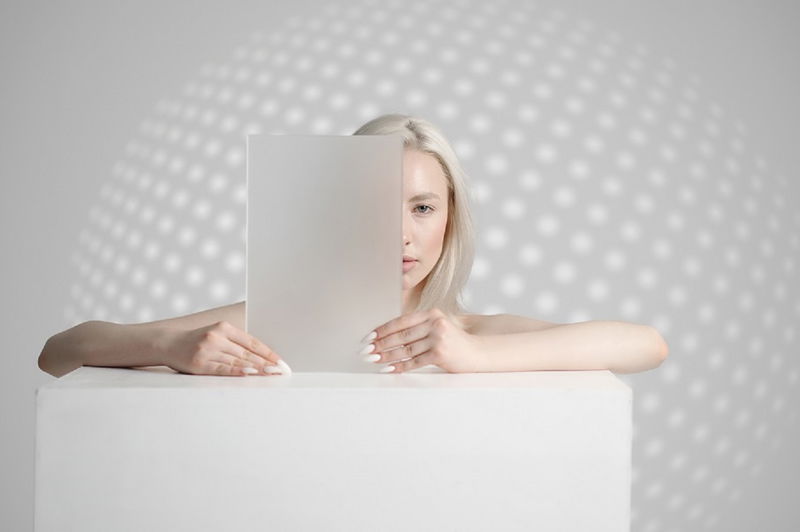
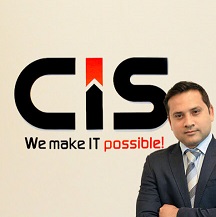
Contact us anytime to know more β Kuldeep K., Founder & CEO CISIN
Google Tensorflow and Facebook PyTorch dominate deep Learning as critical disciplines.
Hadoop and Spark projects revolutionized the manipulation and analysis of large data sets (which exceed one machine). Their tools for artificial intelligence (AI) are among the finest available today; some proprietary programs may enable machine learning without code writing, but modern OSS offers far superior analytics.
Politicians care deeply that some of the top machine learning tools are free. Open-source software deserves greater consideration.
The United States has recently shown strong support for open-source software development (OSS). Notable among these efforts is its Federal Source Code Policy, which encourages agencies and contractors to share more code among themselves.
Unfortunately, AI remains less well known when considering the relationship between OSS policy and AI policies.
Trump administration documents on AI regulations and use at federal agencies only briefly mention OSS, while Obama's AI Strategy mentions its relevance only within the AI context. According to a new report, Europe OSS policy lacks "a clear connection with AI strategies and policies... of most countries", while recent AI regulations do not even acknowledge its existence as an option for implementing their strategies and policies.
AI analyses and comparisons typically focus on data, talent, funding and semiconductors - yet rarely address OSS (Open Source Software).
Unfortunately, this has led to AI policy being silently affected. Tools created within an OSS environment help accelerate AI adoption from science and industry while supporting ethical AI practices - an impactful way for an open-source system like Google or Facebook to operate while breaking away from traditional standards and simultaneously creating innovation across many fields.
OSS speeds AI adoption

OSS makes adopting AI easier by minimizing the technical knowledge necessary for implementation, often taking hours to complete.
Transcribing complex algorithms from math into code takes too much time and resources; by eliminating unnecessary processes. Open-source solutions can be an enormous boon to data scientists. Open-source developers collaborate on projects for community input while hoping to produce popular OSS.
Open source development often results in multiple versions of an algorithm being written, with those which provide greater efficiency or are best documented being chosen over their competitors.
Open source can not only be competitive but can also foster collaboration. Because OSS code is publicly accessible, it's possible to cross-check and examine it for errors or improvements. When coupled with collaborative development and an active community surrounding popular OSS platforms, this collaborative-competitive environment often results in accessible, robust code with the highest possible quality standards.
Not to disparage data scientists, but many lack the mathematical training to develop complex algorithms.
While not meant to criticize them, their work often necessitates being more than mere mathematicians. Data scientists must also be able to explore data and solve programmatic issues. Most often, they focus on interpreting results from analyses they perform and effectively fitting algorithms into digital products or services.
Open-source AI software written correctly can assist data scientists in taking advantage of cutting-edge machine-learning algorithms, making machine learning-accessible.
Open source AI code also has an economic benefit by making AI easier for more people - though at times, its ease of use also facilitates harmful innovations like cyberattacks or deep fakes.
Want More Information About Our Services? Talk to Our Consultants!
Open Source Software Solutions help reduce AI bias
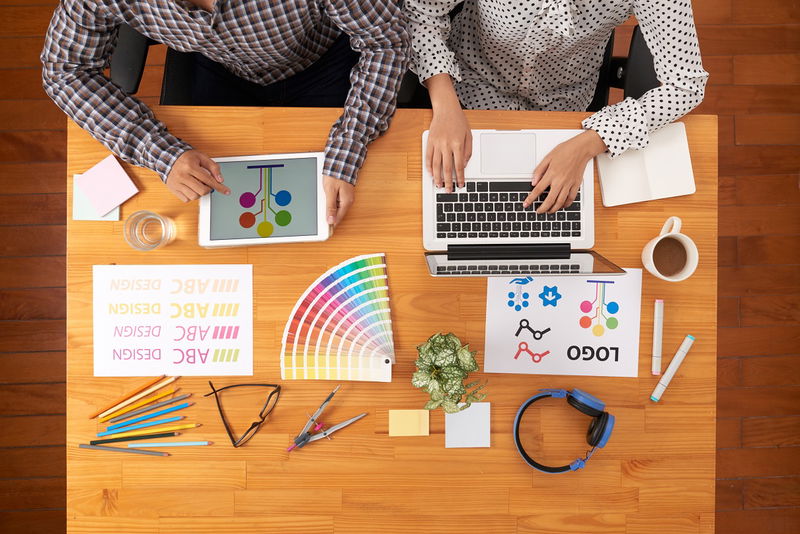
Open-source AI can also facilitate wider, better, and more ethical AI usage. Open source software (OSS), such as IBM's AI Fairness 360 or Microsoft Fairlearn/University of Chicago Aequitas, may help lower technical barriers associated with bias detection in AI algorithms.
Interpretable AI tools that are both open-source and explainable - like IBM's AI Explainability 360 or Chris Molnar's interpretable machine learning tool and book - allow data scientists and engineers to comprehend model workings. Importantly, private company data scientists and machine-learning engineers often operate within a limited time in highly competitive markets.
Data scientists and machine learning engineers must focus on building models and products without being distracted by bias issues, knowing their time will be best spent developing ethical AI systems. Open-source code provides data scientists who may otherwise lack time for this work with a convenient means of discovering and mitigating discriminatory machine-learning aspects.
While more oversight by governments will undoubtedly be necessary for AI technologies, more focus should be put on investing in open-source software to facilitate ethical AI applications that have positive societal benefits rather than solely being used for academic research projects.
At present, most government funds go toward code development for academic studies.
In their funding of critical Open-Source Software, Zuckerberg Initiative states that it plays an essential role in modern scientific and research endeavors, yet most commonly used research software lacks dedicated funding sources.
Ethical AI research faces similar difficulties; government grants only go toward early-stage research of open-source software solutions (OSS). Meanwhile, Amazon and the National Science Foundation provide substantial financial backing for academic work on AI fairness research.
Although AI research will likely yield useful open-source software (OSS), its development, documentation and dissemination may prove challenging without sufficient funding support from government and foundation sources. When considering ethical AI funding options, they should look at OSS development as one potential way of supporting this endeavor.
Read More: Why Should AI in the Life Sciences Be Developed Using Open Source?
AI Advances Science with Open-Source Tools
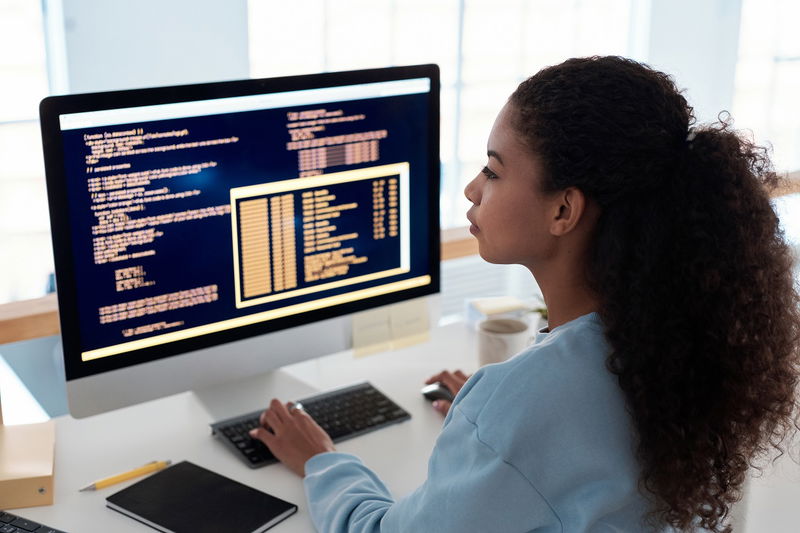
Open-source AI has more to offer scientific researchers across multiple fields than tech companies.
Francoischollet, the developer of open-source AI Keras software, tweeted out several replies showing its potential in building neural interfaces and identifying subcomponents within mRNA molecules to aid those living with visual impairments in seeing better. Separating these roles - that of a developer from a scientist - is becoming more prevalent, allowing for improved tools and scientific advancement.
Scientists cannot produce novel knowledge within their field while simultaneously using statistical tools for discovery.
Even before machine learning reemerged as a powerful field in science, open-source software (OSS) was an integral component. Entire ecosystems of OSS tools often develop around specific scientific endeavors: for instance, the OSS Bioconductor project was established with over 2000 tools specifically tailored for genomic analyses.
Not having seen Open Science tools used in AI at once should not detract from their value or lead one to believe their proliferation was guaranteed.
presented us with serious gaps, yet these have since narrowed significantly, with efforts underway at increasing percentages (currently 50% for Neural Information Processing Systems Conference papers released public code and 70% at International Conference of Machine Learning conferences respectively) of papers releasing code publicly (currently 50% and 70% respectively for Neural Information Processing Systems Conference and International Conference of Machine Learning respectively). Policymakers must continue supporting and encouraging OSS in science (using NSF Fairness in AI Program initiatives) without hindering it in any form whatsoever.
Open Source Software (OSS) makes replicating research easier, enabling scientists to verify each other's findings when science remains plagued with a replication crisis.
As this software is accessible to many researchers, reproducible research becomes much simpler. Reducing uncertainty about an experiment's conduct or analysis by having access to all researchers that conducted it or used an analysis formula is made much simpler using one OSS in all fields analyzed, providing easier analyses.
OSS AI poses both threats and opportunities for technological companies competing within its space

OSS also has significant implications for competition policy. Though, at first, it may appear to increase market competition, the public release of code for machine learning broadens its application and makes it simpler for more users to utilize.
As previously discussed, this development can benefit industries in multiple ways and allow more AI implementation with reduced expert involvement. Unfortunately, open-source AI tools will be powerless against large technology firms' anticompetitive practices or growing power over time.
Google, Facebook and Amazon enjoy an advantage over competitors due to proprietary data, network effects and online platforms with which they operate. Competing against large corporations does not primarily depend on being able to implement similar algorithms as quickly.
Google and Facebook could find that open-sourcing their deep learning tools (Tensorflow and PyTorch, respectively) strengthens their position by further solidifying it in the market.
Google and Facebook seem reluctant to release open-source software despite its potential benefits of increased community involvement and equalized influence distribution. Tensorflow was initially released under open source; however, Google continues to pay administrative staff associated with the Tensorflow project.
Like Google and Facebook's approach, most core PyTorch developers work for Facebook as well, which should come as no surprise; yet still noteworthy. By opening-sourcing these deep learning tools, Google and Facebook do not give up control yet rather make these technologies more widely accessible while increasing transparency of operation - with open-sourcing their goal being 'democratizing technology via open source licensing (OSS).
Companies like Caffe and Theano appreciate open-source software (OSS). Meanwhile, standalone OSS AI products that do not receive support from these firms seem to lose significance within AI research and industry.
Google and Facebook gain from public research using their tools by making them standard in academia and industry, creating a pipeline of data scientists and machine-learning engineers trained on them while enriching public discourse through public engagement efforts with these systems. Tensorflow, PyTorch, and various open-source tools from Google and Facebook are helping them remain leaders in AI technology.
Fast.ai and Keras - two open-source extensions to Tensorflow or PyTorch, respectively - add functionality while making user use easier. Together, these benefits make it evident how creating open-source software tools plays a significant role in these companies' strategies.
Google and Facebook did just this with web development tools by releasing Angular.js and React.js, respectively; both also stand to benefit greatly by dominating open-source deep learning systems, thus keeping this competition within technology in mind when discussing competition within this market sector.
OSS generates AI Standards
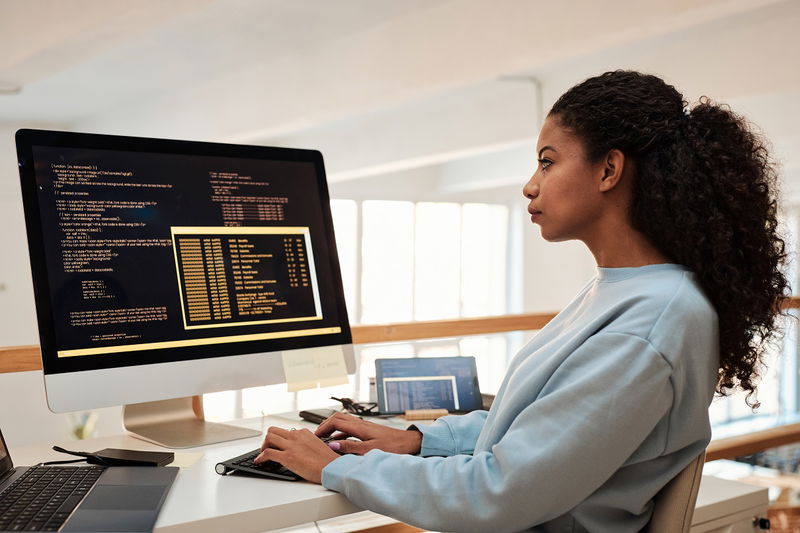
OSS AI also has profound ramifications on some cornerstones of international policy debate--namely, standards bodies.
IEEE, ISO/JTC, European CENELEC-CENELEC, and U.S. NIST are among the many bodies actively trying to influence AI as it rapidly develops; all competing for dominance.
Furthermore, default standards created by open-source solutions such as Google Cloud have become de facto standards that must be managed.
Standards bodies in other industries have attempted to promote best practices and foster interoperable technologies, while in machine learning, this means encouraging consistency and interoperability within various OSS packages.
AI interoperability issues have received considerable consideration due to the wide use of various programming languages, operating systems and tools.
As such, much work has gone into devising technical interoperability solutions without consistent code, like containerization software or cloud-based microservices. As new technologies enter the industry, standards have become less appealing and have been widely adopted by data science communities such as Twitter, blogs and OSS documents distributing practices and standards in informal ways that make compliance difficult to track down and enforce.
Standards bodies must invest significantly into engaging this community in their processes to be effective.
Deep Learning presents many unique challenges to standard bodies. Google and Facebook may resist ceding their influence over deep-learning development due to Tensorflow/PyTorch's prevalence; IBM/MSFT remain highly involved with standard-setting bodies indicating they believe these standards have an important purpose and purposeful effects; there remains some debate as to the exact relationship between open source standards (OSS) and international AI standards (AIS).
However, these discussions should include their participation.
AI Policy and Open software go hand-in-hand, inextricably linked
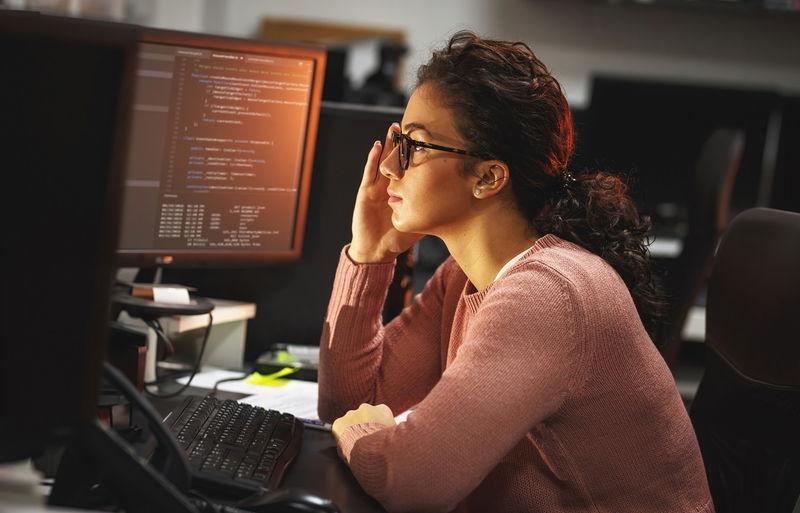
Open-source code is central to AI development, from research and ethics to competition and innovation.
Unfortunately, open-source developers often remain absent from AI policy development discussions despite having significant expertise and influence over it. By including more OSS AI programmers in AI policymaking discussions, AI policymakers will have a greater opportunity to evaluate OSS and its effect in meeting our desired goals - equitable, just, and prosperous AI use - while opening up different and vital discussions.
Perhaps more OSS programmers may even lead us down unexpected pathways leading to important questions posed by other policymakers and researchers. Do we desire an AI world governed by software controlled by corporations but open source? How can we best promote AI's beneficial use, and what role should standards play? In an ecosystem powered by open-source AI algorithms, the goals and challenges for governance will be tied directly to open-source code; policymakers may more accurately gauge its influence in driving development by engaging more OSS AI programmers.
Life Sciences are no exception to this development; artificial intelligence (AI), for instance, has proven essential.
Life Science technologies today can vary considerably; AI allows faster processing of large volumes of data - an advantage relevant to Life Science applications. Over the coming years, your party is likely to experience a significant transition. How will you arrive at your desired goal?
Machine learning and advanced analytics have already demonstrated tangible advantages in the therapeutic services sector.
Doctors use machine learning software to plan treatments; it also identifies which treatments may best serve individual patients (self-treatment/self-finding etc.), automating many repetitive processes for doctors and assistants so they can concentrate on providing care to their patients instead. AI-assisted analytics has numerous benefits extending from everyday life and science - including managing massive volumes of clinical trial data - that cannot be underestimated.
Open Source Development and AI

Open-source development has long been practiced within the industry. Anyone may view and improve upon an innovation or solution's source code to make modifications that increase product quality and development by encouraging developers to collaborate in fixing bugs together.
Studies have proven the effectiveness of open source development when applied effectively - by giving anyone access to its source code; anyone can help make necessary improvements and changes more efficiently than traditional approaches alone can manage.Rapid product usefulness improvement can be achieved by improving existing features and creating entirely new capabilities.
Open source has proven particularly appealing in the AI community. Not only has open-source become popular for current AI frameworks like Hadoop, SPARK and Microsoft AI solutions such as their cognitive analytics stage SPARK-Powered OpenText(tm), Magellan, but many sellers also utilize open-sourcing for their AI solutions - OpenText's AI-enhanced Analytics Solution Magellan being one such example that uses SPARK cognitive analytics stage technology.
Open-source AI is increasingly sought-after because its uses do not limit creativity.As more individuals join, advancements will likely follow suit, which can only happen within communities that actively address quality, consistency and certainty issues.
Accessing data is vitally important for any developer - be it Amazon upgrading Alexa or healthcare developers working on hereditary data. From investigations and factual investigations to adjustments and enhancements - accessing necessary data allows any development.
Life Sciences professionals and open-source concepts have come a long way, taking full advantage of tools like healthcare.ai.
Together they collaborate on improving healthcare using open-source AI networks like healthcare.ai to achieve positive change for patients. This represents a monumental breakthrough for data democratization: any individual with access and the capability of using data sets now has access and can take full advantage of them.
Opening the Black Box
Briefly, I will address the division of labor. AI research has highlighted its ability to dislodge control from central leaders and thought leaders; fate-laden science fiction even predicted their rise! Cognitive analytics - an AI technique using cognitive analysis to process data - can handle Big Data sets.
Intelligence for such analyses comes from humans conducting tests, creating hypotheses, modeling algorithms and making informed decisions; unlikely this trend will change anytime soon.
As is true with information gleaned through bottom-up approaches, understanding hypotheses and issues surrounding any given problem being tackled, as well as being aware of subtleties, is vital for solving complex challenges in Life Sciences such as healthcare providers such as doctors, nurses and specialists; their input plays a huge role in creating analytical AI solutions through AI upgrade solutions for life science problems.
Large programming companies commonly spend millions to protect their intellectual property through Artificial Intelligence development; this method, however, has three major flaws.
Product development may become hindered as models and algorithms can only be modified by merchants; community involvement cannot have any real bearing on future developments.This could hinder similar products being developed; since sellers retain control of the product but do not have permission from others to deliver complementary or extra items.
Discovery AI may also erode trust. Without providing transparency on the exact methods by which results were attained, Discovery AI makes no statement as to its exact results or why certain treatments have been prescribed; such results must also remain reliable throughout a product's lifetime cycle.
Solving these complex challenges using traditional displays for programming progress alone is difficult; therefore, we should employ an open-source strategy which empowers Life Science and Innovation experts to come together in teams to research, prototype and adapt new AI solutions.
Open source software enables clients to collaborate in sharing and creating reports using real-time code, perceptions and conditions data. The network can collaborate on developing algorithms or models - notebooks were not chosen due to their popularity among Life Scientists.
Open Source AI: Benefits

AI is advancing at a rapid pace. ChatGPT and other AI-powered platforms have made what was sci-fi just a few short years ago a real possibility.
Guttmann says that while the capabilities of AI are impressive, it needs to be fine-tuned before they can become a viable business tool.
Custom ML models can be difficult to build from scratch, and generic APIs may not always be tailored to specific use cases.
Guttman predicts that more companies will download open, pre-trained ML models to retrain or customize them for their needs.
Adopting an open-source strategy has many advantages for software providers. Guttmann said that the visibility created by open source helps a project evolve with feedback provided by the community.
In turn, this helps companies build more stable and better products. ClearML, for example, uses a lot of OSS in its backend, user interface and other components. They also provide a popular CI/CD open-source framework for ML workflows.
Open Source AI Development: Use Cases
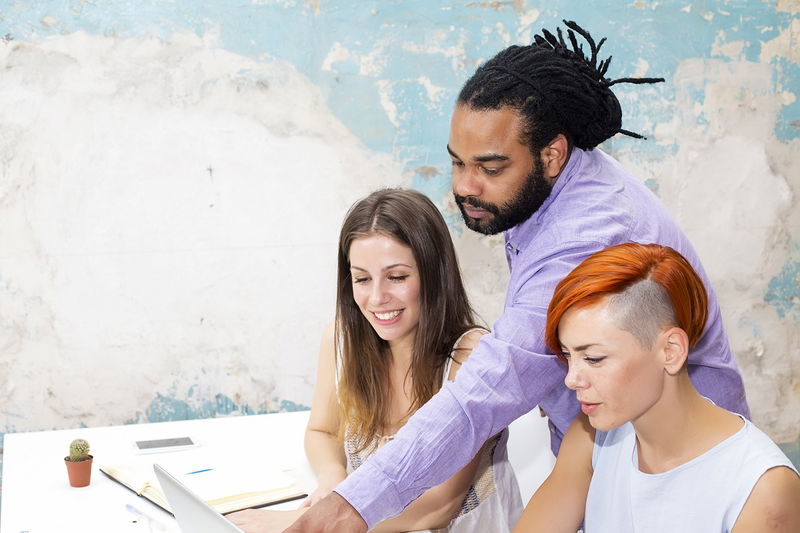
Open-source software drives innovation on multiple levels. There are numerous open-source projects designed to speed AI development.
These include pre-trained models, data structuring tools and frameworks for training models, and AI deployment pipelines. Many open-source machine learning frameworks - TensorFlow is just one among several free options, such as PyTorch or H2O - help engineers create and train their models quickly and effectively.
Guttmann noted that training models are time-consuming and complex; therefore, an alternative to this process may be accessing pre-trained models on GitHub repositories; such tools include PaddleNLP, Natural Language Toolkit and DeepFaceLab, which provide tools for deep fakes/machine vision applications as well as many open source Python packages to clean/structure data for training ML models.
Guttmann highlighted that automating an abstraction layer would provide data scientists and machine learning engineers access to product-grade hardware for training AI or running it themselves.
Open Source Use: Potential Negatives

There may, however, be downsides associated with open-source software in AI research.
Open source can pose risks, especially in light of supply chain attacks, which remain ever-present. Bad actors could exploit numerous vulnerabilities within an open-source package to compromise it - name confusion, unmaintained/untracked software, and malicious code can all present potential compromise points that must be monitored closely; license restrictions could prevent its inclusion into certain projects altogether.
Thankfully, open-source software (OSS) appears more beneficial than anticipated. Automation-powered vulnerability scanning tools allow teams to identify issues more quickly, while 89% of tech leaders agree that open-source enterprise software offers equivalent or superior protection than proprietary products.
How to Get the Best Out of AI
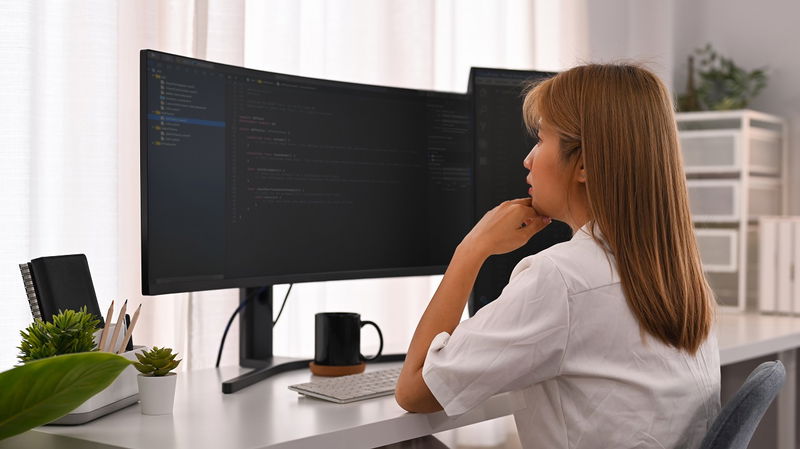
Open source is at the core of digital innovation in multiple fields, including artificial intelligence (AI).
A key aspect to fully capitalizing on AI will be operationalizing machine learning (ML). By setting up appropriate automation infrastructure, you can achieve the agility to retrain models continually; Guttmann noted that security AI/ML should be separate from normal CI/CD processes.
Guttmann believes companies, in general, must be transparent regarding ML models; this includes disclosing details about how these ML models were built and details surrounding any datasets used to feed these AI systems or ethical considerations that could arise.
In addition to that gap being filled through transparency measures regarding artificial intelligence systems (AI), another gap must also be filled: restricting "hallucinations" caused by generative AI that produces output like hallucinations: this could be resolved by giving AI systems the freedom to respond by saying simply, "I Don't Know" rather than boldly spewing out useless words of text out loudly proclaiming itself without thinking.
Want More Information About Our Services? Talk to Our Consultants!
Conclusion
Open-source AI/ML solutions offer many advantages over proprietary ones.
Countless mature open-source tools enable engineers to develop complex AI and manage the deployment of custom machine learning (ML). However, maintaining this open creed while remaining transparent about final creations remains key.