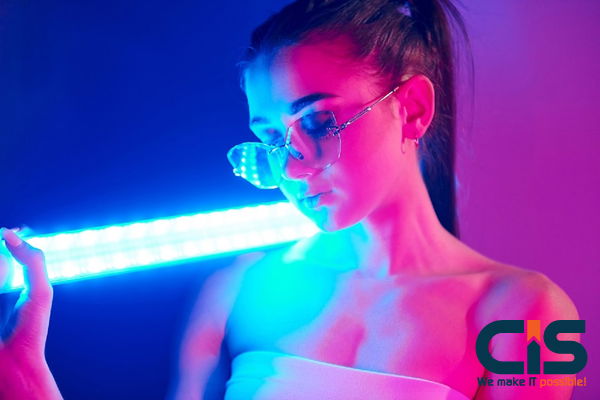
Previously, builders and scientists mixed substances with each other to find out what shaped. This is the way cement, for example, has been discovered. As time passes, they heard the physiological properties of different substances, but a lot of the understanding was nevertheless based on instinct. "If you asked Japanese wrought iron steel has been better at creating knives I don't believe anybody would have told me," states James Warren, manager of the Materials Genome Initiative in the National Institute of Standards and Technology. "They simply had an artisan comprehension of the association between that inner arrangement and awesomeness."
Today, rather than using artisan's consciousness, we could utilize databases and computations to rapidly map out precisely what makes a substance so much lighter or stronger -- which has the capability to revolutionize business after industry, based on Warren. The time between finding a substance and incorporating it into a commodity such as a battery could be over 20 decades, he adds speeding up the procedure is likely to lead us into better glass and batteries for mobile phones and better alloys for rockets, and also much better detectors for health apparatus. "Whatever made from the thing," says Warren, "we could improve."
To comprehend how new substances are created, it is helpful to consider a chemical scientist such as a cook, suggested by Warren. For instance, say you've eggs, and you are in the mood for something chewy and soft. Those would be the qualities of this dish that you need, but just how can you get it? To make a structure by which both white and the yolk are strong, you want a recipe that comprises the step-by-step directions for processing the egg hard boiling it just how you need it. Materials science employs exactly the very same theories: When a scientist needs particular substance properties (state, mild and difficult to break), she'll start looking for the chemical and physical structures which would produce those possessions, and also the procedures -- such as beating or melting metal -- which would produce the required constructions.
Databases and computations will help locate answers. "We perform quantum mechanical-level calculations of substances, calculations complicated enough that we are able to really predict the qualities of a potential new material to a computer before it has ever produced in a lab," states Chris Wolverton, a materials scientist at Northwestern University that operates the Open Quantum Materials Database. (Additional significant databases incorporate the Materials Project along with also the Materials Cloud.) The databases are not complete, however, they are growing and giving us fascinating discoveries.
Nicola Marzari a researcher in Switzerland's École Polytechnique Fédérale p Lausanne, utilized databases to locate 3D substances which may be peeled aside to make 2D substances of only 1 layer. For instance, one case of this may be of the much-hyped graphene, that is made up of one sheet of graphite (the substance at a pencil). Much like graphene, these 2D substances may have exceptional properties, such as strength, they don't possess within their 3D kind.
Marzari's group saw an algorithm shift through data from multiple databases. Beginning from over 100,000 substances, the algorithm finally discovered about 2,000 substances that may be peeled into a single coating, according to the newspaper Marzari printed a month from Nature Nanotechnology. Marzari, that conducts Materials Cloud, states that these substances are a "treasure trove" since most have properties which could improve electronic equipment. Some conduct electricity very well, a few may convert heat into electricity, a few absorb energy in the Sun: They might also be helpful for semiconductors in batteries or computers, and the next thing to do is to research these probable possessions more tightly.
Marzari's job is one such example of how the scientists use databases to forecast which substances might create exciting and new materials. Although, these predictions, still have to get verified at a laboratory. And Marzari still needed to inform his algorithm to follow specific rules, such as searching for poor chemical bonds. Artificial intelligence may make a dictionary: Rather than programming certain principles, scientists could tell AI exactly what they would like to produce -- such as a superstrong substance -- along with the AI will inform the scientists that the ideal experiment to conduct to create the new substance.
That is the way Wolverton and his staff in Northwestern utilized AI to get a newspaper released this month in Science Advances. The investigators were interested in creating new metallic glasses, that are more powerful and less rigid than glass or metal and may one day enhance telephones and spacecraft.
The AI method that they used is like the ways people understand a language that is new, states research co-author Apurva Mehta, a scientist at Stanford University's SLAC National Accelerator Laboratory. A approach to understand a language would be to sit down and memorize all of the rules of punctuation. "But a different method of studying is merely by listening and experience to somebody else speak," says Mehta. Their strategy was a mix. To begin with, the investigators looked through printed papers to discover as much information as you can on just how different kinds of metallic glasses are made. They fed the following "rules of grammar" to a machine-learning algorithm. The algorithm subsequently learned to create its predictions of that combination of components would make a new sort of metallic glass like how a person can boost their French heading to France rather than endlessly memorizing conjugation graphs. Mehta's team then analyzed the system's ideas in laboratory experiments.
Scientists may synthesize and examine thousands of substances at one moment. But at this rate, it could be a waste of time to test every possible mix. "They can not simply throw the entire periodic table in their gear," states Wolverton, or so the function of this AI is to "indicate some areas for them to begin." The procedure was not ideal, and a few suggestions -- such as the specific proportion of components required were away, but the scientists could produce fresh metallic glasses. Additionally, performing the experiments implies they had more information to feed into the algorithm so that it can develop itself into a more smarter and brighter machine every moment.
Another method of utilizing AI is to produce a "cookbook," or some group of recipes to get substances. In two newspapers published late last year, MIT scientists created a machine-learning system which scans academic newspapers to determine which ones comprise directions for making sure substances. It might notice using 99 % accuracy which paragraphs of the paper comprised the "recipe," and also 86 % precision the specific words from the paragraph.
The MIT group is currently preparing the AI to become more precise. They'd love to make a record of those recipes to your science community at large, however they will need to work together with the writer of those educational papers to be certain their collection does not violate any arrangements. At some point, the team wishes to educate the machine to read newspapers and produce new recipes by itself.
"One aim would be to find more effective and cost-effective methods for earning substances that we produce," says research co-author and MIT materials scientist Elsa Olivetti. "Still another is, here is the chemical the computational science called, can we indicate a much better pair of methods to make it"
The near future of AI and materials mathematics appears to be promising, however, challenges remain. To begin with, computers just are not able to predict everything. "The forecasts themselves have mistakes and frequently operate on a simplified version of substances that do not take into consideration the actual world," states Marzari out of EPFL. There are all types of environmental variables, such as humidity and temperature, which influence the way the substances act. And many versions can not take these into consideration.
Another issue is that we do not have sufficient information about each compound, said Wolverton, and also a deficiency of data implies algorithms are not very wise. Nevertheless, Wolverton and Mehta are considering about using their strategy on other categories of substances besides glass. And they expect one evening, you will not require a person to do experiments in any way, it is going to only be AI and robots. "We could make quite an entirely autonomous program," Wolverton says, "with no individual being included."