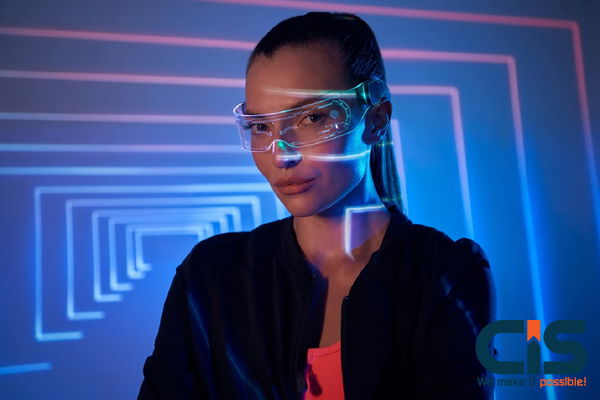
This data can be used by businesses to improve their ROI, staff performance, product quality, and many other things. The use of big data analytics by firms has been a game changer for those who do it. Market strategy is driven by business information and analytics. This article will define "big data", its benefits and the best ways to use it in a wide variety.
Platforms are becoming the best analysis tool for intelligent data management. It is a big business to provide Big data solutions. These innovative data platforms create beautiful graphics from your data mountain. They offer analysis that is relevant to your target audience. How do you make the right business decision from these tools?
Every second, organizations produce unimaginable amounts of data. Real-time analytics helps organizations increase revenue and customers. Social media companies such as Twitter and LinkedIn are using real-time streaming technologies. The classification and synthesis of stream processing systems can help identify issues and improve future systems.
We will use the taxonomy to compare and analyze the latest open-source streaming computing technologies. Researchers will be able to better understand the capabilities of stream platforms with the taxonomy. The survey will help companies choose the right stream processing solution for their domain-specific needs.
Big Data Definition: Five Values
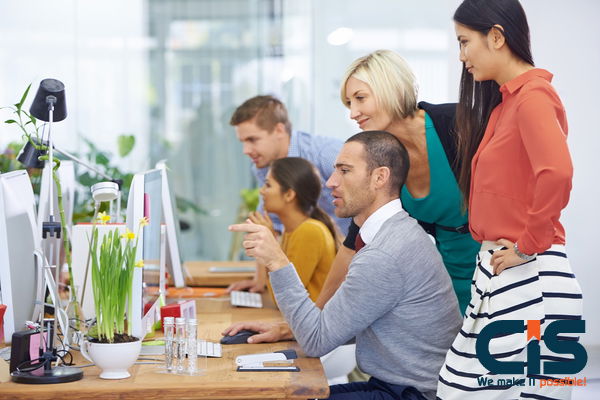
"Big Data" refers to data that is too large or complex to analyze using traditional data processing methods. To better understand this term, consider the five aspects of big data: Unstructured data in large quantities, like data streams from Twitter, could take up terabytes and even petabytes. To put it in perspective, the average Word document is only a few hundred Kilobytes.
More data is generated as more people use the internet, requiring more processing power. Look at the different file types in your database, including MP4, DOC and HTML. You'll discover that your data is much more diverse when you examine additional file extensions. Is all big data worth it? How can your company derive value from it? Data scientists consider several criteria to assess the importance of large amounts of data. "The Five Vs" are a common name for these.
Volume: Because "large" is relative, the amount of data produced determines whether or not it's considered "big data." This statistic can help businesses determine if they need big data solutions to manage their confidential information.
Velocity: Data's utility will directly correlate with the speed at which they are created and transferred between systems.
Variety: In today's society, data is collected in many different ways. These include apps, websites, social networks, audio and video, sensors, smart devices and other sources. These various pieces of information are a component of corporate intelligence.
Veracity: Data from different sources can be inaccurate, inconsistent and incomplete. Data that is accurate, consistent, and comprehensive data can add value to corporate analytics and business intelligence.
Value: The value of big data to an organization depends on the value it adds.
Big data management differs from conventional methods due to its sheer volume and diversity. Before complex, large data sets can be absorbed by analytics and business intelligence systems. They need to be cleaned, transformed, and processed. To provide real-time insights, big data requires innovative storage space and computation options.
What is a Big Data Solution?
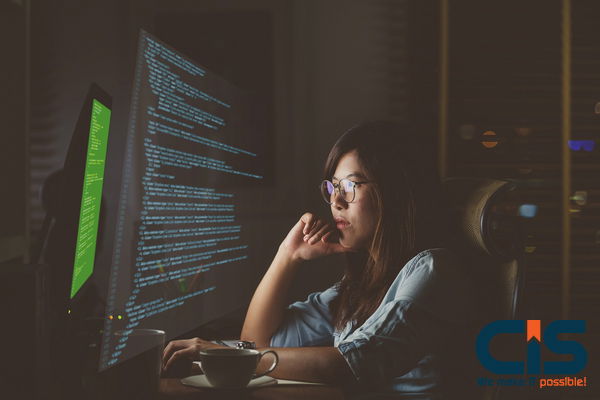
Before investing in a solution for big data, evaluate the data that is available to analyze, the insights that can be gained from it, and the required resources to develop, build, and implement the platform. The right questions can be a great starting point when discussing solutions for big data. Use the questions in the article as a guide to your research. Questions and answers will shed some light on the data and the topic.
Businesses may have a general idea of what information needs to be reviewed, but the details are unclear. Data may reveal patterns that were previously unknown. If one is discovered, it will be necessary to do further research. Start by creating a few simple use cases. You will be able to collect data previously unavailable and discover unknown unknowns. The ability of a data scientist to identify important data and create predictive and statistical models improves with the establishment of a data repository and the collection of more data.
The company may know about the information gaps within itself. The first step in solving these unknowns is identifying external data sources. To do this, the business should work with data science.
What Crucial Actions Comprise Big Data Solutions?
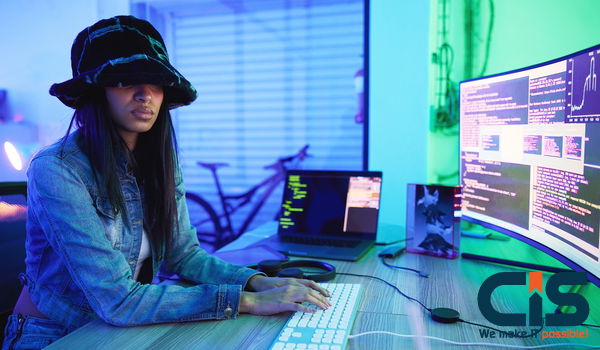
The following steps are required to implement big data analytics:
- Data Ingestion: The first step is to collect data to deploy big data solutions. This can be from an ERP system such as SAP, CRM systems, relational database management systems (RDBMSs) like MySQL and Oracle, log files, files flat, images, documents or social media feeds. HDFS must be used to store this data. Either once-per-day, once-per-hour, or once-per-fifteen-minute batch tasks, or real-time, 100-ms-to-120-second streaming may take in data.
- Data Storage: After data ingestion, it is necessary to save the data into HDFS or NoSQL databases, such as HBase. The HDFS filesystem is more suited to sequential access.
- Data Processing: At the end of the day, you will want to run your data through a processing framework. ).
Big Data Solutions: The Best Big Data Solutions
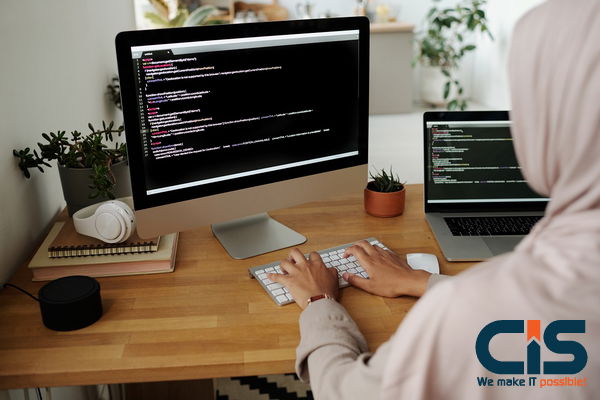
#1. Apache Hadoop
Apache Hadoop, a free and open-source distributed file system, was designed to quickly process massive amounts of data across clusters. It can also grow to meet the demands of any organization. It is one of many prominent solutions for big data storage. NoSQL distributed databases (like HBase), which allows data to be spread over thousands of servers without affecting performance, are supported. Both on-premises and the cloud big data solutions are possible.
Benefits
Data replication allows consistent access to sensitive information, even when spread over multiple servers and storage devices. To facilitate low-latency data retrieval, a cluster-wide load balancer distributes data uniformly across drives. Hadoop sends the code bundle to all the cluster nodes and then distributes files for local parallel data processing.
Its increased scalability, availability and reliability are a benefit to business owners. Application-level errors can be detected and corrected. It is easy to add YARN nodes into the resource management to run tasks and to remove them to scale down the cluster. Users can direct the program from a central point to store blocks of data they choose in local caches on multiple nodes. When using explicit pinning, users can keep only a limited number of blocks in the cache buffer, allowing them to free up memory for other uses.
Hadoop ensures data integrity by relying not on replicating data but rather on snapshots taken at a specific time to preserve block lists and file sizes. It logs changes to the file system in reverse chronological order to quickly retrieve current information.
Limitations
- Only batch processing is supported. It runs slower in general because of this.
- The system is inefficient for iterative processing because it does not allow cyclic data flows.
- The storage layer of encryption is not enforced. Security is provided by Kerberos authentication, which can be difficult to maintain.
#2. Apache Spark
Apache Spark is a powerful open-source computing platform that can process data in batch and real-time. Spark's "in-memory computing" architecture keeps intermediate data and disk I/O in RAM, allowing lightning-fast processing speeds. It was designed to complement Hadoop and is compatible with Java, Python R, SQL and Scala. MapReduce is an extension to Spark that allows for interactive queries and streams of data to be processed at the speed of thinking.
Benefits
During deployment, Spark can run on Apache Mesos or YARN clusters or be started manually using launch scripts. Users can run all daemons for testing and development on one host. Spark SQL: Spark SQL allows data queries through SQL, a DataFrame API and supports many data sources, including Hive. Parquet. JSON. JDBC. It allows access to existing Hive warehouses as well as connections to business intelligence software by supporting HiveQL syntax and Hive SerDes.
Limitations
- Deployments may be vulnerable to attacks if they are not configured correctly, as security is disabled by design.
- It doesn't appear that there is any version compatibility between the major versions.
- A lot of RAM is used by an in-memory processor.
#3. Hortonworks Data Platform
Yahoo created Hortonworks in 2011 to help large companies transition to Hadoop. Hortonworks Data Platform is an open-source and free Hadoop distribution. The company also offers competitive in-house knowledge, which makes it a good option for businesses looking to adopt Hadoop. HDFS, MapReduce, Pig, Hive, and Zookeeper, to name a few, are included in the Hadoop project list.
HDP is open-source, with Ambari, Stinger, and Apache Solr, for query processing and data searching. HDP is known for its uncompromising open-source adherence and includes zero proprietary software. HCatalog, a component of HDP, facilitates communication between Hadoop-based programs and other business applications. It was the enterprise-wide big data solution of choice.
Benefits
Deploy anywhere: This solution can be deployed either on-premises or in the cloud as part of Microsoft Azure HDInsight. It is also available as a hybrid option called Cloudbreak. Cloudbreak is a hybrid solution that offers resource efficiency through elastic scaling. It's designed for businesses with existing on-premises IT infrastructure and data centres. Scalability and high availability: NameNode federation allows a company to expand its infrastructure so that it can accommodate thousands of nodes and billions of files.
NameNodes manage the file path and the mapping information and are completely independent. The result is increased availability with a lower total cost of ownership. Erasure coding also improves data storage efficiency, which allows for more effective data replicas.
Apache Ranger, Apache Atlas and Apache Atlas provide the ability to trace data from its origin point all the way through to a data lake. This allows for the creation of audit trails that govern classified or confidential information. Reduced Time-to-Market: This technology allows organizations to launch apps in just a few minutes, which reduces the time required to get products to market. GPUs allow for the integration of deep learning and machine learning into applications. This company's hybrid data architecture provides unlimited cloud storage of data in its original format. Cloud storage is available in ADLs WASB S3, GCP, and GCP.
Limitations
- It is difficult to implement SSL on a Kerberized Cluster.
- HDP is part of Hive, but data cannot be subjected to additional security measures.
#4. Vertica Advanced Analytics Platform
Vertica was acquired in 2017. Vertica Analytics Platform is similar to Hadoop in that it uses massively parallel processing. However, unlike Hadoop, Vertica Analytics Platform also includes a relational database of the next generation, SQL and ACID transactions. Vertica Analytics Platform is great for real-time analysis, while Hadoop excels at batch processing. The two platforms work together through several connections. For example, an HDFS connector allows data to be uploaded into the Vertica Advanced Analytics Platform.
Benefits
Resource Management: With its Resource Manager, the user can allow simultaneous workloads to run efficiently. It can reduce CPU and memory usage, disk I/O processing times, and data compression by up to 90 per cent without compromising information. Massively parallel processing, active redundancy, including automated replication are all features of its SQL engine. It's a high-performance analytical database which can be installed either on-premises or in the cloud. It can be used on Amazon, Azure, Google and VMware clouds.
Data Management: It is well-suited to read-intensive tasks because of its columnar storage. Vertica supports a variety of file formats as input and can upload data at speeds of up to several gigabytes/second per machine per load stream. Data locking is used when multiple users are accessing the same data simultaneously.
Integrations: It helps analyze data from Apache Hadoop and Hive as well as other data lake systems by using standard client libraries such as JDBC and ODBC. It integrates with BI systems like Cognos Microstrategy and Tableau as well as ETL products such as Informatica Talend and Pentaho.
Vertica combines database functionality with analytical capabilities, such as machine learning and methods for classification, clustering, and regression. Businesses can use the geospatial analysis and time series capabilities of Vertica to quickly analyze incoming data without having to purchase additional analytics tools.
Limitations
- No support is provided for foreign keys or referential integrity checks.
- Automated constraints are not supported when using external tables.
- It can take a long time to delete something, and this could cause other tasks to be delayed.
#5. Pivotal Big Data suite
This comprehensive system for data warehouses and analytics. Pivotal HD's Hadoop distribution is equipped with tools such as YARN and SQLFire. GemFire XD is a NoSQL NoSQL database which runs in memory on HDFS and allows for real-time analysis. It supports SQL, MapReduce parallelism, and large data sets of up to hundreds of Gigabytes. Pivotal Greenplum supports a wide range of cloud providers, including Amazon Web Services, Microsoft Azure, Google Cloud Platform and VMware. Kubernetes is used to automate and repeat deployments. It also provides stateful data persistence for Cloud Foundry applications.
Benefits
Greenplum's MPP architecture and analytical interfaces are compatible with the PostgreSQL open-source community. Pivotal GemFire High Availability includes automated failovers to other nodes within the cluster in case an operation fails. Grids automatically balance and rearrange themselves when nodes are added or removed from a cluster. Using WAN replication allows for multiple sites to be used simultaneously as DR.
Pivotal Greenplum, a scalable analytics database, supports R, Python and Tensorflow, as well as deep learning and machine learning. It provides text analytics with Apache Solr, while GPText is a geographical analytics tool using PostGIS. GemFire's horizontal architecture, which uses in-memory processing to process data, is tailored for low-latency applications. This allows for faster data processing. By sending queries to nodes with the relevant data, the response time is reduced. The results are also presented in an easy-to-read data table.
Want More Information About Our Services? Talk to Our Consultants!
Big Data: Benefits and Uses
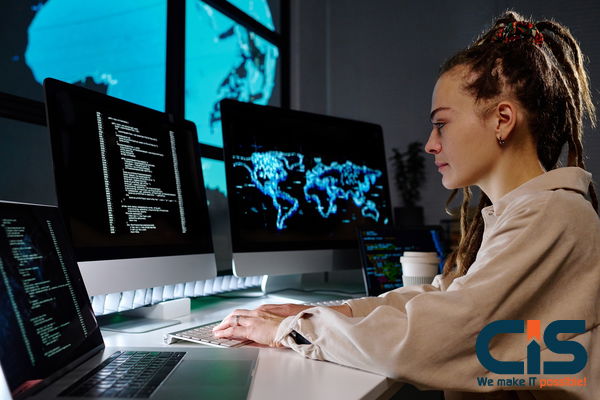
Big data platforms such as Hadoop or Spark offer tremendous cost savings when storing, processing and analyzing huge amounts of data. The cost-cutting advantages of big data are best illustrated by an example from the logistics industry. The cost of returns is typically 1.5 times higher than the standard shipping costs. By assessing the likelihood that a product will be returned, businesses use big data and analytics in order to reduce costs. Businesses can then take the appropriate steps to reduce product return losses.
By allowing you access to vast amounts of valuable customer data through your interactions with customers and their comments, big data solutions can increase operational efficiency. Analyses can then be used to extract patterns relevant to the data in order to create tailored products. The technology can automate routine procedures and activities to free up time for cognitive tasks.
Big data analytics is essential to innovation. You can use big data to develop new products and services while improving existing ones. Data collected in large quantities helps organizations determine what is best for their clients. Knowing what other people think about your products and services can be beneficial to product development. These insights can also be used to improve marketing, customer service, and staff efficiency.
In today's highly competitive market, companies must develop protocols to allow them to track feedback from customers, product success and competition. Big data analytics projects allow for real-time monitoring of the market and keeps you in front of your competition. The use of big data predictive analytics technologies is key to growing businesses.
What To Consider Before Implementing Big Data
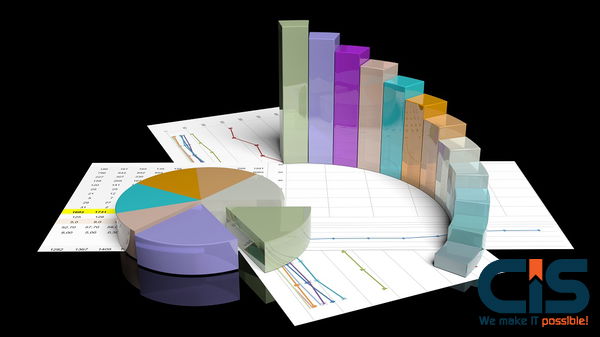
Big data has become a major focus in the marketing, human resource, finance and technology departments around the globe. However, this exciting venture comes with its challenges, especially in terms of privacy and compliance.
#1. Security is a Priority
The IoT network is growing as businesses collect data from a variety of sources. These include laptops, desktop computers, smart devices like mobile phones, and tablets. This wealth of information can be a burden to firms in today's corporate world, where hackers are everywhere and never stop finding new ways to gain access to networks and steal data. Your worries about data security will increase as you collect more big data.
#2. System Integration for a Reliable Big Data Environment
It is important to ask yourself the following question before you start your own Big Data project. Does your computer have the ability to handle data for data analytics and data visualization with Big data visualization tools? When it comes to dynamically altering data in order to turn it into the tool you desire, many firms use outdated technologies. Your firm needs to invest in the right big data solution architecture if it wants to make the most of its big data.
#3. Employee Education
It may prove difficult to find and hire skilled individuals at first, as big data is a relatively new technology. This skill will also be difficult to locate. Consultants are often hired by firms who are just starting out with big data to help them gain the necessary knowledge. It can take a long time to find an in-house data scientist since they must possess exceptional math and computing skills as well as the ability to spot patterns and trends within data.
#4. Appropriate Budgeting
If you consider the factors of security, personnel, and system integration that we have discussed, your budget for tackling big data may soon be exceeded. The cost of analyzing big data and displaying it is high, even though the costs of storing and gathering data are low due to cloud hosting and storage and complex data sets. Businesses must also consider the future outcomes of their investment to determine if it is worth the initial cost.
#5. Put Data-Driven Conclusions into Action
After you have created a safe, cost-effective environment to store your big data and recruited the best data scientist to examine the data, it's time to decide what you will do with the data to make the effort worthwhile. It is important that businesses use the data they collect and analyze in a practical and profitable way. Asking meaningful questions about data is an important tool that firms use.
How to Implement Big Data?
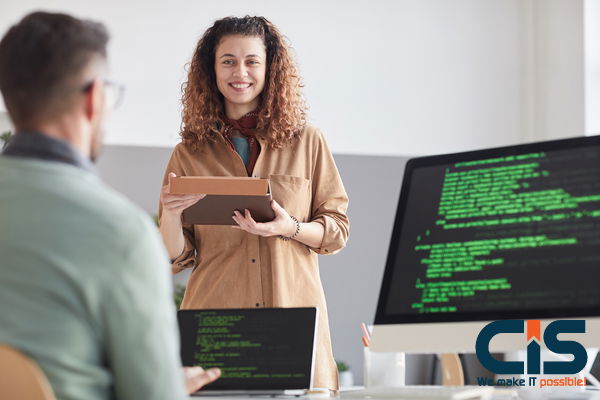
#1. Choose The Right Tools For Your Team And Budget
You're in luck if you have a team that is project-focused. Find specialists if you don't have one. Sponsorship could also be required. Big data initiatives can be time-consuming and expensive. Calculate the costs to determine if you need sponsorship. Open-source solutions are also available if you don't want to invest in enterprise software.
#2. Obtain Data
To collect relevant data, you'll have to identify the data sources. Before moving forward, you should identify, assess, and prioritize them. Data lakes may store data. Data lakes can hold both organized and unstructured data. Lakes are flat storage of data, unlike data warehouses. Data lakes can be deployed using cloud technology or on-premises. This will serve as a staging level for your system in cloud services.
#3. Create Data Hubs
Create data hubs by combining transformations with predictive analytics. You can use this information to learn about the data and alter your processes. Avoid project failure by letting things move at a gradual pace.
#4. Validation
Testing, measuring and learning are essential to the analytical process. Test your assumptions as you gather more data. Big data visualization tools simplify data management and project execution. These tools will allow you to better understand massive data sets and improve outcomes.
4 Crucial Questions to Ask Your Big Data Solution Provider
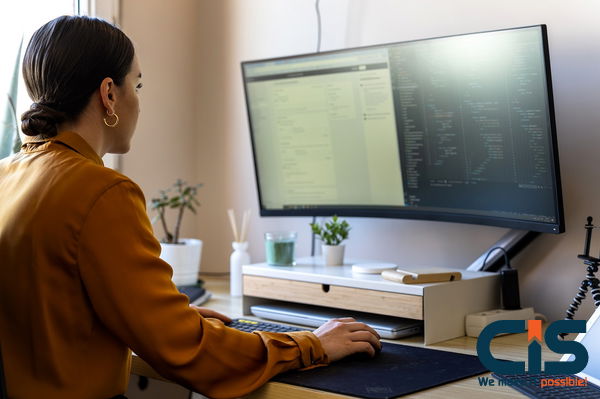
A Reliable Partner: You can be sure that your Big Data Solution Provider will remain at the forefront of intelligent data if it becomes vital for your business's future. Before making a decision, it is essential to consider the long-term benefits. It is a good idea to work with a trusted partner, who you can easily access for technical support.
It is essential to select a technology that is easy to use. Hence, you can continue the relationship even if your supplier leaves or you decide to end it. What questions should you ask the chief data officer, company manager or IT collaborator when interviewing them about a supplier to provide a suitable data system?
Are You Able To Understand Our Problem?
Your business must be able to benefit from the data solution. Your supplier should be able to identify your problem and guide you with the right solution based on the data and customer experience. It should be able to transform big data into intelligent data. Does your provider care? Does your provider care about your professional issues and your target audience? Can they identify the sources of information that can provide you with added value?
Is It Possible To Integrate The Platform With Our Environment?
Your data system should be run in your environment. Data is essential, and investment is necessary. How can you integrate this cloud data management platform into your environment? How can you protect your data?
Are You Using Open-Source Software And The Cloud For Your Software?
Open source and Cloud Computing are the two main trends in IT. It is impossible to create an independent data system that can be used with other applications. Make sure you choose an open system connected to other systems.
What Is The Time It Will Take To Return My Investment?
Significant data software should offer additional value. This surplus value must translate into profit and turnover. Ask your supplier for the numbers that he can provide. What can you do to make your ROI a reality?
Want More Information About Our Services? Talk to Our Consultants!
The conclusion
Big Data is expensive, and so are the solutions. Before you can benefit from Big Data, it is important to understand and familiarize yourself with industry-specific issues. Understanding or familiarizing yourself with the data specificities of each industry. Know where your money goes. Match your skills and services to the market's needs. Big Data can only be exploited effectively if you have a thorough understanding of the vertical industries.
There are many possibilities to explore in the world of data, despite the numerous advantages that cloud-based big data solutions offer. Data analysts are in high demand as organizations look to harness the power of big data. They can help both their firm and themselves.