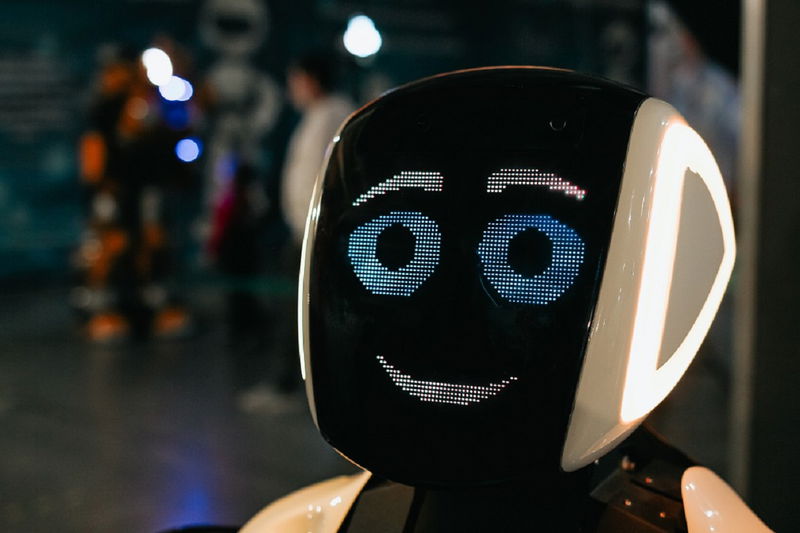
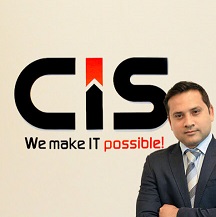
Contact us anytime to know more β Kuldeep K., Founder & CEO CISIN
AI and machine learning (ML) technologies have gained increasing traction with businesses as businesses use these products to analyze large volumes of data quickly, generate real-time insights, recommend actions based on this analysis, as well as create accurate forecasts.
What are the differences between Machine Learning (ML) and AI technologies, how are they related, and what impact these have for organisations today?
We will compare AI and ML.
Artificial Intelligence, commonly referred to as AI, has quickly become one of the hottest topics in technology over recent years - for good reason! There have been multiple advances that were once only fiction turning reality slowly but surely.
Artificial intelligence (AI) is an innovation which many experts see as having the potential to change how people work across industries and introduce new sources of growth.
According to this PWC article, experts predict AI could contribute up to $15.7 trillion globally to GDP by 2035 and that China and the United States would see the most benefit - together accounting for almost 70% of impact globally.
Artificial Intelligence
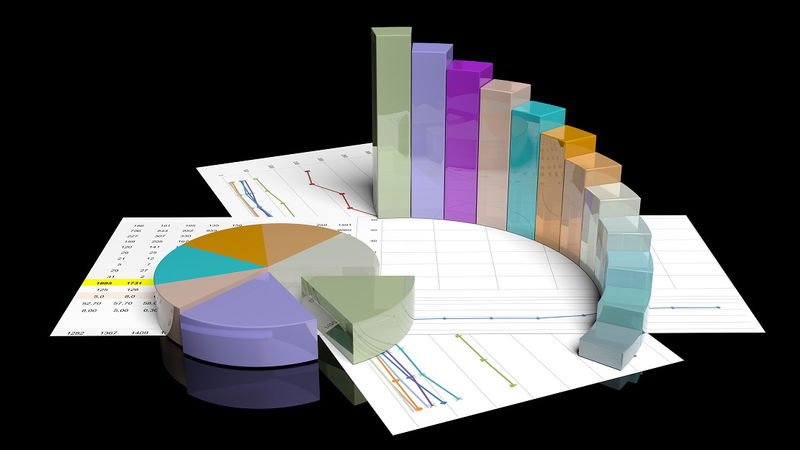
Artificial Intelligence (AI), commonly referred to as machine learning, refers to the capacity for machines to mimic or enhance human intelligence.
Artificial Intelligence has traditionally been employed in computer programs but now being applied across products and services such as digital cameras to detect objects within images; experts predict that artificial intelligence will continue to find use in innovative ways in future applications.
AI is a practical application of algorithms, probability theory and economics used to solve real world issues. Additionally, its applications span computer science, mathematics and psychology disciplines; mathematics provides machine learning tools that model optimization issues while computer science offers models.
AI (artificial intelligence) has existed since Alan Turing proposed his "imitation game" back in 1900 to test machine intelligence.
But only within recent decades have we had access to sufficient computing power for AI systems creation.
Consider what distinguishes humans from other animal species - their ability to apply lessons learned in past experiences to new situations, thanks to our powerful minds with more neurons than other animal species.
Computers today still cannot match our biological neural networks, yet they do possess one major advantage over humans: They can analyze vast quantities of data much quicker.
AI can help you focus on what matters and make better choices based on data acquired for specific use cases. AI has proven useful for complex tasks like predicting maintenance needs, detecting fraudsters or finding optimal delivery routes; its automation automates many business processes so that you can devote yourself solely to core business goals.
Research in this area centers around developing machines capable of automating tasks that require intelligent behavior - for instance answering consumer and diagnostic inquiries, handwriting processing, natural language understanding, speech recognition as well as moving/manipulating objects.
How Does AI Work?
As AI becomes increasingly buzzyworthy, vendors have scrambled to demonstrate its use within their products. What we often refer to as artificial intelligence (AI), however, refers more accurately to machine learning technology that relies on special hardware and computer software in order to train machine learning algorithms and write machine learning programs; Python, Java, C++ Julia and Julia are popular choices among AI developers as coding languages for writing machine learning programs.
AI systems generally work by analyzing large volumes of training data to find patterns and correlations, then applying those patterns to predict future states.
A chatbot, for instance, can learn from millions of examples to simulate conversations between humans; similarly an image recognition program can learn to recognize objects in images after reviewing similar examples; with rapidly improving AI techniques now at their disposal creating realistic images, text documents, music tracks or media can now be produced as real experiences for human viewers and listeners alike.
AI programming is based on cognitive abilities that include:
- Learning: The AI aspect that focuses on this is the creation of rules to transform data into useful information. These rules, also known as algorithms, provide computers with detailed instructions on how to perform a particular task.
- Reasoning: The AI aspect that focuses on selecting the best algorithm to achieve a desired result.
- Self Correction: AI programs are designed to constantly fine-tune their algorithms, ensuring they produce accurate results.
- Creativity: This aspect of AI uses neural networks, rule-based systems and statistical methods, as well as other AI techniques, to generate new images and text, and to create new ideas.
AI: Different Fields To Clarify Common Misconceptions

Artificial Intelligence (AI) has quickly become one of the hottest fields in computer science. However, with technology constantly progressing at such a fast rate and multiple branches within this category each possessing their own algorithmic systems and fields within them forming AI's multifaceted nature it can be difficult to keep pace with technological development and research advances relating to it.
As AI refers to many fields rather than one single specialism it's essential that practitioners recognize it is composed of many intertwined fields rather than one singular thing!
Artificial Intelligence, more generally defined, refers to computers' abilities to perform tasks that would normally require human intelligence.
AI encompasses two fields - Machine Learning (ML) and Neural networks (NN), both part of Artificial Intelligence; each subfield within AI offers its own set of solutions for solving problems.
Machine Learning
Machine Learning (ML), commonly referred to as Machine Learning, is a technique which enables computers to learn from experience in order to improve specific tasks or processes by applying statistical and probability theories.
ML does this using probabilities as its framework. Machine learning is an approach for analyzing data and making decisions without explicitly programming, without resorting to explicitly designed algorithms.
Machine learning algorithms may either be classified as supervised or non-supervised algorithms with unsupervised ones capable of drawing meaningful inferences from data sets. Supervised algorithms use knowledge they have amassed over time to apply it in new situations. Machine learning algorithms aim to discover linear and nonlinear relationships within data.
This feat is accomplished using statistical methods for training an algorithm to classify or predict from given datasets.
Deep Learning
Deep learning is an artificial neural network subset that offers state-of-the-art accuracy in speech recognition, object detection and language translation.
Deep learning technology powers driverless cars. Furthermore, its immense memory storage capacities permit analysis of massive volumes of data; for example recognizing faces in images or videos.
Neural Networks
Artificial neural networks mimic biological neurons found within our bodies' neurons by being made up of layers called "neurons", each composed of mathematical functions designed to process data to predict output values.
Artificial neural networks learn by example similar to how people do and typically consist of three layers at least: input layer, hidden layers and nodes called neurons with weighted outputs within each.
Machine learning models have reached their maximum performance capacity and adding additional data does not help; instead, deep learning models show markedly enhanced results when given more data to work with.
These fields utilize different algorithms depending on their application. Some examples are decision trees, random forests, support vector machines (SVM), K-nearest neighbor algorithms etc that all serve to facilitate machine learning applications; as well as neural networks like convolutional neural networks (CNN), recurrent networks (RNNs), short term memory networks etc used for artificial intelligence purposes.
As AI devices vary greatly in strength and capabilities, classification requires further subdividing it. "Narrow AI", meaning machines that specialize in one task such as image recognition or chess.
By contrast, general AI refers to machines capable of doing all that humans do plus more; many researchers seek ways to use machine learning techniques for such general AI; although much current research focuses on narrow AI.
Want More Information About Our Services? Talk to Our Consultants!
Why is Artificial Intelligence Important?
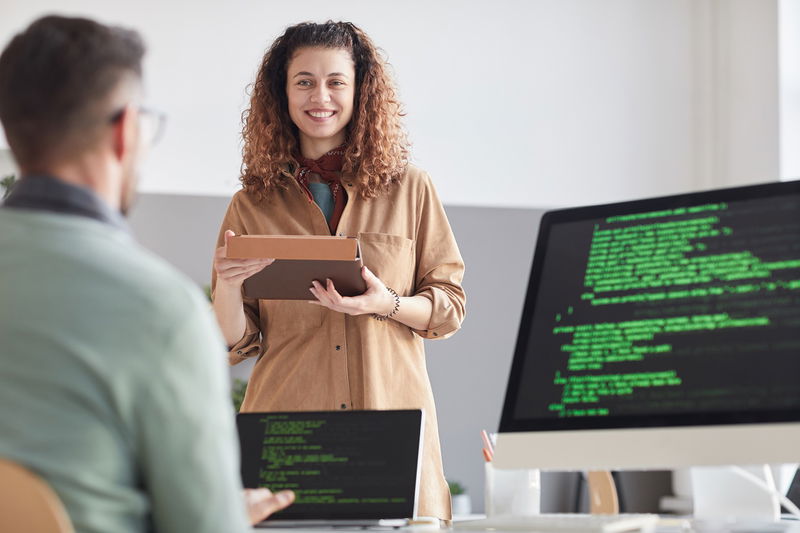
AI holds great promise to revolutionize how we work, live and play. Businesses use it to automate human tasks such as customer service, lead generation and fraud detection; AI excels at performing these duties more efficiently than humans do in various fields; for instance analyzing large volumes of legal documents to ensure all necessary fields have been appropriately filled out managing massive data sets gives businesses insights they otherwise wouldn't; while tools which generate AI tools become ever more crucial with each passing year; this trend holds true across education, marketing and product design fields alike.
AI has not only led to increased efficiency but has also opened new avenues of opportunity for larger organizations like Uber.
A Fortune 500 firm using software to connect taxi drivers and riders has seen incredible growth using this unsupervised learning technique.
AI is already used by some of the world's most successful and largest corporations such as Alphabet and Apple; Microsoft, Meta and Microsoft employ it to outperform competitors and enhance operations; Alphabet's Google subsidiary uses AI in various products like search, Waymo self-driving vehicles and Google Brain; the latter has pioneered transformer neural network architecture which has resulted in many advances in natural language processing capabilities.
What Is Machine Learning?
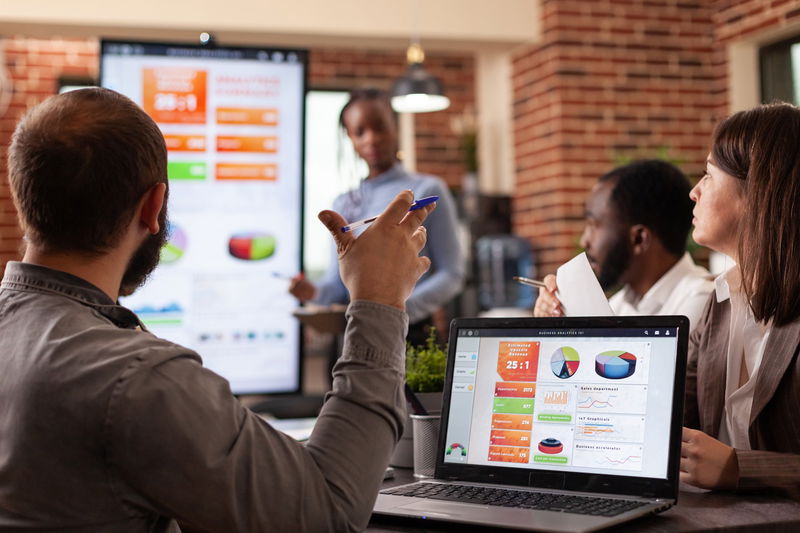
Machine learning (ML), is an AI and computer science branch which utilizes data and algorithms to simulate how humans learn while increasing its accuracy.
Over the last two decades, technological innovations in storage and processing have given birth to revolutionary machine learning-powered products like Netflix's recommendation engine and autonomous cars.
Machine learning is one of the fastest-emerging fields of data sciences. By employing statistical techniques to train algorithms that use predictions or classifications and uncovering key insights for data mining projects, machine learning provides key contributions towards driving business and application decisions that should positively affect key growth metrics.
As more big data becomes available and grows exponentially, more data scientists will be needed in order to identify business questions, their data sources, and possible answers that might provide answers.
Machine Learning: How It Works
UC Berkeley divides the machine learning algorithm learning system into three parts.
- A decision process: Machine learning algorithms are generally used to make predictions or classify data. Your algorithm will estimate a pattern based on input data that can be unlabeled or labeled.
- A model's prediction is evaluated by an error function. An error function can be used to compare known examples and assess the accuracy.
- A Model Optimization Process. If the model fits better with the data points from the training set then weights will be adjusted to reduce discrepancy. The algorithm will continue to "evaluate-and-optimize" the weights until it reaches a certain accuracy threshold.
Machine Learning Methods
Three main categories of machine learning models exist.
Supervised Machine-Learning
Supervised learning involves training algorithms from labeled data sets for accurate classification or prediction purposes using weighted models that adjust as input data is entered until its weights have become appropriately fitted - through cross-validation this occurs to prevent overfitting or underfitting of models.
Supervised Learning helps organizations address real world issues on an immense scale such as sorting spam into separate folders within your inbox or classifying spam into another. Methods commonly employed within Supervised-learning include neural networks (naive Bayes), linear regression, logistics regression random forests support vector machines (SVM).
Unsupervised Machine Learning
Unsupervised machine learning (unsupervised learning) employs machine learning algorithms for the analysis and clustering of unlabeled data without human supervision, using these to discover hidden patterns or clustering without human interference.
Unsupervised machine learning's capacity to find similarities and differences makes it ideal for exploratory data analyses, cross-selling customer segmentations analysis as well as image identification purposes without human interference. Dimensionality reduction techniques like principal component analysis (PCA), singular value decomposition as well as neural networks kmeans clustering or probabilistic methods are utilized during unsupervised machine learning projects.
Semi-supervised Learning
Semi-supervised learning offers a compromise between supervised and non-supervised approaches, using only labeled datasets as training data to guide classification of an unlabeled set.
Semi-supervised algorithms offer another possible solution when labeling too many examples is too expensive; semi-supervised methods also may allow us to avoid learning without enough labeled examples available to learn directly from.
AI and Machine Learning: A Relationship
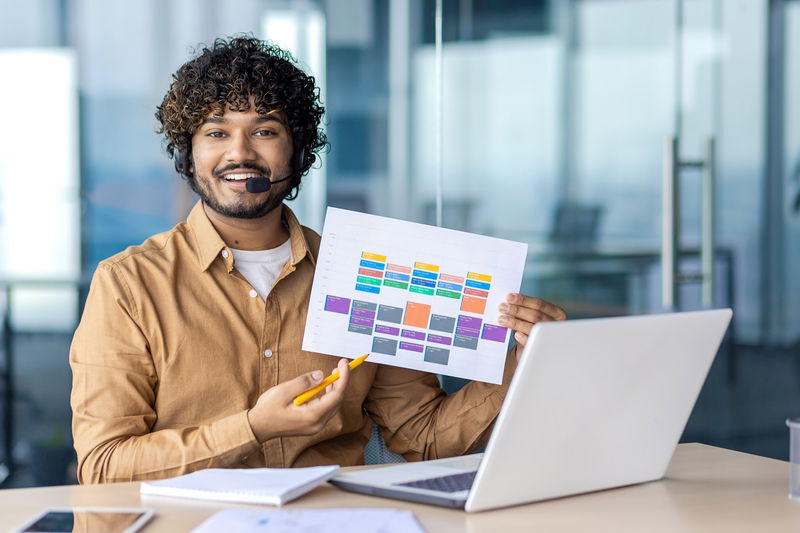
As discussed previously, Artificial Intelligence vs Machine Learning differ significantly, yet both concepts form parts of each other and should therefore be covered together in this chapter.
To do this effectively.
AI refers to the study, development and design of systems capable of cognitively performing the actions, tasks and activities undertaken by humans.
To train such systems on these tasks and activities, datasets with information on them must exist that contain this type of data.
Machine Learning (ML) is an area of AI which involves gathering datasets and training a model with sophisticated statistical algorithms such as Linear Regression to interpret and use that data in ways the AI System was programmed for, performing actions it has been trained for and learning from experience to perform on it's own.
An analogy may help to give a clearer idea of how an AI project actually operates, including explaining its function within machine learning development of Artificial Intelligence.
Machine Learning is at the core of artificial intelligence systems - like building a car's engine! In other words, machine learning provides AI systems with their driving force to move forward in their mission to revolutionise humanity.
Machine Learning algorithms, for example, can analyze vast amounts of data to detect patterns and predict whether a patient has cancer based on similarity to an engine driving a vehicle forward with fuel (i.e.
data) being converted to motion by its engine.
AI cancer classification systems become more accurate and reliable as more data enter the system, while Machine Learning algorithms improve over time - much like how an automobile's engine becomes more powerful as its performance is fine-tuned and optimized with usage.
Read More: How Artificial Intelligence Is Changing The Business World
What Is The Connection Between AI And ML?

They are very closely related, even though AI and ML may not be the same. To understand AI and ML, the easiest way is to:
- AI is a broader concept that enables a machine to act, think, and adapt like a person.
- ML is a form of AI which allows machines to learn and extract knowledge from data.
Imagine these umbrella categories to help you differentiate machine learning and artificial intelligence. AI refers to all manner of algorithms and approaches related to it - this umbrella covers machine learning as well as subfields like deep learning, robots, expert systems and natural-language processing.
AI and Machine Learning: Differences
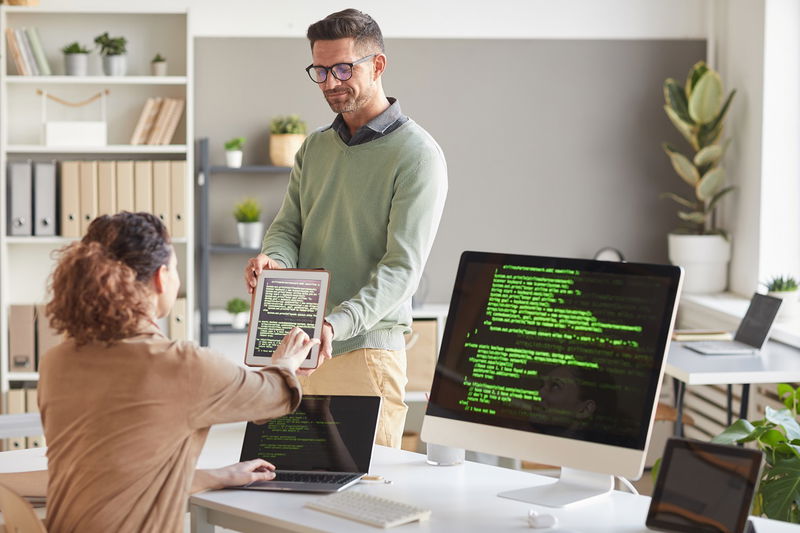
Now that you understand their similarities, what exactly are the distinctions between AI and ML? Machine Learning (ML) differs significantly from artificial intelligence - its purpose being teaching computers how to do certain tasks accurately through pattern identification.
With Google Nest, it is simple and effective. Just ask it "How much time will it take me to reach my office today?," and the device will give an estimate as to when you will reach it.
In doing this exercise you are asking it to perform tasks you would otherwise carry out yourself (ie researching commute times).
At its heart, machine learning (ML) does not aim to perform specific tasks; you could, for instance, train algorithms that analyze real-time transit and traffic data in order to predict its flow - rather the scope of this project lies solely with identifying patterns and measuring accuracy of predictions made using machine learning algorithms.
Artificial Intelligence
- Artificial Intelligence, or AI, refers to machines attempting to mimic human intelligence when solving logical problems.
- Goal of AI project: Building intelligent system capable of performing complex tasks
- We create systems capable of solving complex tasks just like people can.
- Artificial Intelligence (AI) can be utilized for numerous applications and processes. AI provides many applications of its capabilities.
- AI (Artificial Intelligence) is an artificial technology designed to simulate human decision-making processes. AI systems can work with all forms of data including structured, semistructured and unstructured.
- AI systems learn and self-correct using logic and decision trees, improving with experience.
Machine Learning
- ML allows a computer to learn from data in the past.
- It is a goal to create machines that learn from data and increase accuracy.
- We use data to train machines for specific tasks, and we deliver accurate results
- Applications of Machine learning is limited
- Self-learning algorithms are used to create predictive models by ML
- ML only works with structured and semi-structured information
- ML systems rely upon statistical models for learning and can self-correct if provided with new data
AI and ML Can Be Used Together To Achieve Many Benefits
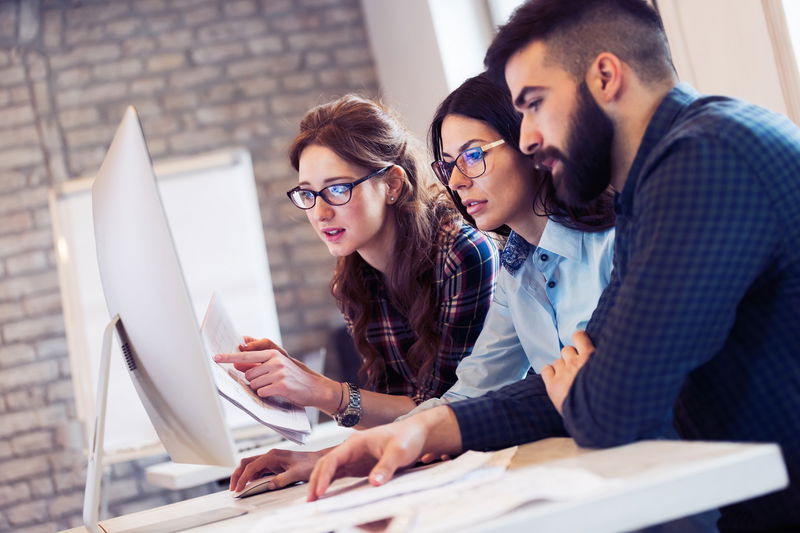
AI and machine learning (ML) technologies offer organizations of any size an incredible competitive edge, and new possibilities continue to open up every day.
As more data floods companies' infrastructures, intelligent automated systems will become even more essential in automating tasks, unlocking values and providing insights that lead to improved results.
Artificial intelligence and machine learning offer numerous business benefits.
Wider data ranges- Analyzing, activating and integrating a wider variety of structured and unstructured data sources.
Faster decision-making- Improved data integrity and faster data processing. Reduced human error.
Efficiency- Increase operational efficiency while reducing costs.
Analytical integration- Integrating predictive analytics into reporting and business applications empowers employees.
AI and ML Applications
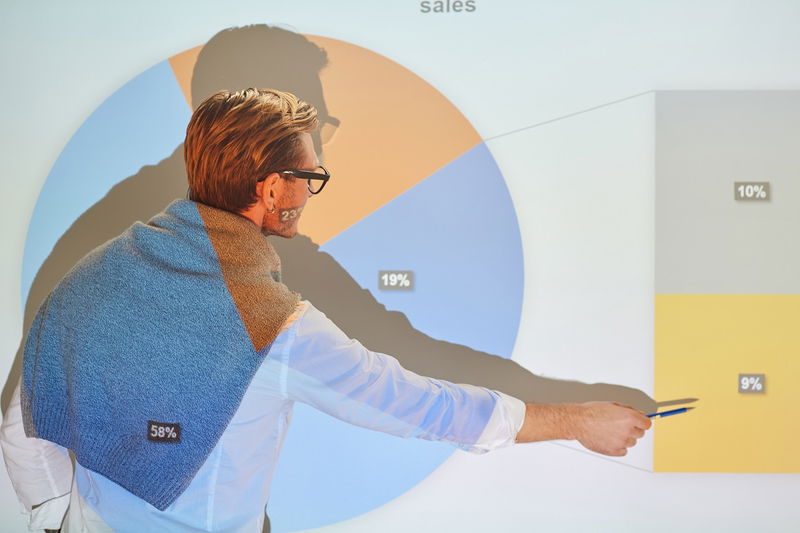
Artificial intelligence and machine-learning technologies offer organizations many potential uses. By automating manual or repetitive processes and helping make informed decisions more quickly.
AI and machine learning (ML) technologies are being leveraged across industries by organizations in an array of ways to revolutionize how they do business and work. Integrating them into strategies and systems enables companies to reimagine how they utilize resources while increasing productivity and efficiency.
Here are a few common AI and ML applications:
Healthcare and Life Sciences
Analysis and insights gleaned from patient health records; forecasting, modeling and outcome prediction, rapid drug development; enhanced diagnostics; monitoring patients; information extraction.
Manufacturers
IoT analytics and production machine monitoring.
Retail and Ecommerce
Inventory optimization and supply chain optimization. Visual search, personalized offers, experiences and recommendation engines.
Financial services
Fraud detection, automated trading and service processing optimization.
Telecommunications
Intelligent networks, network optimization, predictive maintenance and business process automation; upgrade planning and capacity forecasting are some of the services provided.
Want More Information About Our Services? Talk to Our Consultants!
The Conclusion
Artificial Intelligence (AI) and Machine Learning are both widely used in many ways. Both technologies have many real-world applications.
AI and ML do our work without us even realizing it. AI solves tasks that require intelligence from humans, while ML learns from data to provide predictions and is responsible for solving other tasks.
All the ML that we do is part of AI, but not all AI. You can coordinate with any of the machine learning development companies to get the preferred outcome.