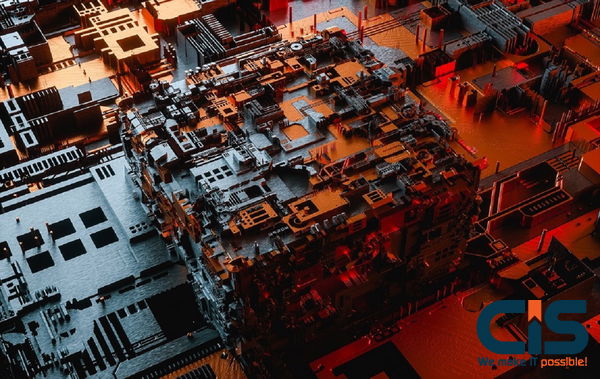
Many different technologies exist now - you may already be familiar with some. At the same time, this article will introduce major categories of data technologies and provide some examples for you to form your own opinion on them.
Every day, consumers generate vast quantities of data. Every time one opens an email, downloads your mobile app, shares you on social media, visits your store, makes an online purchase, speaks to a cloud services customer care representative or inquiries about you, or converses with a virtual assistant, these technologies gather and process this data - not to mention employees, supply chain, marketing and finance teams who also contribute data daily.
Big data refers to large volumes of information from multiple formats and sources. Organizations have realized the benefits of collecting as much data as possible and using big data analysis techniques to convert terabytes of information into meaningful insights.
It refers to any collection of complex data sets or collections with cloud millions of individual pieces that span multiple terabytes or petabytes in size, growing steadily with time. With no end in sight for its rapid expansion, new big data technologies are developing daily that enable this form of analysis; traditional software cannot manage such massive and intricate datasets efficiently; this technology does.
Young people, in particular, are drawn to due to its wide consumer base and long history within the education sphere. You may learn more about big data technology through this article, from learning the appropriate tools and approaches used to the various types of technologies being utilized.
What Is Big Data Analytics?
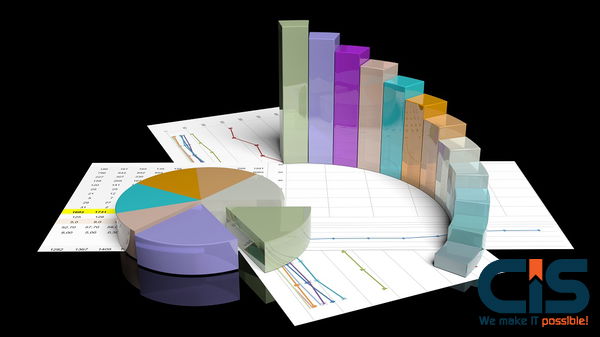
Big data analytics refers to analyzing vast amounts of data for meaningful insights. Modern tools and statistical techniques like clustering and regression are employed when analyzing such large amounts. Big data has become more popular as organizations manage large volumes of unstructured information using software and hardware such as cellphones or Amazon, providing organizations access to much larger amounts of information than before.
Hadoop, Spark, and NoSQL databases were developed as early innovation projects to store and handle massive data volumes, with data engineers always innovating new methods of merging vast amounts of information from smart devices, sensors, networks, transactions, online traffic, or any other source. Big data analysis tools are increasingly integrated with cutting-edge technology like machine learning for greater insight.
Big Data Technology
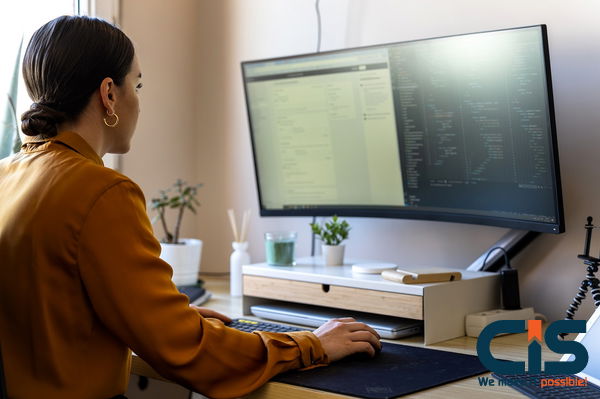
Big data encompasses many technologies that work with large quantities of information - Big Data architecture will amaze you in size and complexity. A collection of tools known as "big data technologies" enable users to efficiently manage and analyze massive amounts of data that are often vast, complex, unstructured, or overwhelming in volume.Traditional tools are optimized to handle small to medium-sized structural data on local machines or servers and work locally. Their four primary goals can help shed more light.
Integration Of Technology
Big data technologies aim to integrate vast quantities of data seamlessly from their source to end users, using different approaches and technologies available today to accomplish this goal easily. Establishing systems and procedures which will facilitate data flow into everyday routines should be central. Much information can be integrated using one or more approaches or technologies available today.
Processors
Big Data technology's integration aspect extends well beyond data processing; processors also play a pivotal role in building infrastructures that help us manage large amounts of information effectively. They use advanced hardware in remote locations and tools designed specifically to integrate it, providing similar experiences as when handling smaller amounts locally.
Storage And Management
Big Data tools and technologies help integrate large amounts of data into everyday work processes while storing it for later retrieval. To do this efficiently and make it accessible when required, these big data tools also play a vital role in their storage management capabilities.
For more details on big data technology solutions that allow users to discover valuable insights that leaders of organizations can use to make important decisions or solve problems. These tools may also be integrated with other big data solutions, so programmers have access to these tools for running statistical analyses, looking for trends, or creating prediction models on large volumes of data - giving consumers access to data they would otherwise miss.
Want More Information About Our Services? Talk to Our Consultants!
What Is Big Data?
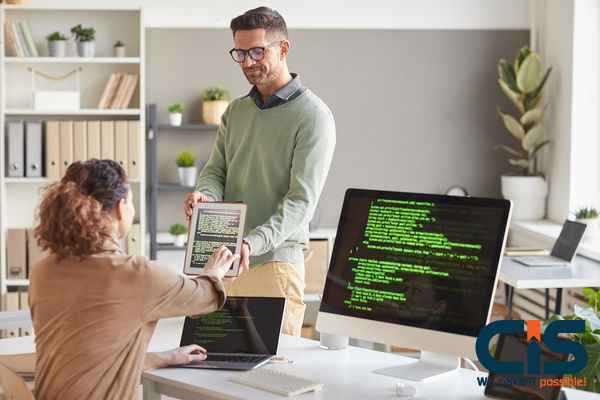
With the rise of big data, technologies have come to an increased demand for data specialists who can tap into its power BI for insights. While mastery may be unattainable at present, understanding what exists is essential for effective analysis.
Big Data Technologies: Types
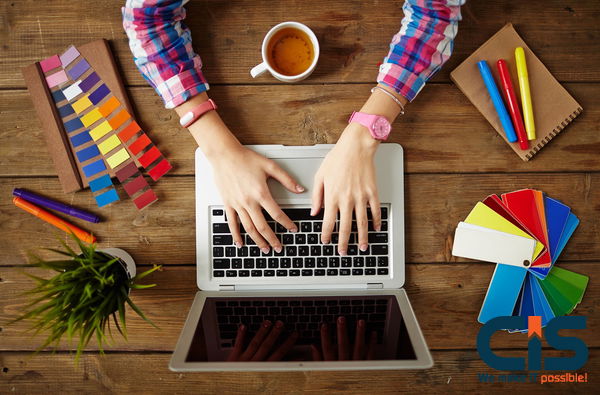
Big Data technology seeks to assist users in storing, managing, integrating, processing, and discovering solutions for their problems by offering methods and technologies known as operational and analytical.
Operational
Within big data technologies, this field brings together engineers and IT specialists. Together they work on processing and storing vast amounts of information.Big data is created and managed through technologies, with efficient storage a key aspect.
However, these solutions need to provide solutions themselves, and the information can come from internal sources, social media platforms, e-commerce sites, and hospitality-based ticket sales sites, among other places.Organizations are ultimately responsible for managing and storing this data. Big data technology arises out of the regular business activities of an organization and provides analytics components with essential raw materials.
Analysis
Analysis for any given study. Scientists and data analysts are needed in this situation. By analyzing large volumes of information using Big Data technology, these professionals use analytics techniques such as stock forecasting, weather forecasting, early illness diagnosis, etc., to find answers to business challenges. Regarding insurance firms, traditional analytics techniques like stock forecasting, weather forecasting, and early illness diagnosis are typically utilized.
Top Big Data Technologies And Techniques
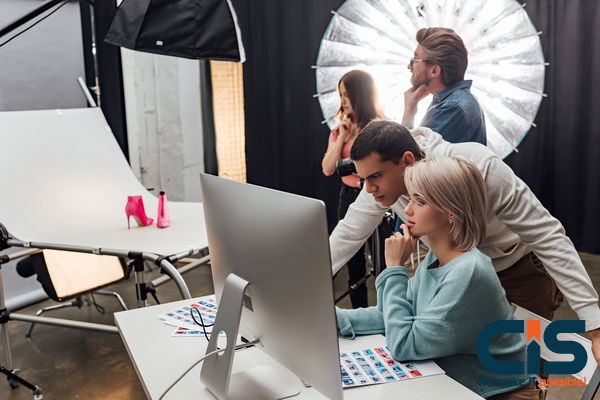
Big data tool usefulness is usually divided into four major areas. These are storage, analytics, mining, and visualization.
Hadoop
One of the most widely-used methods for large data processing, Hadoop uses chunks to organize its information into more manageable units for users to work with. As an underlying Big Data distribution system, Hadoop's ecosystem includes HDFS, YARN, and MapReduce as key elements.
HIVE
HIVE is essential in Big Data tools as it facilitates user-friendly data extraction and writing. HIVE's query language, HQL, is based on SQL. HIVE also features drivers (JDBC Drivers) and its command line (HIVE Command Line), supporting all SQL types.
MongoDB
MongoDB stands out as an indispensable storage solution to store large data sets efficiently and effectively. A NoSQL database like this one differs from conventional RDBMS in that its data structure or schema does not correspond with traditional approaches - making storing massive quantities of information straightforward. Thanks to its numerous distributed designs and fast and flexible storage capacities, this NoSQL solution has become popular.
Apache Sqoop
Mes With Apache Sqoop, users can move massive data sets from Apache Hadoop into structured databases like MYSQL or Oracle without disrupting Hadoop itself - or vice versa! There are connections available for every popular RDBMS in this tool.
Data Lakes
Data Lakes can be used to store all kinds of unstructured data. As their name implies, Data Lakes are ideal for this.
Cassandra
Another powerful NoSQL database tool, Cassandra, is capable of handling dispersed data sets distributed over multiple clusters. Due to its excellent scalability and query-based functionality, Cassandra is often chosen over similar offerings as the NoSQL data store.
Information Analysis Utilizing Big Data Tools
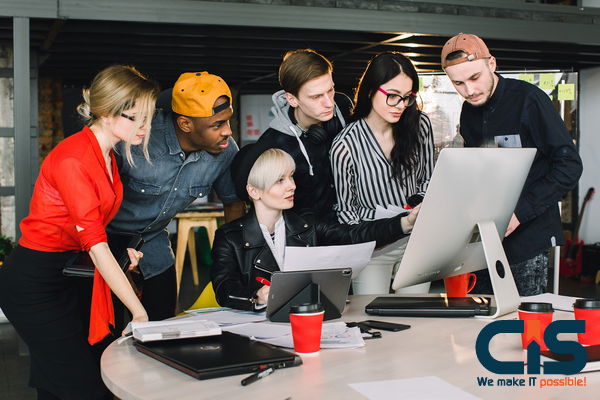
Apache Spark
Apache Spark enables users to quickly perform tasks such as batch processing, interactive and iterative processing, visualization, manipulation, and other related activities, using only RAM as opposed to older technologies like Hadoop, which uses more space-consuming storage media for the real-time processing of Big data tools.
R Language Features And Syntax Arrangement
R is widely known as the statisticians' language. R facilitates the creation and execution of statistical tests for scientists and data analysts. R can also assist with the creation of the Deep Learning/ Machine Learning model; our list now includes it due to its integration with big data technologies.
Qlik Sense
Users can quickly and effortlessly construct narrative reports using Qlik Sense's user interface, similar to QlikView, making the creation of narrative reports quickly and effortlessly.
Hunk
Data from the Hadoop Ecosystem can be quickly and visually analyzed using Hunk, an open-source big data tool designed for rapid deployment with an extensive developer's environment, customer dashboards, drag-and-drop analytics capabilities, rapid deployment speeds, and fast installation times, all made available by this big data solution. Hunk also leverages Splunk Search Processing Language when processing its analysis results.
Platform
This big data analytics platform costs money each month, but users may use this interactive tool to spot early patterns in raw data quickly.
Kafka
Enterprise Messaging System and Kafka, a stream processing framework, are strikingly similar. Both interact with multiple formats simultaneously while publishing massive amounts of data live - ideal for real-time delivery, processing, and transmission of information.
Presto Electric
Presto is widely employed for massive data analysis. Interactive Analytics Queries are executed via its Distributed SQL Query Engine; Hive, Cassandra, and MySQL all support its use; it enables user-defined function creation and pipeline execution with greater ease; code debugging becomes simpler; scaling massive amounts of data quickly is its greatest strength - which explains why businesses such as Facebook employ Presto for this reason alone.
KNIME
Mes This Java-based big data app utilizes powerful data mining algorithms to assist users with data analysis and processing while connecting to Hadoop using machine learning techniques via MLlib integration.
Mahout For Machine Learning
Mahout is a machine learning-based app which performs segmentation and classification functions.
Read More: Utilizing Big Data to Enhance Technology Services
Data Mining-Based Big Data Tools
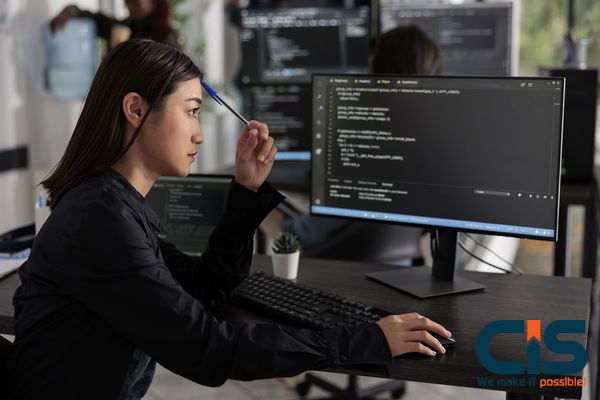
MapReduce
By employing parallel algorithms that employ logic in parallel, MapReduce can efficiently process the huge amount of information generated by Big Data. MapReduce also transforms otherwise unintelligible data into something easily manageable - hence its name "MapReduce," composed from "Map" and "Reduce," refers to this approach for merging and summarizing it all together.
Apache PIG
Developed by Yahoo to develop PIG, resembles SQL syntax regarding data processing, analysis, and organization. They are used mainly to manage massive volumes of data quickly while offering custom functions written by users that will be automatically transformed into MapReduce programs in the background.
RapidMiner
RapidMiner can be used for predictive modeling, machine learning, and ETL purposes - among many others - thanks to its user-friendly interface, ability to address business analytics challenges effectively, and support for multiple languages. RapidMiner's main benefit lies in being open-sourced and still viewed as secure while being so available.
Apache Storm
Apache Storm is an open-source real-time computing framework designed for distributed real-time processing using Clojure and Java to manage unbounded data streams.
Flink
Flink can handle limited and unbounded data streams, much like batch processing, except with no start and end dates. As it's a highly scalable distributed processing engine, data analytics pipelines may take advantage of Flink as a distributed processing engine.
Elastic Search, a widely utilized Java-based search engine used by several businesses, including Accenture, StackOverflow, and Netflix, is well known. It offers an HTTP Web interface and Schema-free JSON documents, which provides an effortless experience for customers.
Data Visualization-Based Big Data Tools
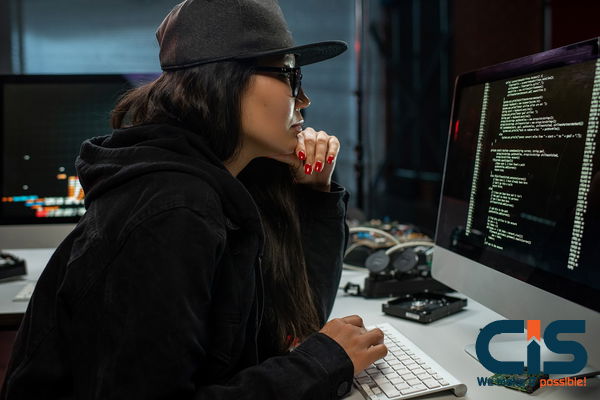
Tableau
Spreadsheets, big data platforms, and cloud-based databases can all easily connect with Tableau's powerful tool for data visualization: Tableau. It delivers immediate insights from unprocessed data while being a complete, secure application that supports real-time dashboard sharing - making it highly sought after among businesses.
Plotly
Users can create interactive dashboards with Plotly. Python, R, MATLAB, and Julia support its API library; Plotly Dash offers interactive graph creation in Python.
Big Data Technologies And Tools Are Evolving
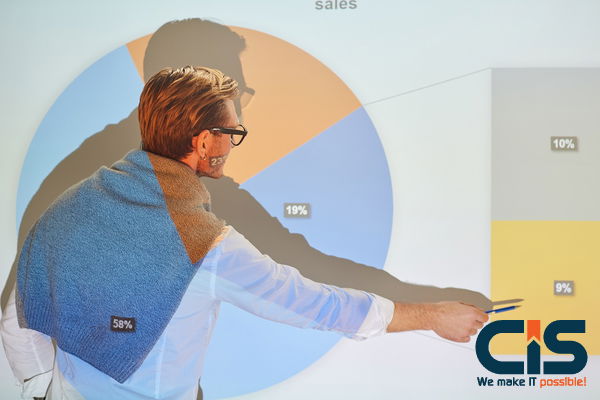
Big data's latest innovations are expected to gain in popularity, and data scientists should remain abreast of them if they wish to pursue careers in big data research. We discussed essential tools in an earlier blog post; here are additional ones you should be familiar with.
TensorFlow
TensorFlow is an expansive ecosystem consisting of modules and resources. As a tool for Machine Learning and Deep Learning Model creation on big data sets, TensorFlow allows for ease of use when it comes to Big Data models.
Apache Beam
Apache Beam can create parallel data processing pipelines quickly, simplifying large-scale processing operations by writing pipeline programs in Java, Python, or Go.
Kubernetes
Kubernetes is a big data tool designed to automate the deployment and scaling of containerized applications across clusters while providing visibility into these apps in the cloud computing.
Blockchain
The Big Data Technology that creates encrypted data blocks linked together for security transactions like Bitcoin transactions. Blockchain holds huge promise in Big Data applications; BFSI industries have acknowledged its benefits.
Airflow
Apache Airflow can assist in scheduling and managing pipelines effectively. This application assists with organizing complex data pipelines from varying sources using Directed Acyclic Graph models; airflow provides this service, improving the precision of machine learning (ML) models.
What Benefits Does Big Data Technology Provide?
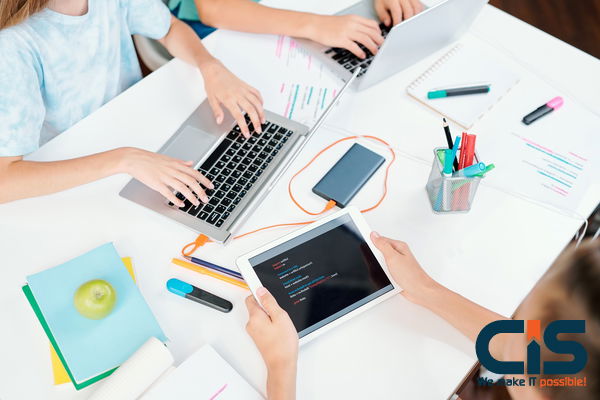
Implementing a central pricing system offers numerous advantages. Some of the key ones include reduced costs, enhanced operational effectiveness, and promotion of competitive prices. Predictive analytics is one of the best tools businesses have available to mitigate risk when making decisions. By processing large volumes of data, predictive analytics software and hardware solutions allow businesses to discover scenarios, evaluate them, prepare for future events, and identify problems through deep analyses and understandings.
These databases offer reliable data management across a range of storage devices. NoSQL uses relational databases, JSON documents, and key-value pairs as storage options to hold information securely. Companies can leverage technology to access big data from various structured and unstructured sources, including file systems or APIs, for example. Using search and knowledge discovery tools, companies can use this data effectively for their own advantage.
Stream Analytics
A company must manage large volumes of data stored across various formats and platforms. Filtering, collecting, and analyzing massive volumes requires stream analytics software which enables application flows to incorporate other sources of information.
In-Memory Data Fabric
Large data volumes may be distributed with this technique over a variety of system components, including Flash Storage, Dynamic RAM, and Solid State Storage Drives, resulting in low-latency processing and access across nodes for big data services.
Distributed Storage
Distributed file storage with replicated data can protect from independent node failures, data loss, and corruption from large data sources. For low-latency access across wide networks, copies may occasionally be made. Typically these non-relational database systems use data compression methods.
Data Virtualization
Thanks to Data Virtualization, applications may access real-time or near real-time data regardless of its type, location, or format in real-time or almost real-time regardless of its source or format.One of the most well-known big data technologies is data virtualization, which is employed by Apache Hadoop or other distributed data stores to offer real-time and nearly real-time access across platforms.
Integrating Data
Effectively utilizing and interpreting large volumes of data is often the biggest challenge when dealing with big data. Integrating solutions like Hadoop MapReduce, Apache Spark, Amazon EMR (Amazon Elastic Compute google Cloud storage), Apache Hive (Apache Pig), and Couchbase may provide solutions.
Preprocessing Data
The software can transform raw data into an easily usable format that can be utilized for further analyses. Data preparation tools facilitate faster sharing by structuring and cleaning unstructured datasets quickly. Unfortunately, data preprocessing cannot be fully automated - thus necessitating human intervention at certain points, which is time-consuming and laborious.
Data Quality
Data quality is an integral aspect of large data processing, enabling large datasets to be cleaned and enhanced through parallel processing using data quality tools. Massive data processing uses these tools to deliver reliable results that provide consistent and dependable solutions.
Want More Information About Our Services? Talk to Our Consultants!
Conclusion
Its Big data has become an invaluable asset to businesses to solve daily issues and make key strategic business decisions. To become proficient with big data technology, mastery requires understanding its fundamentals - learning more about its components will give you an incredible learning experience. It would be best to start by exploring more big data tools - you are in for an incredible adventure.
Big data analytics encompasses various approaches and procedures, making them especially powerful when applied collectively by firms to produce meaningful results in strategy management and execution.
Although investors have expressed great enthusiasm for using data as a transformative force in enterprise transformations, results vary widely. Organizations still struggle to create an "information culture," with only 40.2% of executives reporting success implementing such programs; big transformations typically require considerable time to achieve their goals, and culture transformation rarely happens overnight.
Businesses implementing big data often need help beyond technological implementation; most of their challenges stem from cultural aspects: lack of understanding, organizational alignment issues, and change management issues. Big Data is becoming an indispensable technology.
Big Data has already proven its use by increasing operational efficiency and making decisions with up-to-date information, becoming an industry standard in no time. Big Data will become an invaluable technology in many sectors shortly. Businesses benefit immensely from adopting Big Data technologies; to get maximum value out of it for themselves and their workers, they should learn to manage Big Data to increase productivity and efficiency at work.