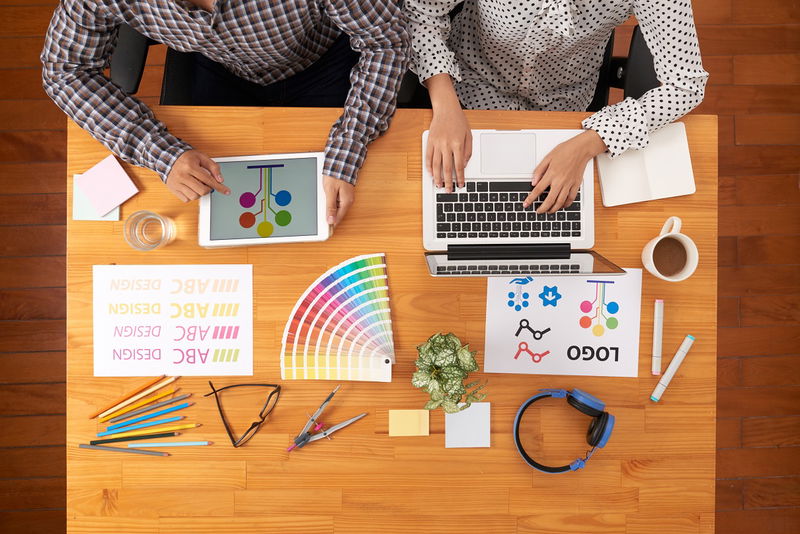
A typical kind of AI training model is a neural network. It takes some loose cues from the human brain. A neural network is a group of synthetic neurons, often known as perceptrons.
These are the nodes used in computation to categorize and examine data. Each perceptron chooses to transfer its knowledge to the following layer. Training models with more than three layers are called deep learning or deep neural networks.
Numerous neural networks have thousands or even hundreds of thousands of layers. The last perceptrons complete the task given to the neural network. They can, for instance, categorize an object and look for patterns in data.
The most well-known artificial neural networks you might come across are:
One of the first varieties of neural networks is the feedforward neural network (FF). Data travels in a single direction across layers and synthetic neurons until it reaches its goal.
With numerous layers and more than one hidden layer, contemporary feedforward neural networks are frequently referred to as "deep feedforward" networks.
Feedforward neural networks are frequently used in conjunction with the "backpropagation" error-correcting process.
Simply put, this starts with the output of a neural network and proceeds backward to identify mistakes that can be fixed. A powerful and straightforward type of neural network is deep feedforward.
Recurrent neural networks (RNN) differ from feedforward neural systems because they can incorporate sequence-containing or time-series data.
Feedforward neural networks, which apply weights at each node, are distinct from recurrent neural networks. In its place, they have "memory," or a portion of the layer's output. Any additional words in a sentence can be "kept in mind" by RNNs when they process natural language.
RNNs recognize speech.
Long/short-term memory (LSTM) RNNs are a cultivated variety that can "remember" earlier levels. The primary distinction between LTSM and RNNs is that LTSM uses "memory cells" to remember what happened in earlier stories.
In speech prediction and recognition, LSTM is frequently employed. Network of convolutional neurons (CNN).
Feature a few of the most well-liked neural networks used in contemporary AI. The most popular use for CNNs is image recognition.
Before joining the filtered portions of the image back together, they use multiple layers (a convolutional and then a pooling). The first convolutional layers might have concentrated on specific elements like edges and colors. Still, the last layers will search for more intricate features.
A game in which two neural networks compete against one another ultimately increases the accuracy of the output.
The discriminator seeks to confirm or refute the examples that the generator generates. can be used to produce artistic or realistic images.
Machine learning and artificial intelligence technologies have advanced significantly in recent years. Organizations of all sizes now have access to these capabilities because of platforms like the cloud, AI, and machine learning capabilities.
However, seasoned technology professionals know that just because a technology is available for purchase does not guarantee that it will be adopted or deployed. It might get put on a shelf.
The ERP supply chain and manufacturing programme, finance and procurement, human resource management, customer expertise programs, sales, support, and advertising are a few.
He claims that the impact is revolutionary and that some apps powered by AI or system learning are assisting in boosting efficiency throughout the entire firm.
The usage of machine learning and artificial intelligence is rising due to these promises for good change. According to reports, the amount of knowledge, sophisticated algorithms, and super-fast computer capabilities make AI conceivable today.
However, he cautions that even if the technology is accessible, individuals will still use it. He suggests the following to promote the acceptability of AI and machine learning: Introduce attributes that are helpful to end users, even the smallest ones.
According to a report, such as slide-outs and alerts, are made to provide customers with hints about new AI features in the user interface.
According to the report, other suppliers will imitate him.
Ensure the best data is applied to the correct small business problem: Obtaining quality, trustworthy information from the appropriate sources is another crucial factor, in addition to consumer familiarity with AI.
According to the report, the issue determines the data needed for AI and machine learning. You can divide the problem into multiple sections to identify customers for targeted digital advertising. How can I tell which client is the most dependable? Can a customer value score be determined based on prior purchases, lifetime values, and social media activity? The information I have regarding the endeavor must then be known to me.
Metadata and target demographics are included here. Then, you may locate and rate the most suitable customers for your advertising campaign using machine learning, deep learning, and learning-to-rank techniques.
Double-check data to ensure it is accurate: The fight starts by putting fixed and mutable data from various independent sources onto a single platform.
Not only must the data be entered, but it must also be verified as being accurate and updated. Maintaining data quality and performing tests at the data ingestion stage is crucial. This illustrates what may go wrong if the information provided in the system is not checked.
Consider expanding the training dataset to include ages. Although your range was 23 to 68, a data-collecting mistake resulted in readings of 220. The checks would stop units from adjusting their weights in extreme conditions.
Useful Evaluation: According to the report, exploratory data analysis and the usage of feature technologies are becoming more crucial to machine learning and AI.
These methods are essential for producing important characteristics and insights that aren't there in raw data. Some feature scaling and attribute transformation phases must be completed before the data can be delivered to the algorithms.
The version can use procedures from previous data sets to forecast patterns in upcoming data points.
Ways Artificial Intelligence Can Help Businesses: Uses and Examples
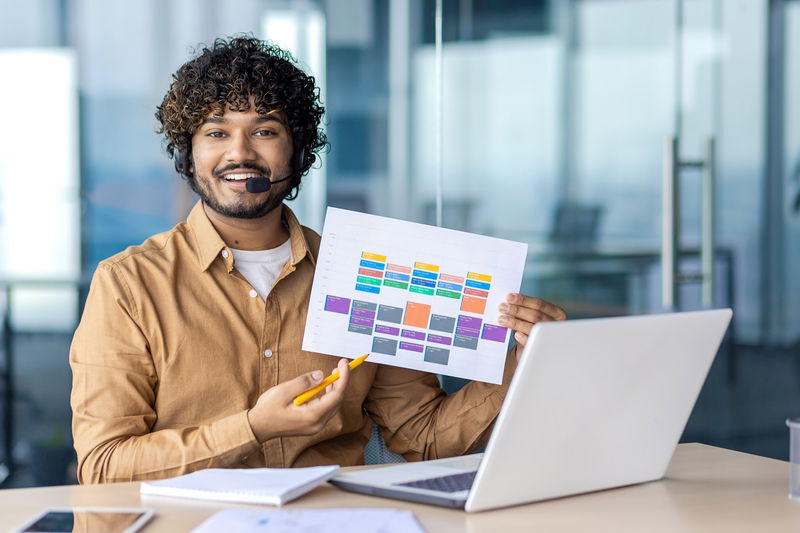
Leading businesses understand the value of artificial intelligence and how to leverage it. Artificial intelligence technology helps both small and large organizations since it helps them develop and make better decisions.
It alters management as well.
What effects does AI have on business? What business benefits may AI provide? We'll provide you with the response.
The article is as follows:
- AI definition Definition.
- What role does artificial intelligence play in the corporate world?
- Applications, advantages, and roles of AI.
- Examples of AI applications that can help enterprises
- most widely used AI software products
- PDF infographic
Why Is Artificial Internet Important For Business?

Let's begin with the definition.
Artificial intelligence implementation is the term used to describe a machine's capacity to carry out tasks that a human would typically do.
Artificial intelligence can be defined as the capacity for decision-making and interpersonal interaction. Making machines think and act like humans is known as artificial intelligence or AI.
AI typically doesn't take the place of people. It improves our abilities and changes the way we work. The implementation of AI makes use of software tools.
What's Artificial Intelligence software?
Artificial intelligence software automates various operations using unique algorithms and AI technologies. Businesses can save time and increase productivity by automating business operations.
You can utilize various AI software applications to streamline and improve the efficacy of your company processes.
Software that suggests products in an online store, chatbot software, content production automation software, sales forecasting tools, and software that automates content development.
AI will change every industry sector and organization. A corporation looking to the future must have an AI strategy and knowledge.
Your AI strategy will give you significant competitive advantages and enable you to accomplish your business objectives.
AI has various applications. It can do data analysis, cybersecurity defense, and customer service. This promotes better customer service, reduces energy costs, forecasts sales, and makes firms more customer-focused.
Additionally, more marketing and sales departments than any other component of an organization today (40%) place a high value on AI and machine learning.
Now, let's examine how AI may provide you with a competitive edge, boost productivity, and spur revenue growth.
How Does AI Work Help Your Business To Grow
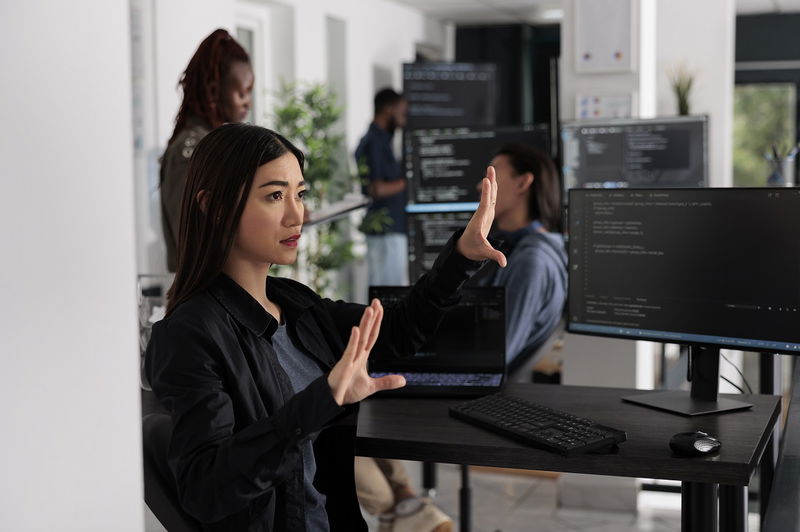
Recommendations for Product Development can Boost Revenue
Systems for recommending products frequently use AI. These systems provide customers with product and information recommendations based on unique data analysis techniques.
Daily product recommendations are common for online shoppers.
Customers might be assisted in their purchase decisions by offering product recommendations. You can find it in emails, advertising, and eCommerce websites.
The recommendation may change depending on various variables, including past customer purchases and similar user activity.
Chatbots for Customer Service Enhancement
Systems for recommending products frequently use AI. These systems provide customers with product and information recommendations based on unique data analysis techniques.
Daily product recommendations are common for online shoppers. Customers might be assisted in their purchase decisions by offering product recommendations.
You can find it in emails, advertising, and eCommerce websites.
The recommendation may change depending on various variables, including past customer purchases and similar user activity.
Make the Best Content Marketing Strategy
Every day, untold numbers of individuals look for goods and services online. You must present both yourself and your company to them.
How? You may achieve this via effective content marketing. Through distributing helpful content, content marketing strives to draw in, hold the attention of, and please customers.
Content marketing comes in various formats, including blogs, social media posts, and podcasts. In content marketing, artificial intelligence is becoming more critical.
Algorithms are used by artificial intelligence to analyze data and create the most effective marketing content strategy.
You can pick the most excellent option if you discover that a particular kind of material is in high demand. AI can assist you in deciding what to write about, what keywords to use, and how to write. You can also use AI to create material that is of a high caliber and ranking.
AI can perform a wide range of tasks that might benefit your company and market, including keyword research, content creation, and social media posting scheduling.
The benefits of AI in content marketing
Many companies go above and beyond the fundamentals to advance their marketing strategies and produce high-caliber blogs, compelling social media posts, exciting films, and engaging webinars.
Utilize tools based on AI to power content.
Using Sentiment Analysis to Assess Customer Feelings
Sentiment analysis can examine and track people's thoughts and sentiments in various text formats. Text can refer to dialogues on blogs and social media platforms as well as reviews of goods and services.
Businesses can use sentiment analysis to find and gauge client feedback in the millions of online reviews and forums.
By combining various forms of social media data and using AI in sentiment analysis, organizations may analyze massive volumes of data, comprehend their customers' emotions, and learn about their wants, intentions, and preferences.
Effective competitor intelligence, Thanks to AI, research and analysis, competitive intelligence is now simpler than ever.
Competitive intelligence is gathering and evaluating data on your sector, rivals, and business environment.
Every action taken by your rivals, from promotions to merchandise to personnel, may be followed.To make wise selections, it is crucial to find out from other companies in your field what works.
Using artificial intelligence, you can spy on, monitor, and comprehend the actions of your rivals and identify the factors that contribute to their success.
Sales Forecasting to Grow Your Business
The technique of predicting and projecting future sales is known as forecasting sales. It has a significant role in predicting long-term business growth.
Forecasts can assist you in identifying possible issues before they escalate.
If your team's trend is 28% below quota, you can determine why. Your rival may be running a very alluring discounting campaign.
Businesses may make short- and long-term predictions and resource allocation decisions using accurate sales forecasts to determine the likelihood of customers making purchases.
This AI-based programme analyzes CRM data and purchase history and deals with history information.
Emails, phone calls, and meetings are among the data items examined. Then, text mining is used to ascertain how they link to sales outcomes.
The AI programme examines the relationship between each data point and sales success before assessing a customer's following action to produce a precise prediction.
Optimize your Price
Customers purchase goods from merchants, particularly if given a favorable price. Price is essential, according to studies.
Even though we may be in a value-oriented era, money is still king. Your price is affected by the competition, brand reputation, and past price changes.
All these elements can be successfully considered by artificial intelligence software when figuring out the ideal price.
It will assist you in choosing the right price for each product across all channels and seasons.
- Define optimal prices first.
- Prices like these will draw clients and bring in the full money for you.
- AI software may analyze a lot of data to get the optimum pricing.
- AI's advantages in pricing optimization
Intelligent Cybersecurity
Credit card firms use AI more and more to assist financial institutions in preventing billions of dollars in fraud annually.
Cybersecurity is crucial for all organizations, not just financial ones. It is critical to a variety of sectors, including utilities, retail, consumer products, and telecom.
Read More: What Is The 9 Reasons Behind Choosing A Web Development Company?
The prevalence of cyberattacks is rising as digital firms grow. Numerous risks, including identity theft, account takeovers, and vengeful ransomware, could affect a firm.
Cybersecurity issues can be handled by AI software in various ways, such as vulnerability management, phishing detection, and network security.
Additionally, it has network security, preventative management, and behavioral analytics.
Enjoy Delightful in-store Experiences
Physical stores continue to dominate sales despite the rising interest in online shopping. With tailored service that delights customers, artificial intelligence may reinvent conventional in-store encounters and elevate them to a new level.
By offering immediate access to customer care, rapid checkout, product support, and personalized suggestions, AI may enhance the shopping experience for customers.
Retail is a very intriguing and promising application of AI. In-store When a consumer last visited the store, AI may track their previous purchases and determine.
With the help of this data, AI may recommend appropriate things and offer individualized benefits like savings or loyalty points.
AI-powered gadgets can read face clues, like voice-activated webcams. Customers' feelings and reactions can be recorded to provide the appropriate goods or support.
Want More Information About Our Services? Talk to Our Consultants!
Conclusion
Businesses may automate various activities using artificial intelligence technology, which also helps them save time and increase productivity.
AI enables you to automate routine operations and produce more work at a cheaper cost. AI can speed up your company's operations and eliminate menial jobs so staff can focus on more crucial work. Your workforce is your most important resource.
By automating their less crucial and time-consuming duties, AI can help them save time.