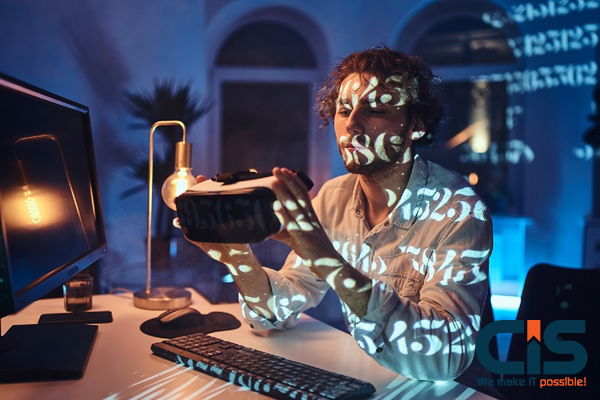
Earlier, thieves had to fake client IDs. Now they only need the password to a person's account to steal money. Both digital and physical environments have an impact on customer loyalty and conversion. A study found that brick-and-mortar financial institutions take 40 days or more to detect fraud. Fraud affects banks that offer online payment services. As an example, 20 percent change banks after scams.
Why Use Machine Learning For Fraud Prevention?
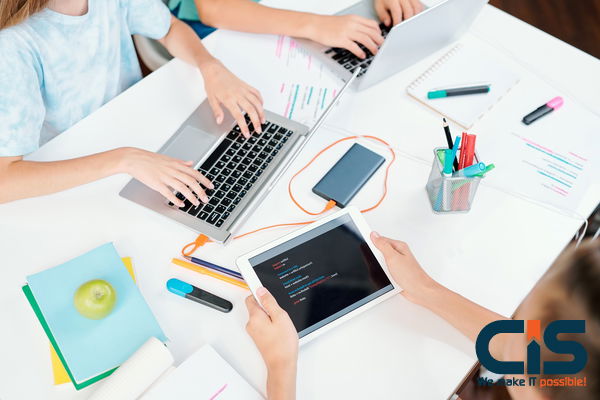
Machine learning is a powerful tool for financial fraud detection, as the above examples show. Machine learning algorithms are ideally suited to the high volume of consumer and transactional data. ML allows banks and financial institutions in real-time to detect and flag fraud.
A Machine learning algorithm is more accurate, which means that financial firms can reduce the number of false negatives and false positives. It is for this reason that ML has become the leading technology in the financial sector. You can read the benefits listed below if you are still unsure about how machine learning will help detect fraud in your financial services company:
- Data Collection Is Faster: With the increasing speed of commerce, it's essential to have solutions that are quicker to detect fraud. Machine learning can help. Machine learning algorithms are able to evaluate huge amounts of data within a short period of time. They can continuously collect data and analyze it in real time and detect fraud within seconds.
- Machine Learning Models And Algorithms Are More Effective As Data Sets Increase: The machine's learning is improved with more data because the ML models can identify similarities and differences in multiple behaviors. The system will then begin to filter out the fraudulent transactions and only keep the genuine ones.
- Machines Are More Efficient Than Humans At Performing Repetitive Tasks And Detecting Changes In Large Volumes Of Data: This is crucial for fraud detection within a shorter time frame. Algorithms are capable of accurately analyzing hundreds of thousands of payments every second. It reduces the time and costs required to analyze transactions.
- Security Breaches Are Reduced: By implementing machine learning systems, banks can reduce fraud and offer the highest level of security to their clients. It compares every new transaction to the previous one (personal data, IP addresses, location, etc.) and detects suspicious cases. Financial units can then prevent fraud involving payment cards or credit cards.
Let's look at the machine learning models that are used to detect fraud now that we know the benefits.
What Is The Role Of ML In Fraud Detection?
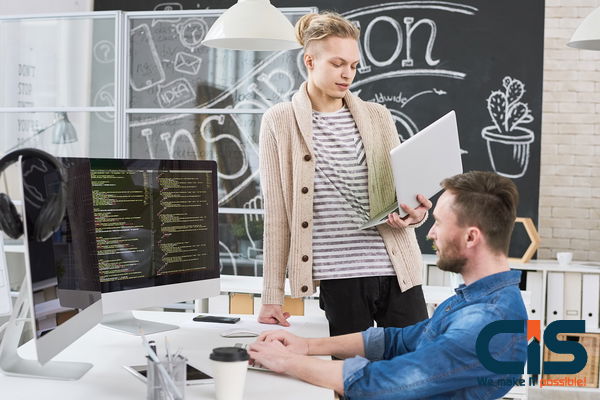
Machine learning systems are used to segment data and begin the fraud detection process. After that, the machine-learning model is fed with algorithms for training to predict fraud probabilities. Here are some steps to show you how an ML system can be used for fraud detection:
- Data Input: Before a machine-learning system can detect fraud, it must first collect data. The more data a machine learning model has, the better able it is to learn and improve its fraud detection skills.
- Next, We Will Extract Features: This stage adds features that describe both positive and fraudulent customer behavior. These features include:
- Identity: This includes the fraud rate, account age, and number of devices that were used to view customers.
- Order: The order feature displays the number of orders, average order value, and number of failed transactions.
- Location: The feature allows you to check if your shipping address is the same as the billing address. It also checks if the country where the shipping address is located matches that of the IP address.
- Payment Methods: It is used to determine the fraud rate in banks that issue credit/debit cards and the similarity of the billing name with the customer's name.
- The Network: This includes the number of emails, phone numbers, and payment methods that are shared in a given network.
- Train Algorithm: A set of rules is used by a Machine Learning algorithm to determine whether a particular operation is legitimate or fraudulent. The more data you can provide to a training set for an ML model, the better it will perform.
- After The Training, Your Company Will Have A Machine-Learning Model For Fraud Detection: This model is able to detect fraud with great accuracy in a short time. Machine learning models must be updated and enhanced often if they are to be successful at detecting fraud.
Fraud Detection: Machine Learning Vs. Rule-Based Systems
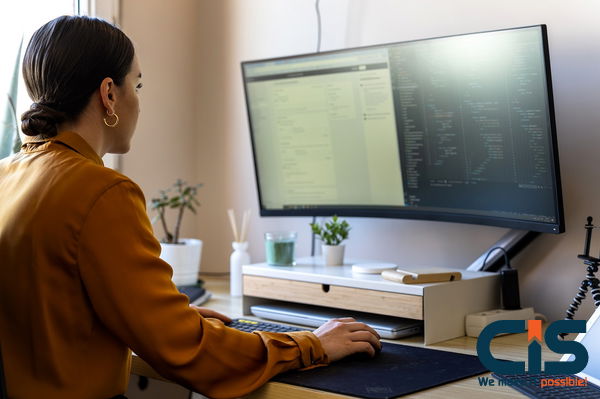
In recent years, the machine learning (ML) approach to fraud prevention has gained a lot more attention. This has shifted the industry focus from rule-based systems to ML solutions. What are the differences between machine learning versus rule-based fraud detection?
The rule-based method. On-surface and obvious signals can help detect fraudulent activities in the finance sector. Large transactions and those that occur in unusual locations or at untypical times require additional verification. Purely rule-based systems use algorithms to perform multiple fraud detection scenarios. These algorithms are manually written by fraud analysts.
Legacy systems today apply an average of 300 rules to approve a single transaction. Rule-based systems are too simple. The systems require manual scenarios and adjustments, but they can't detect implicit correlations. Rule-based systems are often based on legacy software, which is unable to process real-time data streams.
Fraud detection using ML. There are subtle events that occur in the user's behavior, which may not be obvious but can still indicate fraud. Machine learning can create algorithms to process large datasets containing many variables and find hidden correlations that are present between user behavior and fraud likelihood. Machine learning systems are also faster at processing data and require less manual labor than rule-based systems. Smart algorithms, for example, work well with behavior analytics to reduce the number of verification steps.
Although rule-based systems may be inferior to ML, they still have a dominant position in the market. Leading financial institutions have already started employing ML technology to fight fraud. It also tracked and processed purchase data and device information. The technology determines if a transaction is fraudulent in real time by analyzing the account activity throughout each transaction.
Fraud Detection Through Insurance Claims Analysis
Scams still affect the insurance industry, even though companies take several days or weeks to assess a claim. Property damage, auto insurance scams, and fake unemployment are the most common problems. The secrets to successful detection are a good dataset and well selected models.
False Claims
A semantic analysis task is a machine-learning task that analyzes both structured data in table form and unstructured text. This feature is used to detect false and fake claims in the insurance sector. It can improve the processing of car insurance claims, for example. The algorithms used by machine learning analyze documents written by clients, insurance agents, and police to search for any inconsistencies.
These textual datasets contain many clues. Rule-based engines can't detect suspicious correlations between textual data, and fraud analysts may miss important evidence hidden in boring investigation files. This is why machine learning applications are most likely to be successful in analyzing claims.
Overstating Repair Costs And Duplicate Claims
Smart Machine Learning algorithms are efficient in detecting duplicate claims or inconsistencies with car repair costs. The problem is solved by classifying data from repair claims. This reveals hidden correlations between claim records or even the behaviors of agents, repair services, and clients. The repair service provider may offer higher prices to customers who are represented by a particular agent.
Here are the results of an AI-based solution study of claims for insurance vehicles. The company analyzed four datasets that included features such as vehicle type, client gender, and marital status. They also looked at license type, injury types, date of loss, date of claim, date of police notification, repair amount, total insured value, and market value.
The results of a pre-research study revealed the following:
- Police are less likely to report fraudulent claims.
- Fraud involving older vehicles is more common.
- Fraud is responsible for 80 percent of all accidents that occur during holidays.
- Frauds that involve third parties are more likely than those that involve legitimate claims to be scams.
Five different Machine learning algorithms were used to process the data: Logistic regression, Modified Multivariate Gaussian (MMG), Modified Randomized undersampling (MRUS), Adjusted Minority oversampling (AMO), and Adjusted Random Forest. Modified Randomized Undersampling was the model that produced the best results, with a 79 percent accuracy.
Fraud Prevention In eCommerce
Payments are a key component of the eCommerce scam. Let's talk about the eCommerce aspects. Identity theft and scams involving merchants are typical scams. Identity theft. Scammers will hack into a user's account, change personal data and then try to obtain money or products from retailers using this semi-fake information. Behavior analytics solves nearly all fraud detection tasks. Smart algorithms detect suspicious activity, analyze it, and then find inconsistencies within historical data sets.
Scams Of Merchants
They are usually related to fraudulent companies or merchants who operate through marketplaces. Reviews are a major factor in influencing the choices of customers on marketplaces. Fraudsters may create fake reviews to lure customers. By analyzing sentiment and behavior and detecting suspicious activity linked to merchants and their products, machine-learning algorithms can reduce the influence of fraudsters.
Fraud Detection For Banking And Credit Card Transactions
The payments industry and banking sector is the most digitized in the financial sector, making it particularly vulnerable to a wide range of cyber fraud. Mobile payments are on the rise, and banks compete to provide the best possible customer experience. This has led them to reduce the number of stages of verification. The rule-based method is less efficient. Banks and payment companies now use data analytics, AI, machine learning, and other AI-driven methods.
Modern fraud detection systems are able to solve a variety of analytical problems in order to detect all scams within the payment streams. Data credibility assessment. Machine learning algorithms are able to reconcile system data and paper documents, eliminating the need for human intervention. This will ensure data credibility by identifying gaps and verifying details through public sources and transaction history.
Copying Transactions
This is a common scam. It involves creating transactions that are similar to the original or copying a transaction. A company may try to charge the same counterpart twice by sending the invoice to two different branches. Currently, rule-based systems are unable to differentiate between fraud and error. A customer may accidentally press a button twice or decide to purchase twice as many goods. The system must distinguish between human error and suspicious duplicates.
Machine learning techniques will improve the accuracy of identifying fraud attempts from erroneous copies. Account theft and unusual transactions. Fraud detection in payments often focuses on the user's behavior during transactions. A client, for example, visits a certain supermarket every evening between 9-10 pm. The supermarket is located close to the client's home. Payment amounts range from $10 to $400. The client drives to the gas station every two days.
The algorithm will be suspicious if a transaction is made in a bar in another part of the city and the amount is $40. It will then assign a high level of fraud probability. The system will send an email to the cardholder in order to verify this transaction.
For analyzing behavior, descriptive stats such as averages, standard errors, and high/low numbers are extremely useful. These metrics can be used to compare separate transactions against personal or intragroup benchmarks. Payments with high standard deviations are suspicious. It is a good idea to ask the account owner to verify any deviations.
Preventing Loan Application Fraud
Lending is sensitive about scams that misuse personal information in the finance industry. In just a decade, fraudsters had a hard time accessing IDs, pictures, addresses, and mobile numbers. Most data is now available on social networks and the Internet. Financial institutions have to deal with this. The fraudsters are becoming more sophisticated, the loan applications need to be assessed more thoroughly, and clients want their money as quickly as possible.
Personal details are counterfeiting. False personal information is a common fraud. Scammers will provide false personal information, including misspellings or misrepresentations about income or credit qualifications. It makes debt collection more difficult. There are two possible solutions to the problem.
The first includes swiftly checking record fields using open APIs after studying the history of a customer's associations with a certain bank to search for any discrepancies. The second method is more complex and requires a scoring model to be built or the calculation of fraud probability. The scoring models grade the records based on their fraud potential. It can help determine which applications are most likely to be fraudulent. By classifying applications, machine learning and advanced analytics can solve the problem by assessing fraud probabilities.
Solutions like this allow you to reduce costs by not having to verify every application and instead concentrating on only the risky loans. This improves the general credit score - a process for grading the creditworthiness of customers - by separating fraudsters and bad borrowers. By separating fraudsters from problematic borrowers, credit statistics are improved.
Machine Learning For Anti-Money Laundering
Banks, regulators, and investment firms all play a role in the monitoring of possible money laundering activities. They are required to detect suspicious activity and share information with each other.
Combining a rule-based model with a machine-learning approach can help uncover hidden relationships between money movements and criminal activities. This type of system can reduce the monitoring workload for small and medium banks. The presented technique decreased the number of reported transactions by 30 to 1% while revealing 99.6% of money laundering operations.
Want More Information About Our Services? Talk to Our Consultants!
Fraud Detection Systems: Common And Advanced
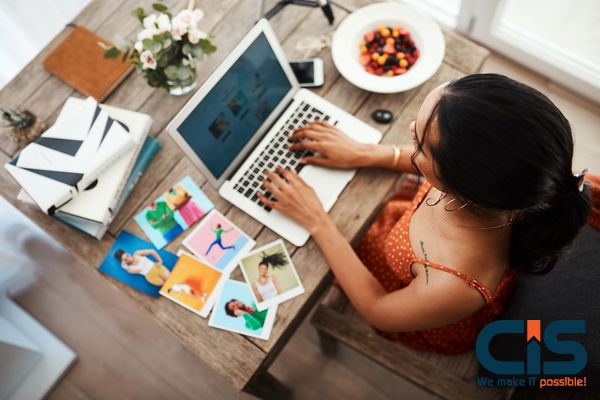
We've covered general fraud scenarios. Let's talk about how fraud detection engines are created and how machine learning is used.
Anomaly Detection For Suspicious Transactions
Data science uses a variety of anti-fraud techniques, including anomaly detection. The method is based on dividing all data objects into two categories: outliers and normal distribution. In this case, outliers are objects (e.g., Transactions that are different from the norm and considered fraudulent.
Data that can be used to detect fraud is a vast collection of variables. These variables range from transactional details to images and unstructured text. These parameters can be used to answer questions about anomaly detection algorithms:
- Are clients using services as expected?
- What are normal user actions?
- What are typical transactions?
- Do you find any discrepancies in the information that users provide?
This is the simplest approach, as it gives simple binary results. In some cases, this may be useful. If the transaction appears suspicious, for example, the system might ask the user to perform multiple additional steps of verification. Traditional anomaly detection does not reveal fraud. However, it can be used as a support tool for rule-based systems.
There are also more sophisticated approaches that combine multiple Machine Learning algorithms in order to reduce uncertainty. These can be implemented with a variety of machine learning styles, as well as mathematical models. Here are the most common ones.
Advanced Fraud Detection Systems
In many cases, advanced systems can identify patterns that indicate specific fraud scenarios. ML is used to detect fraud in two ways: supervised learning and unsupervised. These machine learning algorithms can be combined or used separately to create more sophisticated algorithms for anomaly detection.
Supervised learning involves training an algorithm with labeled historical data. In this situation, the target variables are already marked in the datasets, and the training goal is to have the system predict the variables from future data.
Unsupervised learning models classify unlabeled data into clusters and detect hidden relationships between variables. How can supervised and unsupervised learning styles be combined to create robust fraud detection systems:
- Labeling Data: Although data can be labeled manually, humans find it difficult to identify sophisticated fraud attempts based on their implicit similarities. Data scientists use unsupervised learning to group data items into clusters that account for hidden correlations. Data labeling is more precise. Not only are fraud/nonfraud items labeled, but the labels also differentiate between different types of fraudulent activity.
- Training A Model Under Supervision: After the data has been labeled, it is time to use this labeled dataset in order to train a supervised model that will detect fraudulent transactions during production.
- Ensembling Models: In data science, assembling multiple models is common. Even if you make one model, it'll always have strengths and weaknesses. It will identify some patterns but miss others. Data scientists often combine different methods or multiple models to make more accurate predictions. All models in the ensemble will analyze the same transaction, and they'll "vote" to come up with a decision. This allows you to leverage the strengths of different methods in order to make a decision that is as precise as possible.
- Set An Express Verification: Ensembles require a lot of computing power to process all the data. The time it would take to calculate all transactions could be detrimental to the user's experience. It's a good idea to do the verification in two stages. Express verification is a simple anomaly detection method or a straightforward ML technique to separate all transactions into normal and suspicious ones. Regular transactions do not require any further verification and are therefore approved by the system. Those who appear suspicious are sent to a complex group of people for advanced verification.
Read More: What Is Machine Learning? Different Fields Of Application For ML
Machine Learning Algorithms For Fraud Detection
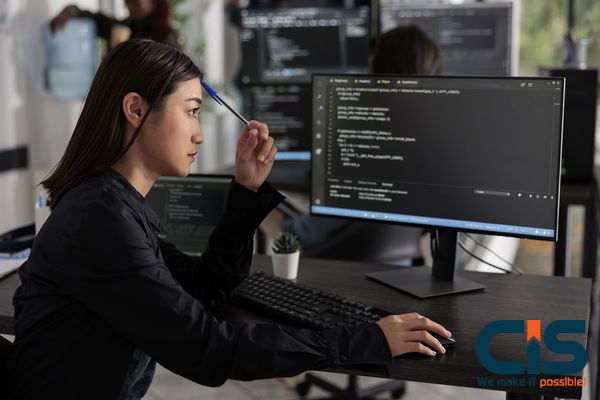
There are five types of machine learning methods. We will only cover supervised learning methods, as they are the most commonly used for building complex ensembles.
Random Forest
Random forest is a decision tree algorithm that builds trees for classifying data objects. The model chooses a variable that allows the best splitting of data records and then repeats this process many times. If we visualized how the algorithm worked, it would look like an image of a tree. Data scientists use random subsets of a dataset to train multiple decision trees. This allows them to make more accurate predictions. Trees vote, and the model gives a consensus judgment to decide if a transaction appears fraudulent.
Pros
You can set up fraud detection quickly using random forests. Payment systems are the most common application. Random forests are fast and simple to use. They can also be used for different data types, such as credit card numbers, dates, or IP addresses. They are considered to be precise predictors and can work with datasets that have missing records.
Cons
Sometimes engineers get confused by the problem of overfitting. Overfitting is when the model remembers patterns from the training data too well and cannot make accurate predictions for future data. The dataset balance is another problem. The accuracy of a dataset may be affected if it contains a majority of normal transactions with only a small percentage of fraudulent ones.
Support Vector Machine
Support vector machines (SVMs) are supervised machine-learning models that use a binary linear non-probabilistic classifier to group records within a dataset. What does this mean? The algorithm divides the data into two distinct categories with a clearly defined gap. The division line can be defined by creating several hyperplanes on the multidimensional surface. The algorithm then selects the hyperplane that separates records the best. SVM is inferior to the random forest in the analysis of credit card transactions when using small datasets. However, it can approach their accuracy with large datasets.
Pros
Support vector machines excel at dealing with complex multidimensional systems. The support vector machines also avoid the problem of overfitting that may occur with random forests. SVM is used a lot in the detection of credit card fraud. The abundance of research makes it easier for data scientists to adjust SVM models for credit card fraud.
Cons
The complexity and accuracy of SVM models require a lot of engineering work to achieve. SVMs can be very slow and computationally intensive. This is why they'll need a powerful computing architecture.
K Nearest Neighbors
The k-Nearest Neighbor algorithm classifies records based on similarity in multidimensional space. The record is assigned to a class of nearest neighbors. Each cluster's record votes for a new record using distance. K-nearest neighbor is another popular approach to analyzing credit card transactions.
Pros
The method is not sensitive to missing or noisy data. This allows it to be configured with larger datasets and less preparation. Additionally, it is quite accurate, and modifying models is easy.
Cons
Like neural networks, K-nearest neighbors require powerful infrastructures. They also lack interpretability.
Neural Networks And Deep Neural Networks
The neural network is a model which allows the determination of non-linear relationships between records. The algorithm structure is based on principles similar to those found in human brain neurons. The model is built on a labeled data set, which passes input data through multiple layers. Sets of mathematical functions. Models of this type use 1-2 hidden layers.
Deep Neural Networks are similar to neural networks, but they have many more layers. This results in more accurate data but also requires more computing power. In the last few years, deep learning has revolutionized data science. It has also affected the financial services sector. At the moment, neural networks can be used for both transactional verifications as well as insurance claims.
Pros
Deep neural networks, and in particular deep neural networks, are very powerful when it comes to finding complex and non-linear relationships within large datasets. This is true for both transactional data as well as text and image analyses, which can be used for insurance cases. Because they are usually highly accurate, neural networks are a vital part of modern fraud detection systems.
Cons
Neural Networks are high-tech systems that require a lot of work to create and optimize. These networks require highly-skilled professionals and powerful computing architecture. We don't recommend this method to analyze all transactions. The lack of interpretability is another major issue with deep neural networks. It's difficult to determine how the system came to a particular conclusion, even if it is highly accurate.
Want More Information About Our Services? Talk to Our Consultants!
Final Word
The right Machine learning models and methods to use depends on a number of factors, including the type of problem, the size, and complexity of the dataset, the resources available, etc. It is a good idea to use multiple models in order to streamline the assessment process and improve accuracy.
Anti-fraud systems must meet these standards of today:
- Detect fraud in real time.
- Improve data credibility.
- Analyze user behavior.
- Discover hidden correlations.
Machine learning algorithms can offer these benefits to business models, but they also have some serious disadvantages. For example, they still need large datasets that have been carefully prepared for training. They also still require some features from rule-based engines, like checking legal limits for cash transactions. Machine learning solutions require data science expertise to create complex ensemble algorithms. It is difficult for small and mid-sized companies to leverage internal talent.