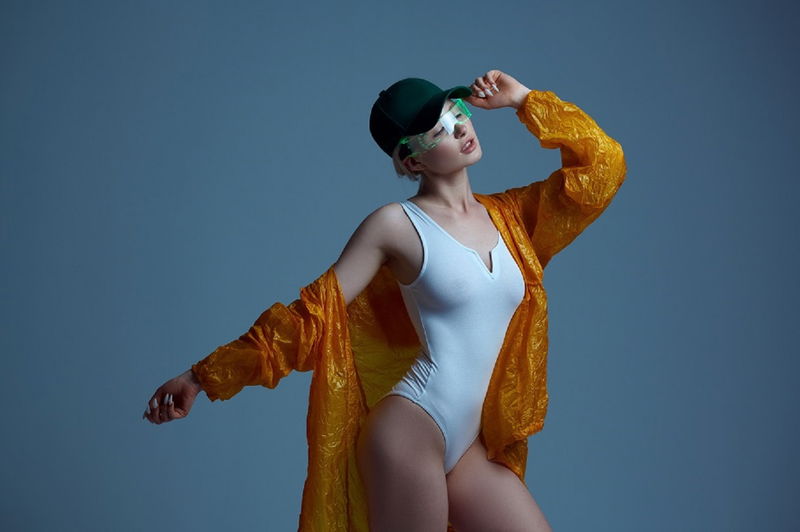
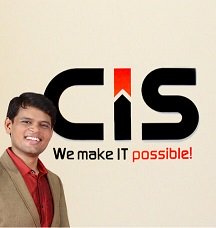
Contact us anytime to know more — Amit A., Founder & COO CISIN
AI can process large amounts of data quickly and accurately and even execute complex algorithms. AI is becoming more intelligent every year and improving business efficiency.
Artificial Intelligence (AI) is the next big thing in this high-tech industry. Innovation and research conducted by top tech companies profoundly impact industries such as manufacturing, finance, healthcare, and auto.
Technology has always been an important component of all domains, but AI puts technology at the heart of every company. AI will be integrated into every program or apparatus, from life-saving medical equipment to self-driving cars.
Amazon, Microsoft, and platforms like Google, and IBM, are investing in AI research and development. They are working to make AI more accessible for businesses.
AI has been used in various ways, including medical research and diagnostics. AI can access thousands upon thousands of diagnostic resources and match doctors' clinical knowledge.
AI may eventually decrease the need for human doctors. Recent research and data show that AI can improve clinical diagnosis and decision-making. The field is still in its infancy.
The future of healthcare will only be known when AI is fully developed.
It will be determined whether AI is effective in human medicine by how it is used in healthcare. It must first be approved by regulatory agencies, integrated into the EHR system, and trained for clinicians.
The second step is to develop and update the systems in the field. This will take longer than the actual technology. It is still in its early stages, so it is not surprising that AI will take longer to be used in the field than doctors.
Three Essential Aspects Will Accelerate Innovation In Machine Learning and Artificial Intelligence
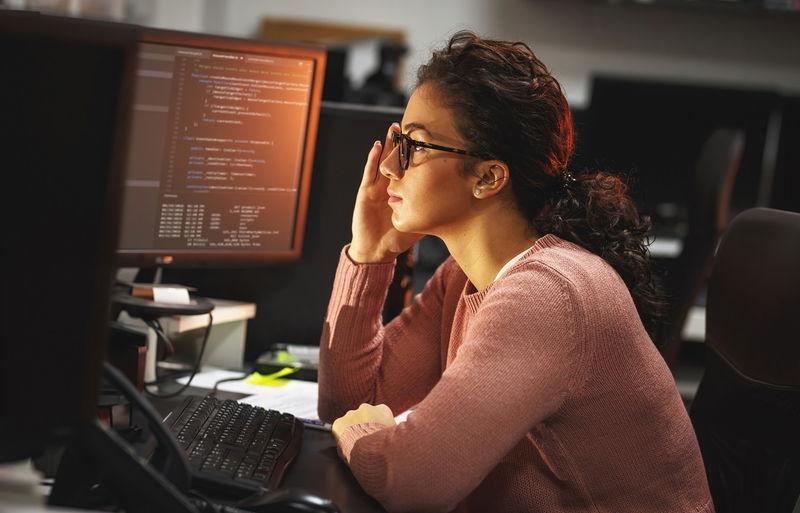
Advanced computing architecture
Machine Learning is not something that conventional CPUs and microprocessors can do. There may be better choices than a fast CPU training a complex ML version.
A new breed of chips is needed to complement CPUs to coach and infer ML versions that provide intelligence for software.
Graphics Processing Units (GPU) are in high demand due to the rise of AI.
The GPU is now a highly sought-after processor in the cloud. GPUs come with tens to thousands of cores which speed up the ML training process, contrary to CPUs. GPUs are becoming essential for any type of inferencing.
A GPU will likely be present wherever a CPU is in the future. GPUs will be key to AI, whether on customer devices or virtual machines in the cloud.
Conventional CPUs are designed for general-purpose computing. FPGA devices can be used for market computing tasks such as training ML versions.
Public cloud providers are using FPGAs to offer highly optimized, optimized infrastructure for AI.
The cloud's access to bare-metal servers allows scientists and researchers to perform high-performance computing.
These dedicated, single-tenant servers offer best-in-class functionality. This multi-tenant infrastructure makes virtual machines susceptible to noisy neighbors—Cloud infrastructure providers like Amazon EC2 and IBM Cloud supply bare metal servers.
These inventions will encourage the adoption of AI across a range of areas, including Aerospace, Health, Picture Processing, Manufacturing, and Automotive.
Deep Neural Networks: Progress
The progress in deep learning and artificial neural network research is the next and most important element of AI research.
Artificial Neural Networks (ANNs) replace conventional Machine Learning versions to develop precise and accurate versions.
Convolutional Neural Networks, or CNN, provide deep learning for vision monitoring. Image processing is one of the most important advances in computer vision, such as the Single Shot Multibox Detectors (SSD) or Generative Adversarial Networks (GAN).
For example, images taken in low light or very low resolution can be HD by using a few techniques. Computer vision research is the basis for picture processing in transport, health care, and other domains.
These new ML methods, such as Capsule Neural Networks or CapsNet, will fundamentally change how ML versions are trained and deployed.
They can forecast with precision even when they are trained using restricted data.
Access to historical data sets
Before Cloud was mainstream, it was expensive to save and access information. Cloud-based companies have enabled academia and authorities to access previously only available information on magnetic disks and cassette cartridges.
Data scientists need access to large historical data sets to help them build ML models that can predict with greater accuracy.
The dataset's quality and size directly determine an ML model's efficacy. Researchers need large datasets that cover various data issues to address complex problems such as calling rain or discovering cancer.
Government agencies, hospitals, and other institutions are making unstructured data available to researchers as information storage and recovery become more affordable.
Researchers now have access to rich datasets, from historic rainfall trends to clinical imaging. This variable alone has a significant impact on AI research.
Combining high-performance computing equipment with much data will produce next-generation AI options.
Microsoft, Google, IBM, and Google support AI research. They are investing billions in AI research across diverse business verticals.
Researchers and information scientists can innovate quickly with the help of rich datasets and next-generation computing architectures.
These variables will make AI an integral part of devices and applications.
Want More Information About Our Services? Talk to Our Consultants!
Explore Recent Developments in Artificial Intelligence
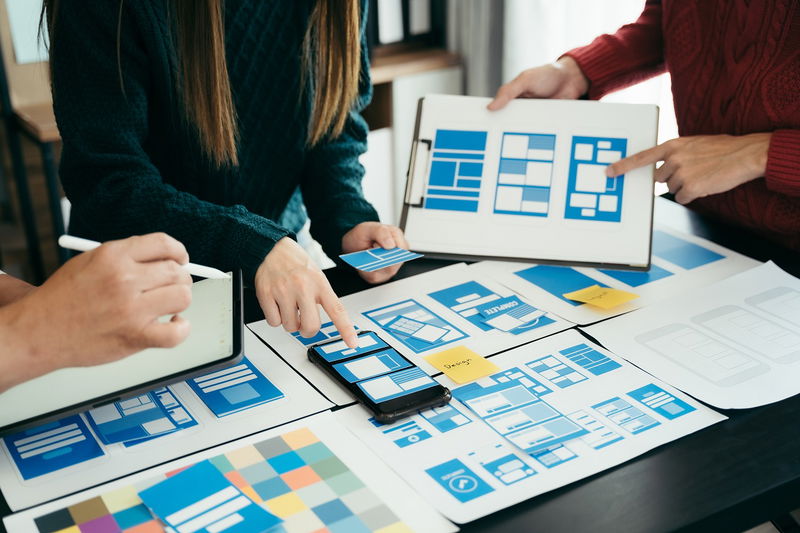
These are the most recent development of ai that you need to know.
Artificial Intelligence Trends In Robotics
Robotics startups are now using AI to automate and develop new AI systems. Robotics is a pioneer in innovation using plug-and-play controls that are equipped with AI-powered software.
It has stated that the firm's proprietary software and controllers allow it to operate tethered robotics on ocean floors.
They can also use robotics for satellite service using high-latency satellite links and to clean up hazardous chemical spillages using robotics that relies upon 4G and 5G networks.
These breakthroughs rapidly expand robotics' role and significantly contribute to humanity's collective progress.
The advancement of robotic natural language processing is being influenced by artificial intelligence, including its history and current developments.
The adoption of advanced NLP has been accelerated by the release of Google’s BERT model. This NLP allows language to be identified and understood in a more complex and machine-computable way.
Google's model can be downloaded openly and freely available for public use.
Machine Learning for Nature Conservation
Oxford University used the latest artificial intelligence developments to create new software to identify endangered species, such as chimpanzees, and trace their faces in their natural habitats.
This software assists researchers and conservationists in maximizing their impact. The software reduces the time and resources required to track and observe at-risk species.
The team plans to use artificial intelligence trends software to positively impact conservation efforts for endangered primates and other species.
AI Advancements in Healthcare
Artificial intelligence is the latest advancement in healthcare. It allows for better diagnosis, clinical decision-making, and patient care.
AI research drives the widespread adoption of Telemedicine at Healthcare institutions. Wearable devices incorporate it into their apps, which offer useful features such as screen sharing, video chat, and texting.
Patients can use these apps to book appointments, follow-ups, and request prescriptions.
There are many challenges that diagnostic AI faces, but they are being steadily overcome. It has been difficult for AI for a long time to interpret diagnostic results while under human supervision accurately.
Due to image shortages, it is difficult for machines to recognize rare diseases. AI technology has allowed computer-generated Xrays to augment AI training and create a larger database.
This database allows neural networks to be trained to recognize rare conditions.
Recent advances in artificial intelligence have made it possible to produce COVID vaccinations.
This allows for the development of more effective vaccines with fewer side effects. This increases the willingness of patients to get vaccinated.
AI Developments in the Automobile Sector
Autonomous self-driving cars are widely considered the next step in artificial intelligence and the future of transportation.
Autonomous vehicles powered by AI are rapidly gaining popularity.
In the future, cars will be able to connect with each other and transport infrastructure via AI. Augmented reality will allow vehicle occupants to connect with the outside world via multi-media services.
According to Analytics Insight, industry analysts have estimated that more than 250 million vehicles will be connected to the internet by 2022-23.
These vehicles are equipped with Internet of Things-paired sensors, geo-analytical capabilities, and connectivity platforms for Big Data. This is the beginning of a shift towards a more unified platform for vehicle operations.
The US Department believes self-driving cars will replace e-commerce or business road freight. Road freight is currently one of the biggest carbon emitters in the world.
This will allow future vehicles to consume less fuel and increase safety and efficiency.
These artificial intelligence trends can be used to reduce the rising CO2 levels. These levels are causing climate change and potentially threatening the stability of the world's natural ecosystems.
Global transportation currently contributes 15% to 20% of total global carbon emissions.
Read More: The Impact Of Artificial Intelligence (AI) In Mobile Applications
Computer Vision and Video Analysis Technology Artificial Intelligence Trends
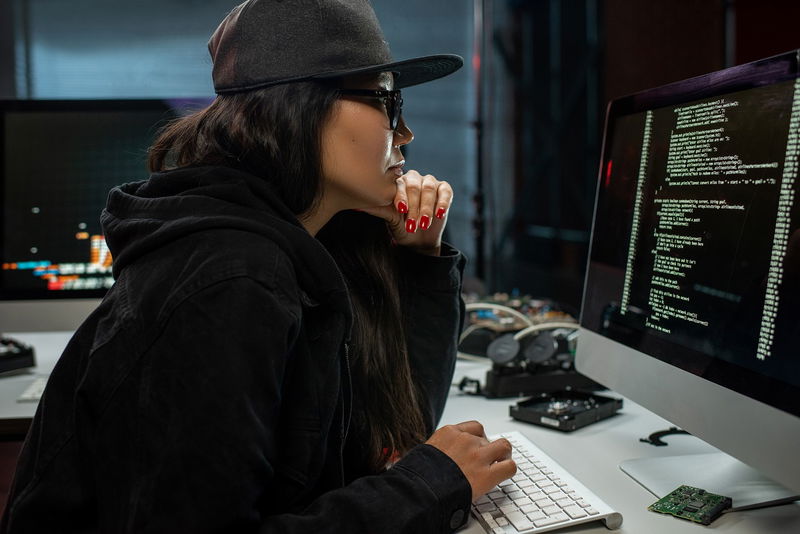
Deep learning and other AI innovations have made image and video analysis tools easier and more affordable than ever.
These computer vision tools are important in the medical and manufacturing sectors.
Radiology uses Artificial Intelligence development tools to increase the efficiency of diagnostic procedures.
This allows doctors to provide more precise and faster diagnoses.
To fuel Industry 4.0, accessible computer vision AI technology is used in manufacturing. It is revolutionizing safety management, supply chain management, and quality control.
All professionals in all industries would benefit from monitoring future computer visual trends.
AI Advances Towards High-Level Human Functions
Using tools to accomplish certain goals and objectives is a long-held human habit. Except for a few animal species like corvids and chimpanzees, this is only true of humans.
OpenAI recently developed new AI agents that learned to use tools in an experiment using multi-agent competition.
This was without having to be taught. This is a significant step forward in developing and applying autonomous, valuable artificial intelligence trends.
Cyber Infrastructure Inc. and other companies have shown robots that can perform tasks in real life by learning from human actions.
Robots can observe how tasks are performed and then re-enact them. This is a significant improvement over how robots normally behave.
AlphaGo, another bot program, has learned advanced strategies to play the game of 'Go' without human training. It is possible that artificial intelligence will lead to robots that can operate independently of humans and human knowledge.
Robotic Assistant Development
If you were to fall ill, would you feel comfortable having a robot nurse care for you? What would you think about a robot caregiver caring for your elderly relatives or parents? A robot retail assistant to help you choose a new pair of jeans.
Recent AI advancements could help bridge this gap. Japan's government is working to promote the acceptance of technology that could replace human caregivers and nurses.
Japanese engineers are developing robots that will assist people in getting out of bed, predicting when they might need to go to the bathroom, and managing their medication.
Future priorities for the researchers include the development of wearable mobility aids, technology that can direct people to the bathroom and bed when AI predicts it is the right time.
Artificial Intelligence and Cybersecurity
Cybersecurity is a current topic because businesses, remote workers, and major brands are all moving their operations online.
With the rapid advancement of technology and recent developments in AI, there have been growing threats to sensitive data networks and networks.
To provide greater cybersecurity, many companies have created intuitive AI solutions. This cybersecurity enhances incident detection and response, identifies, communicates, and maintains optimal control over the situation.
Magnifier, a Palo Alto Networks behavioral analytics solution, is one of the latest developments in this area. Machine learning is used to improve threat detection and model network behavior.
This AI development solution uses machine learning to improve network behavior.
Alphabet, Google's parent company, introduced the cybersecurity intelligence platform Chronicle to improve and streamline cybersecurity measures.
More Intelligent Smartphone Apps
AI-based custom code is now available in many smartphone and tablet apps that are designed for everyday use. According to a Garner report, it will have around 80% of smartphones with embedded AI capabilities.
This compares to the 10% of smartphones with these capabilities.
Currently, three apps use AI to deliver their services: Google Assistant, Microsoft Pix, and Socratic. Google Assistant lets users check their appointments, search the internet, listen to music and send messages hands-free.
Socratic, an AI-based tutoring application that helps you solve math problems by analyzing images of equations, is called Socratic.
Microsoft Pix is another machine-learning tool that uses AI to choose the best three images from ten frames. The app automatically deletes all other shots to optimize users' photography skills and reduce storage space.
To maximize AI benefits, there are nine steps we recommend:
-
Encourage researchers to have greater data access without compromising users' privacy.
-
Increase government funding for unclassified AI research.
-
Encourage new digital education and AI workforce development models to ensure employees are equipped with the necessary skills for the 21st-century economy.
-
To make policy recommendations, create an AI advisory panel at the federal level.
-
Engage with local and state officials to ensure that they implement effective policies.
-
Regulate AI principles and not specific algorithms.
-
AI should not be used to replicate historical injustices, unfairness, or discrimination.
-
Maintain mechanisms for human oversight.
-
Cybersecurity is promoted and punished for AI-related crimes.
Qualities Of Artificial Intelligence- Researchers believe that AI refers to machines that respond to stimulation like traditional human responses.
They "make decisions which normally require human level expertise" and can help people deal with problems as they arise. They are intelligent, adaptive, and intentional.
Intentionality
Artificial intelligence algorithms are used to make decisions. They often use real-time data. They are not passive machines that can only provide predetermined or mechanical responses.
They combine data from many sources and instantly analyze it. They can achieve incredible sophistication in analysis, decision-making, and processing speed thanks to massive improvements in storage and analytic methods.
Intelligence
Machine learning is often used in combination with data analytics and machine learning. Software designers can use that information to identify specific problems if it finds something relevant.
Data that can be used to identify patterns is all that is needed. Data can be in the form of digital information, satellite imagery, text, and unstructured data.
Adaptability
AI systems can adapt and learn as they make decisions. Semi-autonomous vehicles can use tools to inform drivers and vehicles about traffic conditions, such as potholes, road construction, or congestion.
Vehicles can benefit from the experience of other vehicles, which is done without the need for human intervention. The entire corpus of their "experience" can be immediately transferred to other similar-equipped vehicles. The advanced algorithms, sensors, and cameras combine experience in current operations.
They use dashboards and visual displays that present real-time information to allow human drivers to understand traffic and other vehicular conditions. Advanced systems can also control fully autonomous vehicles and make navigational decisions.
Applications To Diverse Sectors

AI is not something futuristic. It is a technology already in use and integrated into many sectors. These include finance, security, healthcare, criminal justice, and transportation. Many examples of AI are already impacting the world, augmenting human abilities in important ways.
The growth of artificial intelligence is increasing rapidly.
Want More Information About Our Services? Talk to Our Consultants!
Wrap up
AI is more important than ever. High-tech smart speakers and coffee makers are more common with the advent of the Internet of Things.
Artificial intelligence is also rapidly growing. The AI job market is growing as more industries are dependent on AI. AI professionals have bright prospects. The field's growth can only be expected to continue.
The rapid rise of artificial intelligence is creating new exciting opportunities in various sectors and industries.
Artificial intelligence is already disrupting many industries, and it's expected to change many more over the next few years.
Both public and private companies realize that artificial intelligence can significantly impact virtually all aspects of our lives, provided Cyber Infastructure Inc.
have the right structure and guidance.